Robust Liver Vessel Extraction Using DV-Net with D-BCE Loss Function
Advances in Artificial Intelligence and SecurityCommunications in Computer and Information Science(2021)
摘要
Recently, liver vessel segmentation has aroused widespread interests in medical image analysis. Accurately extracting blood vessels from the liver is a difficult task due to the complex vessel structures and image noise. To make the network better adapt to this complex feature, a deeper network is needed to fit this nonlinear transformation. In this work, we introduce the dense block structure into the V-net to construct a new Dense V-Net (DV-Net) and use data augmentation to segment the liver vessels from abdominal CT volumes with few training samples. Besides, we propose the D-BCE loss function to cope with the problem of dynamic changes in pixel ratio caused by 3D segmentation random patches, which can also control the trade-off between false positives and negatives. In this way, the proposed DV-Net structure can acquire a more powerful discrimination capability between vessel areas and non-vessel areas. Our method is tested on the public datasets from 3Dircadb. The average dice and sensitivity on the 3Dircadb dataset were 74.76% and 75.27% respectively. Experiments are also conducted in another public dataset from Medical Segmentation Decalthon and also obtain much higher accuracies. Moreover, our approach is automatic, accurate and robust for liver vessel extraction and can enjoy better convergence properties, making it more efficient and reliable in practice.
更多查看译文
关键词
extraction,liver,dv-net,d-bce
AI 理解论文
溯源树
样例
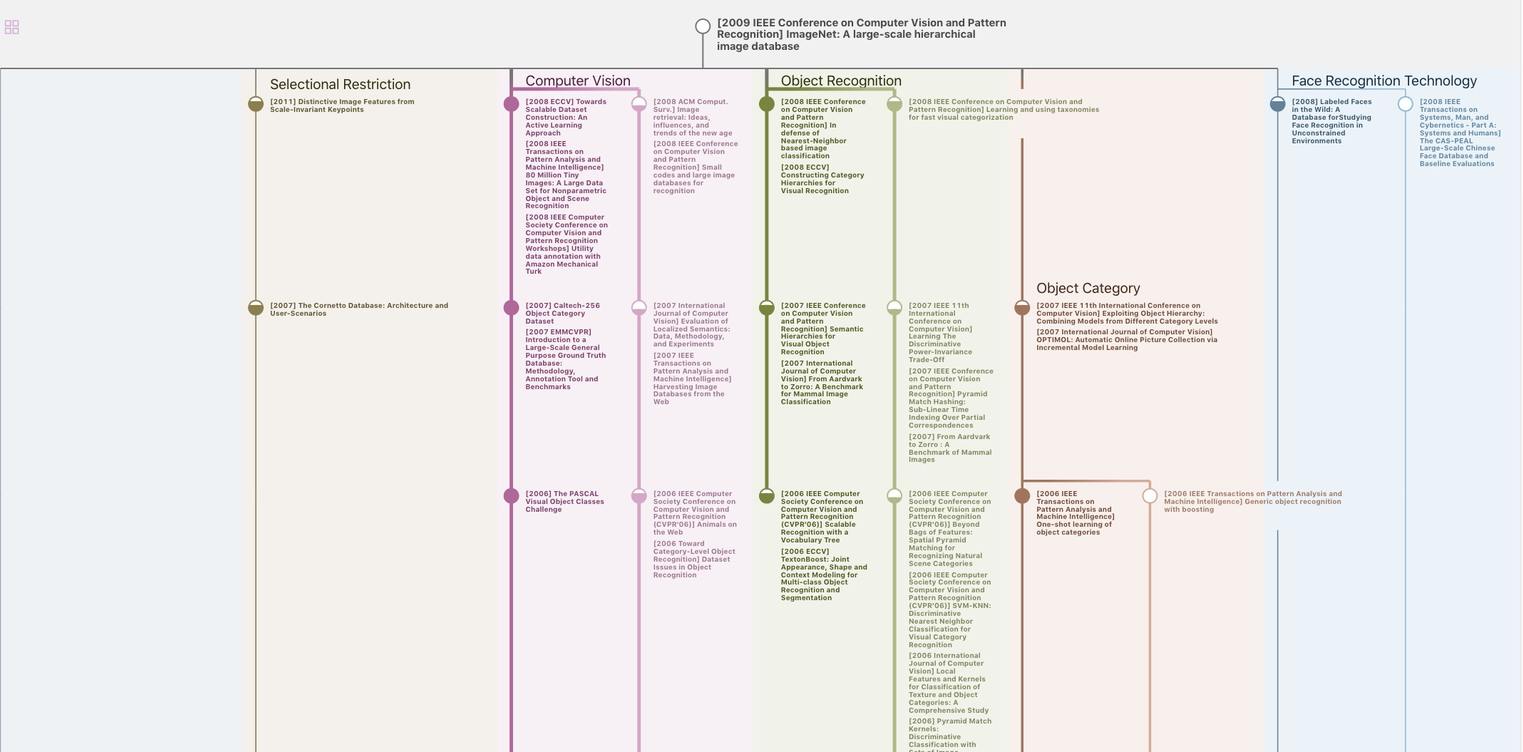
生成溯源树,研究论文发展脉络
Chat Paper
正在生成论文摘要