Visual Analytics e Outlying Aspect Mining: contextualização de anomalias considerando questões temporais e multidimensionais
Anais da XVI Escola Regional de Banco de Dados (ERBD 2021)(2021)
摘要
Outlying Aspect Mining (OAM) is a new way of handling outliers that, instead of focusing solely on the detection, also provides an explanation. This is done by presenting a subspace of attributes that had the most abnormal behavior. Acknowledging this group of attributes is important but only listing them is not sufficient for a human specialist to comprehend the situation and take the necessary actions. A higher-level, visual approach can improve the process, providing better cognitive clues to experts. Here we describe a Visual Analytics platform developed to present data and OAM outputs in a human-friendly interface. A novelty available on this platform is a parallel coordinates plot that also display temporal multidimensional data. Such representation overcome human visual system limitations and helps in the outlier investigation. To explore the applicability of the developed tool, a locomotive operation user case is employed with focus on fault analysis in an OAM point of view.
更多查看译文
AI 理解论文
溯源树
样例
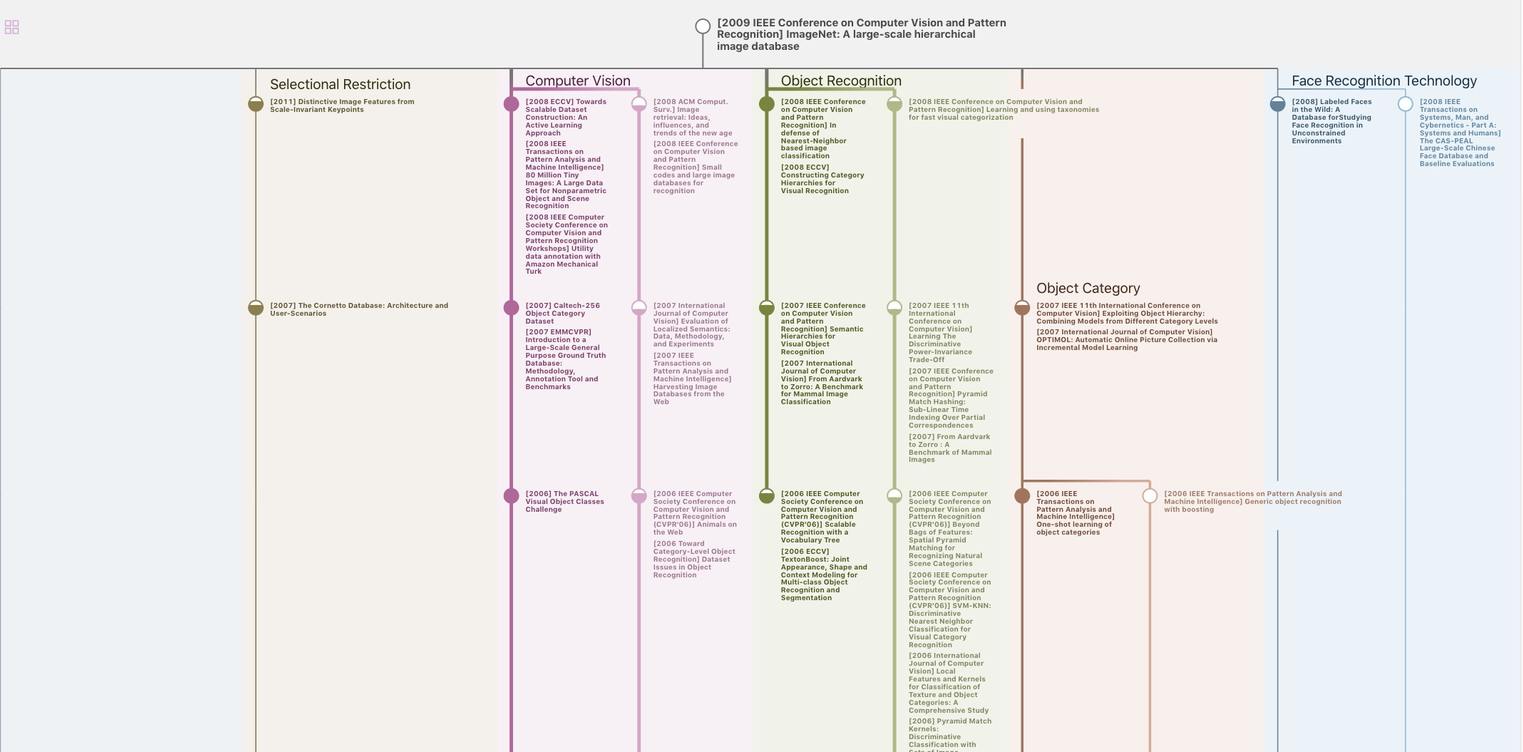
生成溯源树,研究论文发展脉络
Chat Paper
正在生成论文摘要