Robust Portfolio Optimization Models When Stock Returns Are a Mixture of Normals
Springer Proceedings in Business and EconomicsAI and Analytics for Public Health(2022)
摘要
Using optimization techniques in portfolio selection has attracted significant attention in financial decisions. However, one of the main challenging aspects faced in optimal portfolio selection is that the models are sensitive to the estimations of the uncertain parameters. In this paper, we focus on the robust optimization problems to incorporate uncertain parameters into the standard portfolio problems. We provide reformulations of the robust versions of portfolio optimization problems under different uncertainty sets as conic programs with two risk measures: variance and Conditional Value-at-Risk under the assumption that the stock returns are a mixture of normals. Finally, we conduct a computational study on a real data set to evaluate and compare the effectiveness of the robust optimization approaches. Our results suggest that employing robust models with budgeted uncertainty may provide higher returns for the same levels of risk, especially for investors inclined to take more risk, and the uncertainty in the covariance estimations does not have a significant impact.
更多查看译文
关键词
robust portfolio optimization models,stock returns
AI 理解论文
溯源树
样例
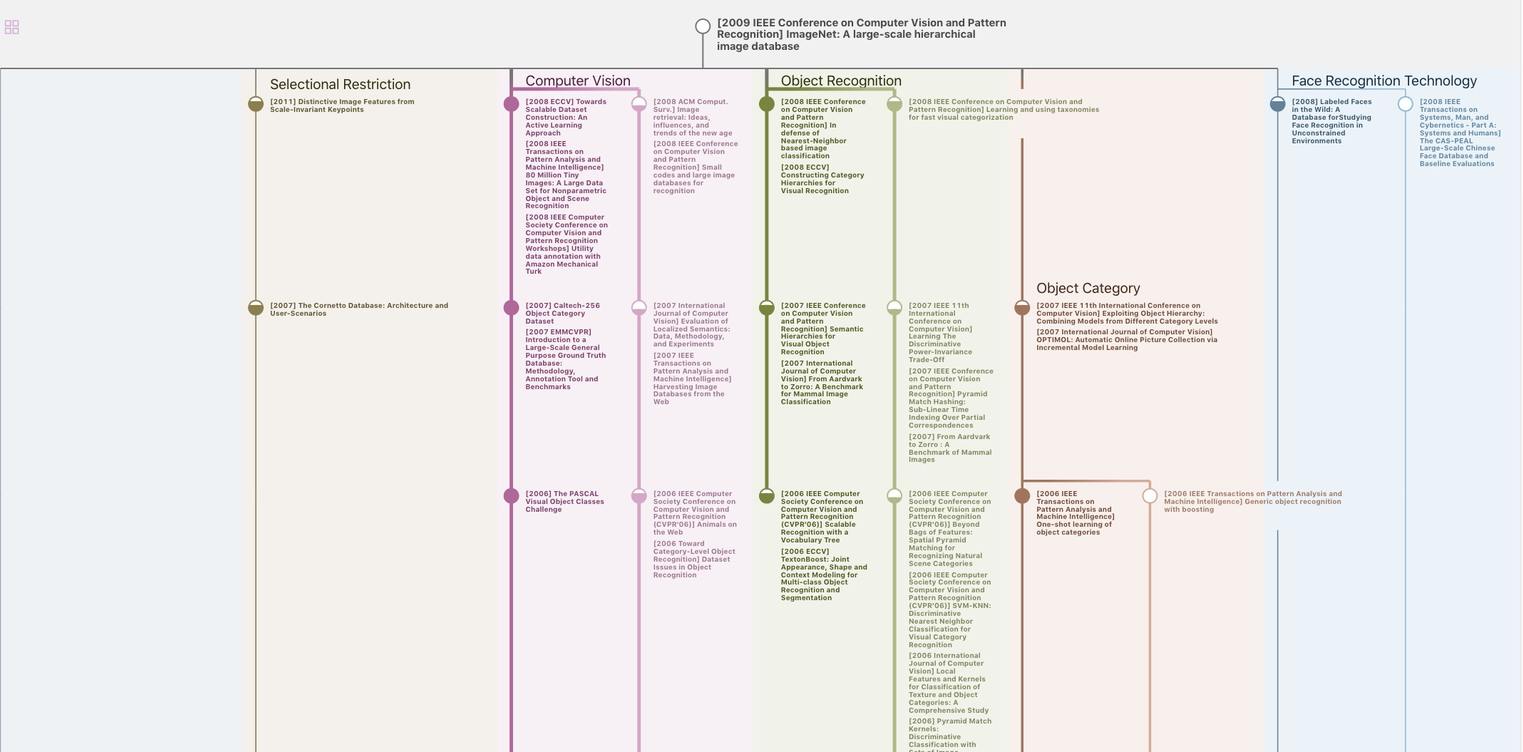
生成溯源树,研究论文发展脉络
Chat Paper
正在生成论文摘要