A Novel Fusion Method for Generating Surface Soil Moisture Data with High Accuracy, High Spatial Resolution, and High Spatio‐Temporal Continuity
Water Resources Research(2022)
摘要
Surface soil moisture (SSM) has a considerable impact on land-atmosphere exchanges of water and energy fluxes. However, due to the inherent deficiencies of remotely sensed data (e.g., cloud contamination in thermal remote sensing and coarse resolutions for microwave remote sensing), none of the current algorithms alone can provide daily and seamless field-scale (30 m) SSM information. To fill the gap, we proposed a novel SSM fusion framework to Generate high Resolution, Accurate, Seamless data using Point-Surface fusion (GRASPS) based on remotely sensed, reanalysis, and in-situ data sets. First, 30 m seamless continuous SSM correlated variables (land surface temperature, NDVI, and albedo) were downscaled by enhanced spatial and temporal adaptive reflectance fusion model. Then, downscaled auxiliary variables and other background variables were input into a deep learning model to produce 30 m daily and seamless SSM fields. To further improve the SSM estimation accuracy, a pixel classification-based bias correction method was developed. The GRASPS method was validated over an in situ soil moisture sensor network (HOAL network) in Austria. The average Pearson correlation coefficient, root mean square error (RMSE), unbiased RMSE (ubRMSE), bias, and mean absolute error (MAE) over all validation sites achieved 0.78, 0.048, 0.033, -0.001, and 0.041 m3m-3 ${\mathrm{m}}<^>{3}{\mathrm{m}}<^>{-3}$, respectively. After bias correction, RMSE, ubRMSE, bias, and MAE decreased by 13%, 7%, 22%, and 18%, respectively. The proposed method maximizes the potential of data fusion and deep learning in generating field-scale seamless SSM, which is promising for fine-scale studies and applications in agricultural, hydrological, and environmental domains.
更多查看译文
关键词
surface soil moisture, point-surface data fusion, deep learning
AI 理解论文
溯源树
样例
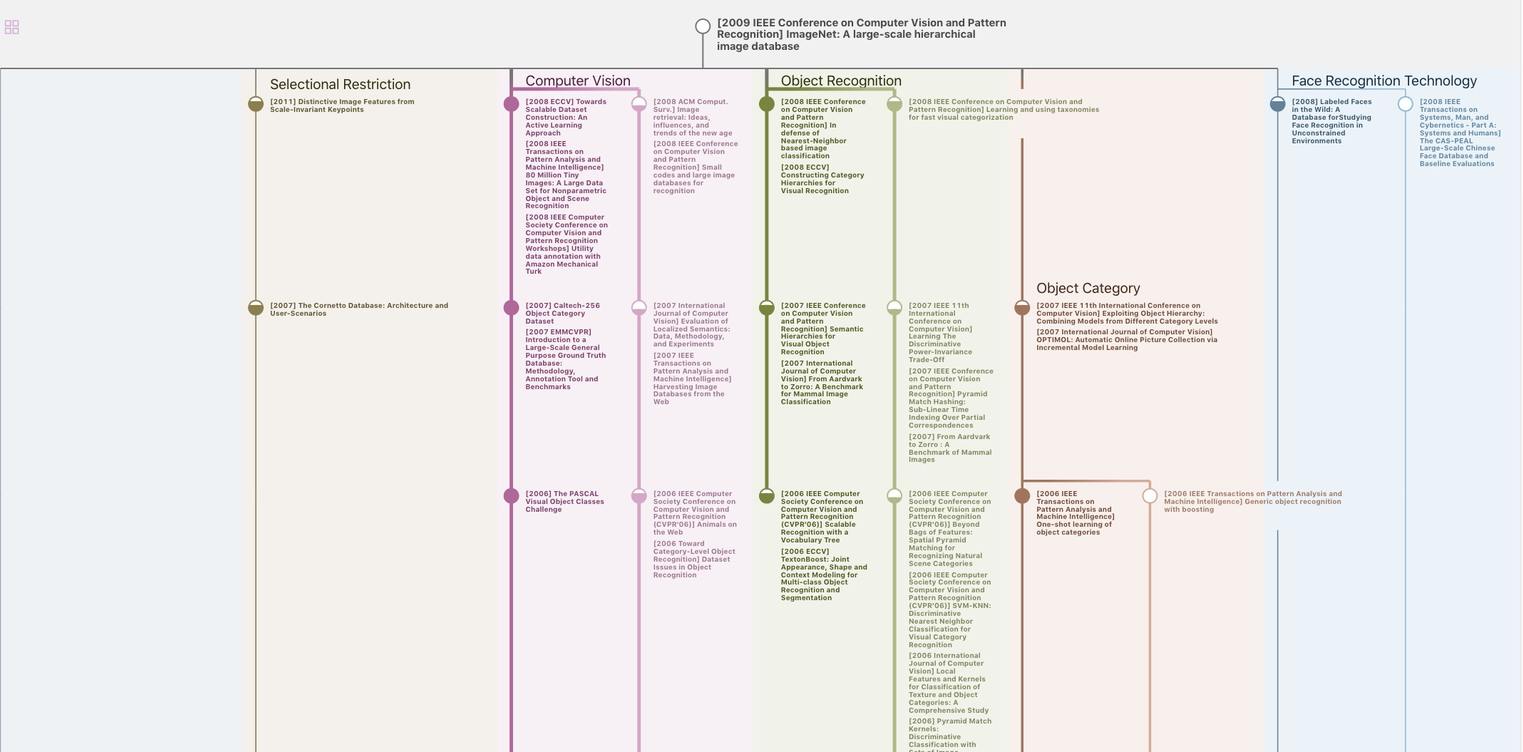
生成溯源树,研究论文发展脉络
Chat Paper
正在生成论文摘要