Calculating probability densities with homotopy, and applications to particle filters
International Journal for Uncertainty Quantification(2022)
摘要
We explore a homotopy sampling procedure and its generalization, loosely based on importance sampling, known as annealed importance sampling. The procedure makes use of a known probability distribution to find, via homotopy, the unknown normalization of a target distribution, as well as samples of the target distribution. We propose a reformulation of the method that leads to a rejection sampling alternative. Estimates of the error as a function of homotopy stages and sample averages are derived for the algorithmic version of the method. These estimates are useful in making computational efficiency decisions on how the calculation should proceed, given a computer architecture. Consideration is given to how the procedure can be adapted to Bayesian stationary and nonstationary estimation problems. The connection between homotopy sampling and thermodynamic integration is made. Emphasis is placed on the non-stationary problems, and in particular, on a sequential estimation technique known as particle filtering. It is shown that a modification of the particle filter framework to include the homotopy process can improve the computational robustness of particle filters.
更多查看译文
关键词
sampling,homotopy,sequential Monte Carlo,Bayesian estimation,data assimilation
AI 理解论文
溯源树
样例
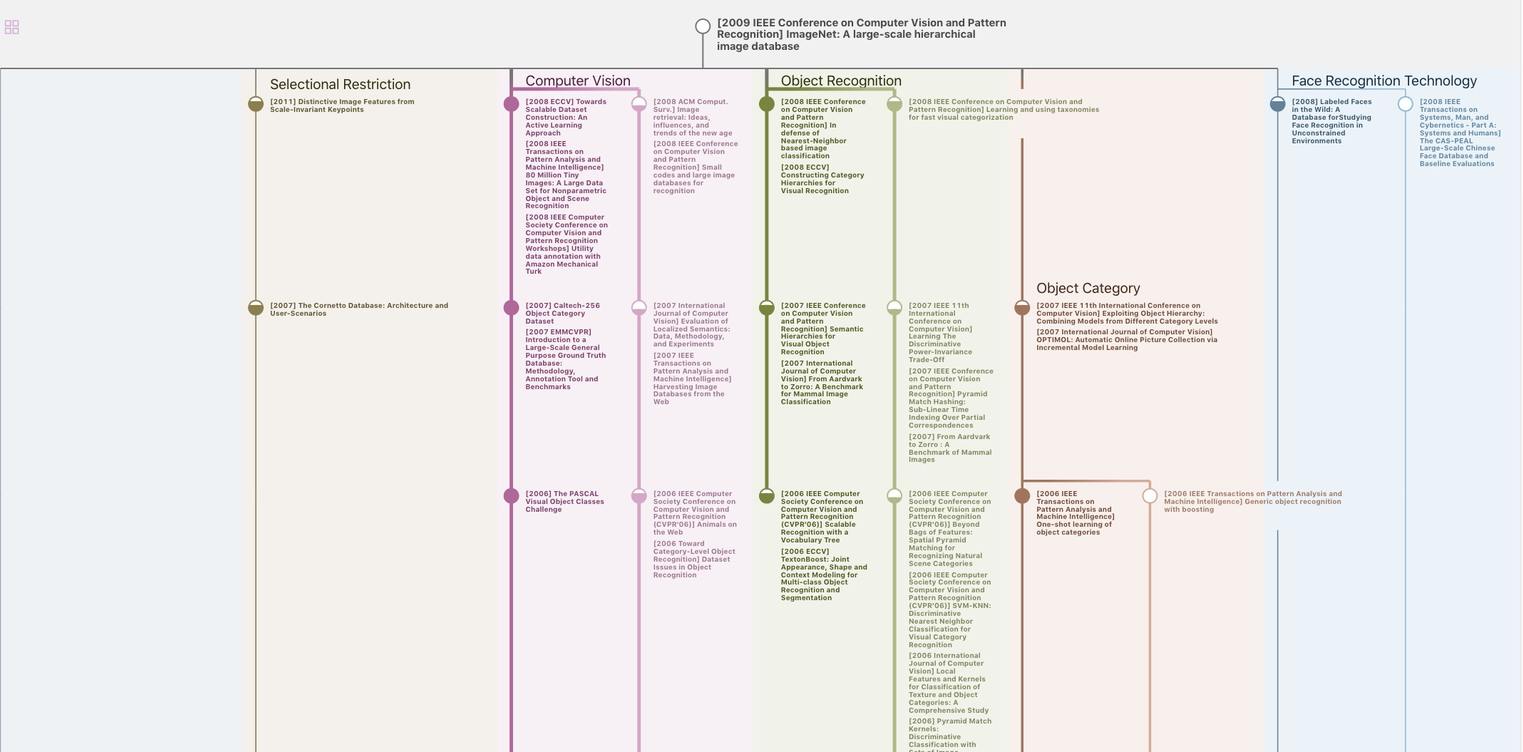
生成溯源树,研究论文发展脉络
Chat Paper
正在生成论文摘要