Challenges and Opportunities in Deep Reinforcement Learning With Graph Neural Networks: A Comprehensive Review of Algorithms and Applications
IEEE TRANSACTIONS ON NEURAL NETWORKS AND LEARNING SYSTEMS(2023)
摘要
Deep reinforcement learning (DRL) has empowered a variety of artificial intelligence fields, including pattern recognition, robotics, recommendation systems, and gaming. Similarly, graph neural networks (GNNs) have also demonstrated their superior performance in supervised learning for graphstructured data. In recent times, the fusion of GNN with DRL for graph-structured environments has attracted a lot of attention. This article provides a comprehensive review of these hybrid works. These works can be classified into two categories: 1) algorithmic contributions, where DRL and GNN complement each other with an objective of addressing each other's shortcomings and 2) application-specific contributions that leverage a combined GNN-DRL formulation to address problems specific to different applications. This fusion effectively addresses various complex problems in engineering and life sciences. Based on the review, we further analyze the applicability and benefits of fusing these two domains, especially in terms of increasing generalizability and reducing computational complexity. Finally, the key challenges in integrating DRL and GNN, and potential future research directions are highlighted, which will be of interest to the broader machine learning community.
更多查看译文
关键词
Deep learning,deep reinforcement learning (DRL),graph neural network (GNN),hybrid DRL-GNN,survey
AI 理解论文
溯源树
样例
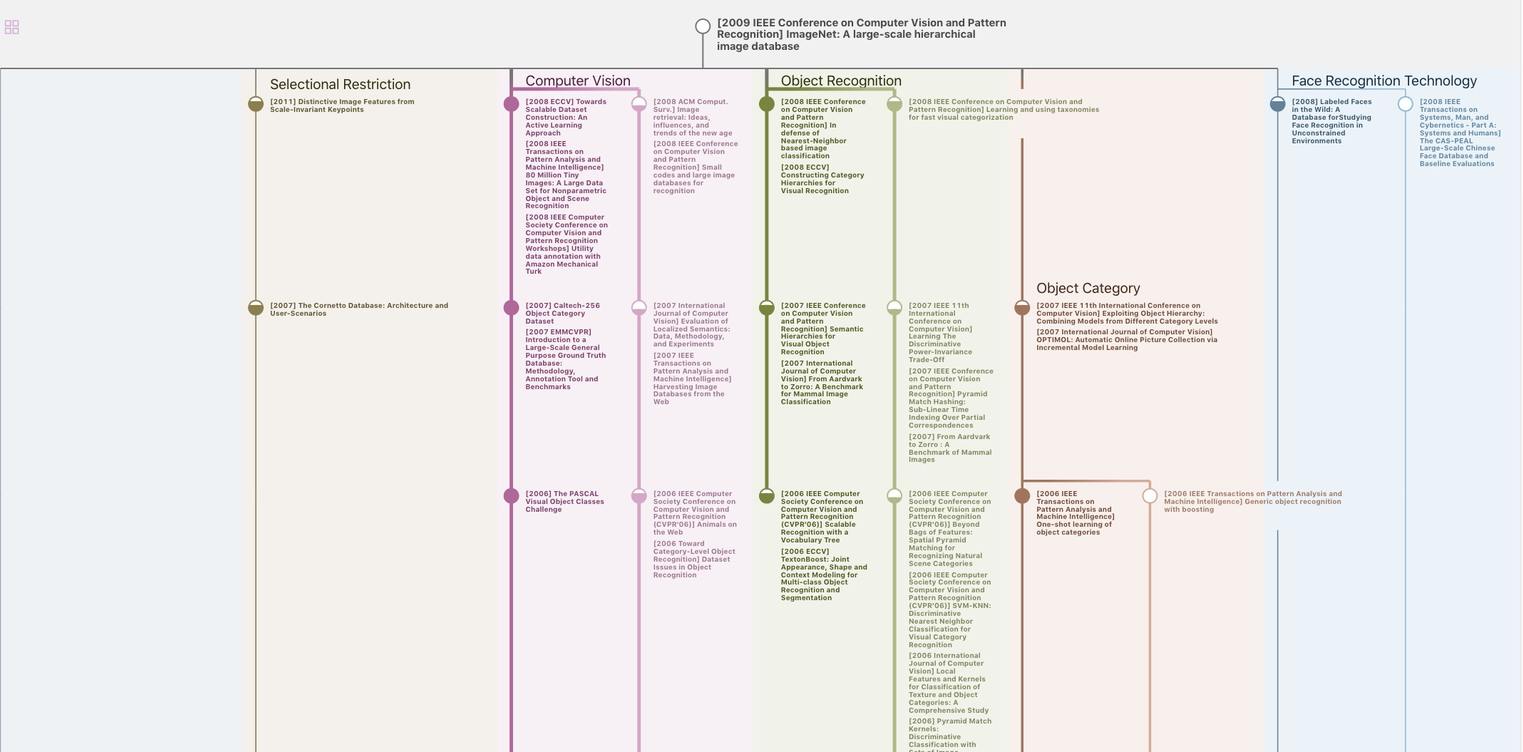
生成溯源树,研究论文发展脉络
Chat Paper
正在生成论文摘要