Domain Generalization via Selective Consistency Regularization for Time Series Classification.
ICPR(2022)
摘要
Domain generalization methods aim to learn models robust to domain shift with data from a limited number of source domains and without access to target domain samples during training. Popular domain alignment methods for domain generalization seek to extract domain-invariant features by minimizing the discrepancy between feature distributions across all domains, disregarding inter-domain relationships. In this paper, we instead propose a novel representation learning methodology that selectively enforces prediction consistency between source domains estimated to be closely-related. Specifically, we hypothesize that domains share different class-informative representations, so instead of aligning all domains which can cause negative transfer, we only regularize the discrepancy between closely-related domains. We apply our method to time-series classification tasks and conduct comprehensive experiments on three public real-world datasets. Our method significantly improves over the baseline and achieves better or competitive performance in comparison with state-of-the-art methods in terms of both accuracy and model calibration.
更多查看译文
关键词
domain generalization,selective consistency regularization,classification,time
series
AI 理解论文
溯源树
样例
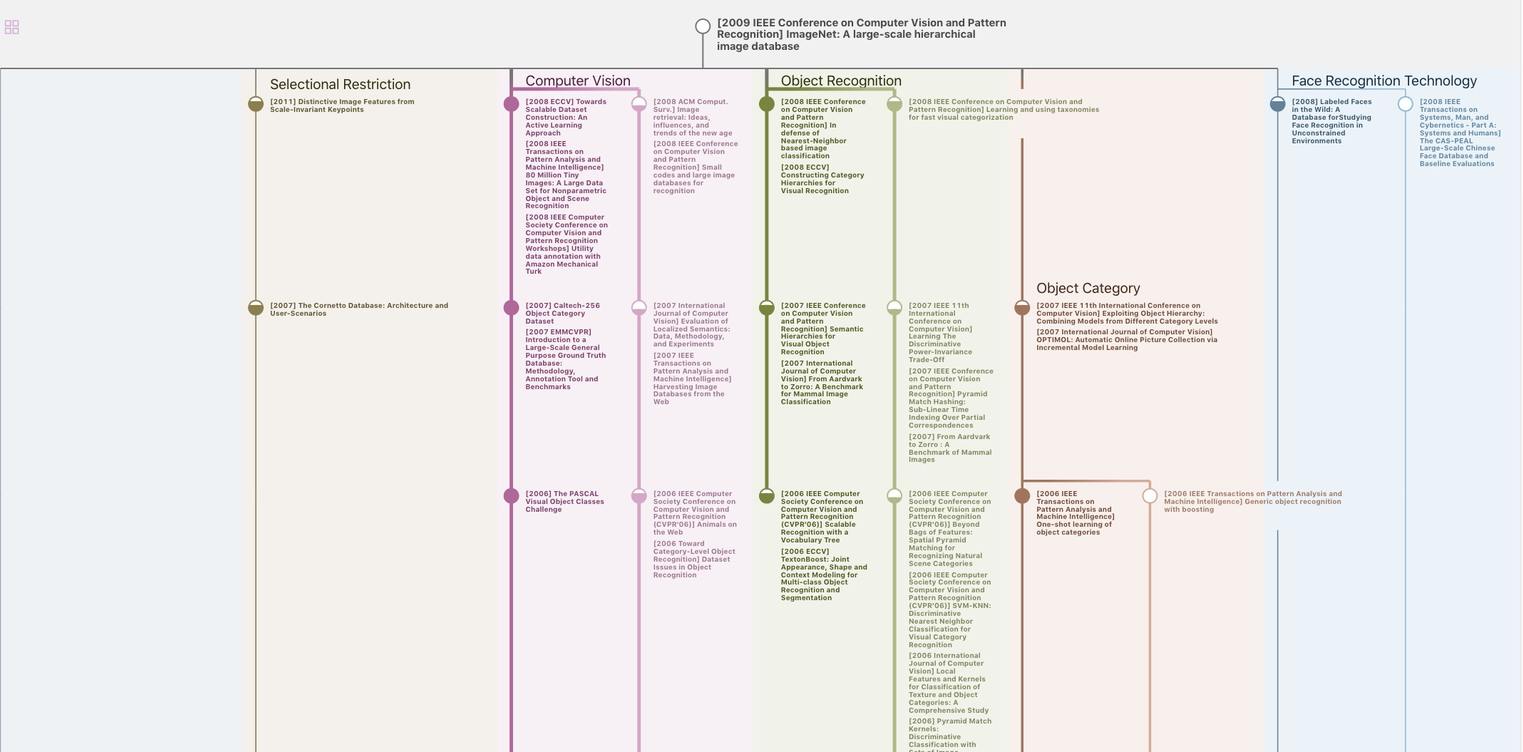
生成溯源树,研究论文发展脉络
Chat Paper
正在生成论文摘要