Variable Bitrate Neural Fields
International Conference on Computer Graphics and Interactive Techniques(2022)
摘要
BSTRACT Neural approximations of scalar- and vector fields, such as signed distance functions and radiance fields, have emerged as accurate, high-quality representations. State-of-the-art results are obtained by conditioning a neural approximation with a lookup from trainable feature grids [Liu et al. 2020; Martel et al. 2021; Müller et al. 2022; Takikawa et al. 2021] that take on part of the learning task and allow for smaller, more efficient neural networks. Unfortunately, these feature grids usually come at the cost of significantly increased memory consumption compared to stand-alone neural network models. We present a dictionary method for compressing such feature grids, reducing their memory consumption by up to 100 × and permitting a multiresolution representation which can be useful for out-of-core streaming. We formulate the dictionary optimization as a vector-quantized auto-decoder problem which lets us learn end-to-end discrete neural representations in a space where no direct supervision is available and with dynamic topology and structure. Our source code is available at https://github.com/nv-tlabs/vqad.
更多查看译文
关键词
variable bitrate neural fields
AI 理解论文
溯源树
样例
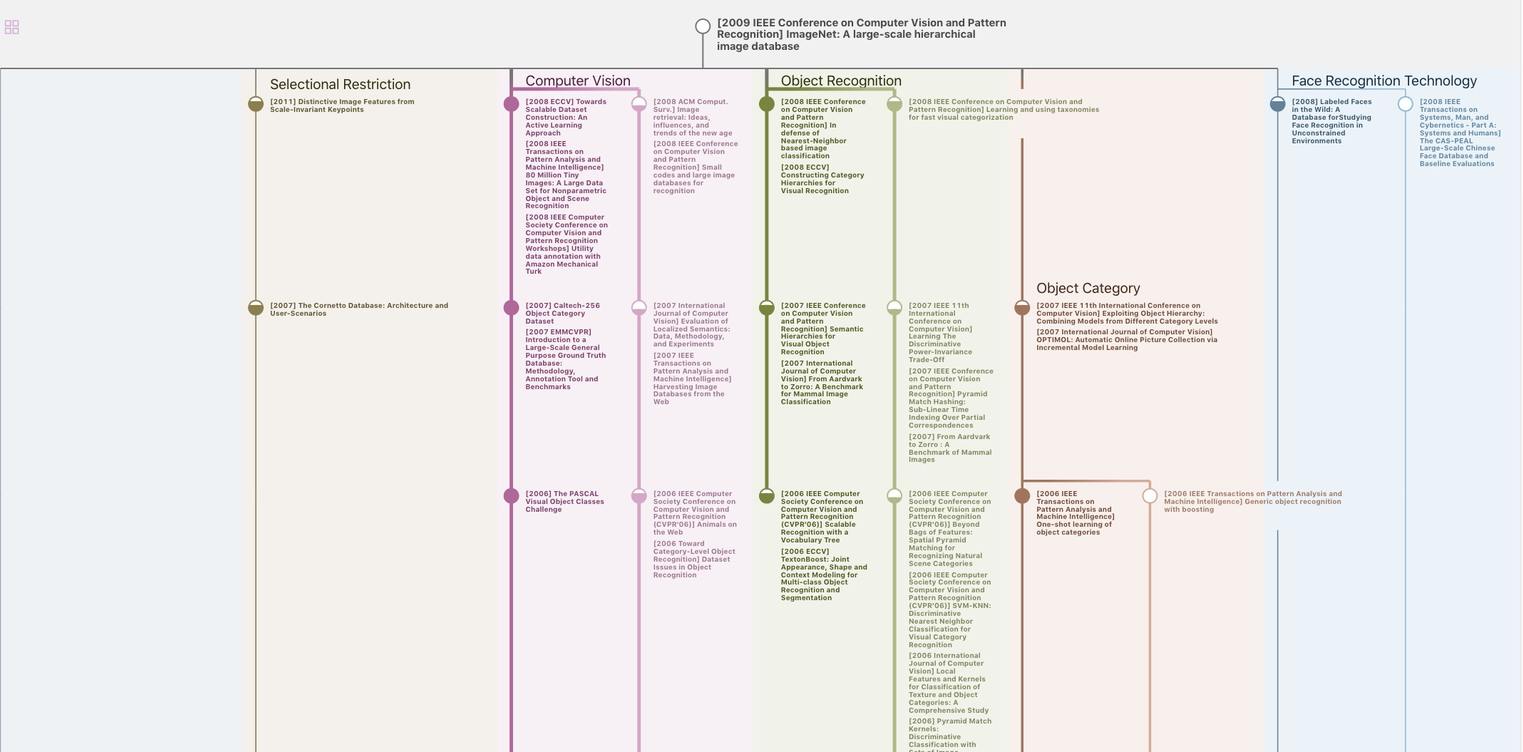
生成溯源树,研究论文发展脉络
Chat Paper
正在生成论文摘要