LAVENDER: Unifying Video-Language Understanding As Masked Language Modeling
CVPR 2023(2023)
摘要
Unified vision-language frameworks have greatly advanced in recent years, most of which adopt an encoder-decoder architecture to unify image-text tasks as sequence-to-sequence generation. However, existing video-language (VidL) models still require task-specific designs in model architecture and training objectives for each task. In this work, we explore a unified VidL framework LAVENDER, where Masked Language Modeling (MLM) is used as the common interface for all pre-training and downstream tasks. Such unification leads to a simplified model architecture, where only a lightweight MLM head, instead of a decoder with much more parameters, is needed on top of the multimodal encoder. Surprisingly, experimental results show that this unified framework achieves competitive performance on 14 VidL benchmarks, covering video question answering, text-to-video retrieval and video captioning. Extensive analyses further demonstrate LAVENDER can (i) seamlessly support all downstream tasks with just a single set of parameter values when multi-task finetuned; (ii) generalize to various downstream tasks with limited training samples; and (iii) enable zero-shot evaluation on video question answering tasks.
更多查看译文
关键词
masked video-language,understanding
AI 理解论文
溯源树
样例
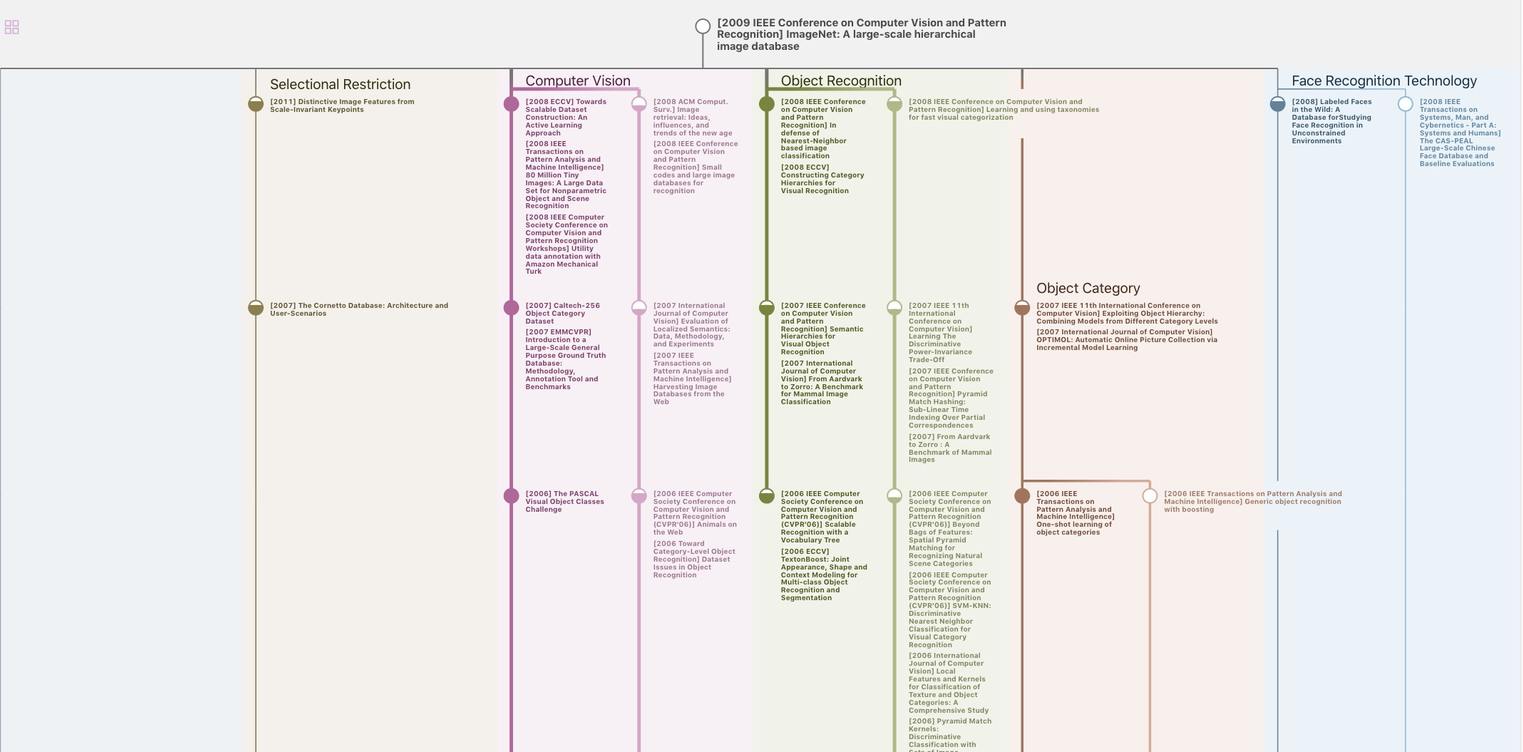
生成溯源树,研究论文发展脉络
Chat Paper
正在生成论文摘要