Fast Text Generation with Text-Editing Models
PROCEEDINGS OF THE 29TH ACM SIGKDD CONFERENCE ON KNOWLEDGE DISCOVERY AND DATA MINING, KDD 2023(2023)
摘要
Text-editing models have recently become a prominent alternative to seq2seq models for monolingual text-generation tasks such as grammatical error correction, simplification, and style transfer. These tasks share a common trait - they exhibit a large amount of textual overlap between the source and target texts. Text-editing models take advantage of this observation and learn to generate the output by predicting edit operations applied to the source sequence. In contrast, seq2seq models generate outputs word-by-word from scratch thus making them slow at inference time. Text-editing models provide several benefits over seq2seq models including faster inference speed, higher sample efficiency, and better control and interpretability of the outputs. This tutorial provides a comprehensive overview of text-editing models and current state-of-the-art approaches, and analyzes their pros and cons. We discuss challenges related to productionization and how these models can be used to mitigate hallucination and bias, both pressing challenges in the field of text generation.
更多查看译文
关键词
text editing,text generation,language models
AI 理解论文
溯源树
样例
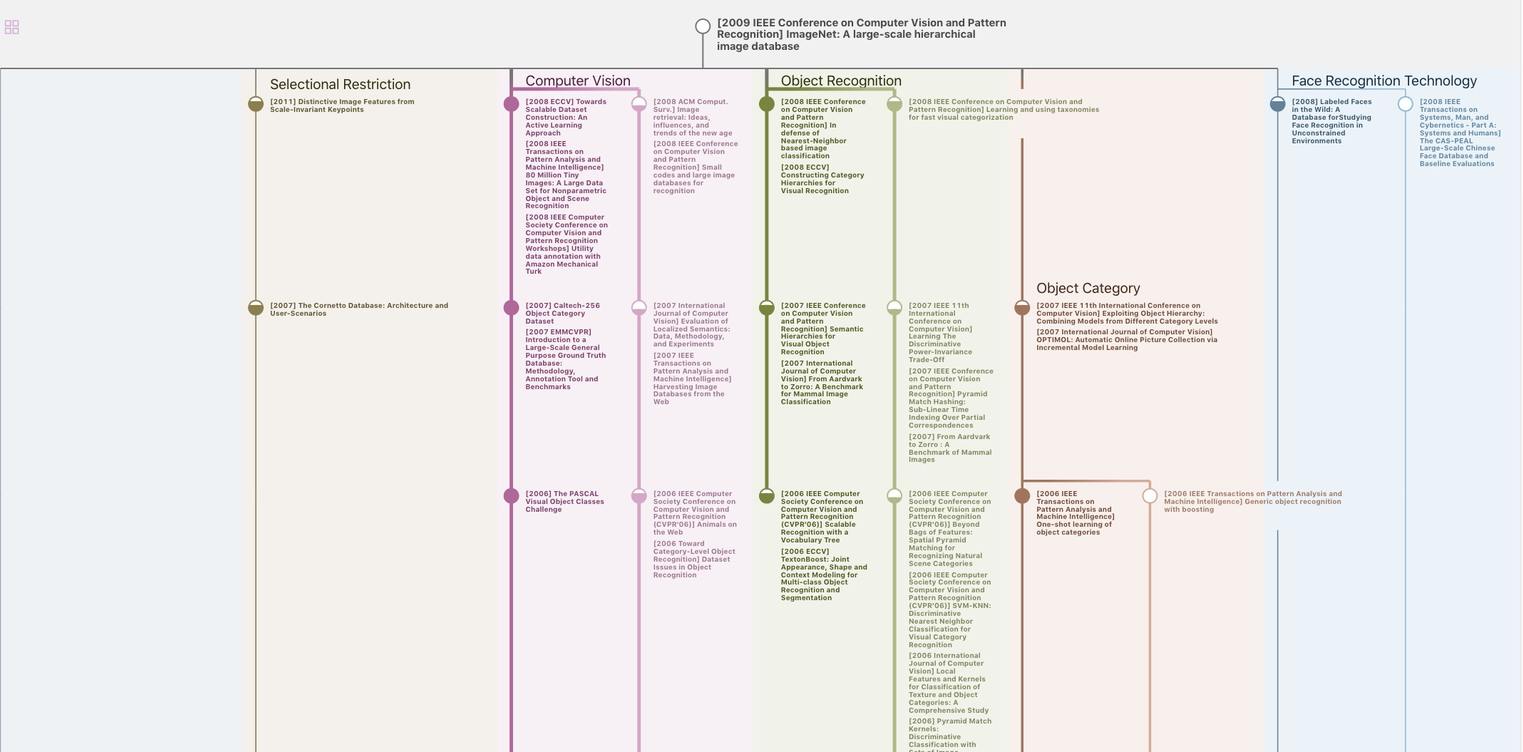
生成溯源树,研究论文发展脉络
Chat Paper
正在生成论文摘要