Evaluating histopathology transfer learning with ChampKit
arxiv(2022)
摘要
Histopathology remains the gold standard for diagnosis of various cancers. Recent advances in computer vision, specifically deep learning, have facilitated the analysis of histopathology images for various tasks, including immune cell detection and microsatellite instability classification. The state-of-the-art for each task often employs base architectures that have been pretrained for image classification on ImageNet. The standard approach to develop classifiers in histopathology tends to focus narrowly on optimizing models for a single task, not considering the aspects of modeling innovations that improve generalization across tasks. Here we present ChampKit (Comprehensive Histopathology Assessment of Model Predictions toolKit): an extensible, fully reproducible benchmarking toolkit that consists of a broad collection of patch-level image classification tasks across different cancers. ChampKit enables a way to systematically document the performance impact of proposed improvements in models and methodology. ChampKit source code and data are freely accessible at https://github.com/kaczmarj/champkit .
更多查看译文
关键词
histopathology transfer,champkit,learning
AI 理解论文
溯源树
样例
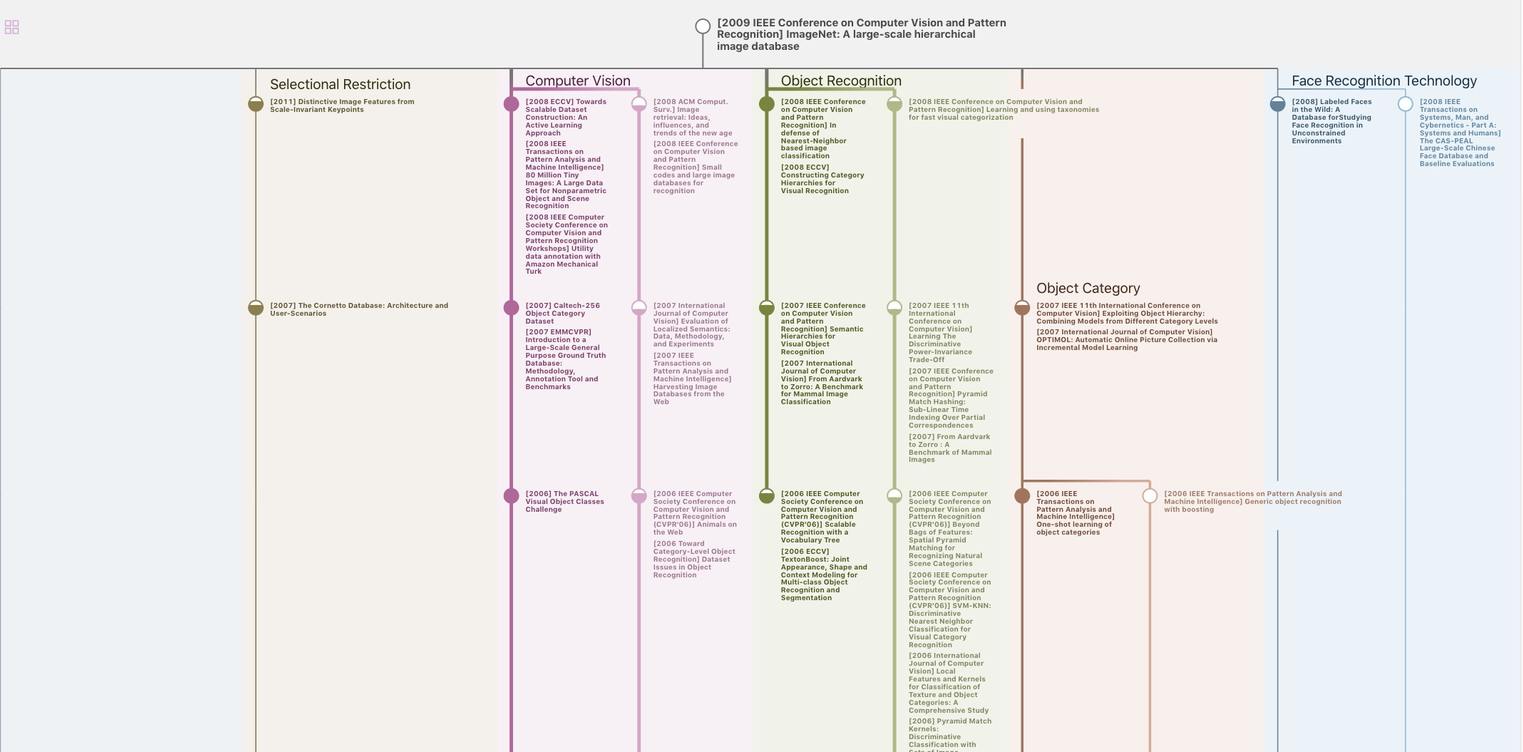
生成溯源树,研究论文发展脉络
Chat Paper
正在生成论文摘要