Multi-type feature fusion based on graph neural network for drug-drug interaction prediction
BMC Bioinformatics(2022)
摘要
Background Drug-Drug interactions (DDIs) are a challenging problem in drug research. Drug combination therapy is an effective solution to treat diseases, but it can also cause serious side effects. Therefore, DDIs prediction is critical in pharmacology. Recently, researchers have been using deep learning techniques to predict DDIs. However, these methods only consider single information of the drug and have shortcomings in robustness and scalability. Results In this paper, we propose a multi-type feature fusion based on graph neural network model (MFFGNN) for DDI prediction, which can effectively fuse the topological information in molecular graphs, the interaction information between drugs and the local chemical context in SMILES sequences. In MFFGNN, to fully learn the topological information of drugs, we propose a novel feature extraction module to capture the global features for the molecular graph and the local features for each atom of the molecular graph. In addition, in the multi-type feature fusion module, we use the gating mechanism in each graph convolution layer to solve the over-smoothing problem during information delivery. We perform extensive experiments on multiple real datasets. The results show that MFFGNN outperforms some state-of-the-art models for DDI prediction. Moreover, the cross-dataset experiment results further show that MFFGNN has good generalization performance. Conclusions Our proposed model can efficiently integrate the information from SMILES sequences, molecular graphs and drug-drug interaction networks. We find that a multi-type feature fusion model can accurately predict DDIs. It may contribute to discovering novel DDIs.
更多查看译文
关键词
Multi-type feature fusion,Graph neural network,Gating mechanism,Link prediction
AI 理解论文
溯源树
样例
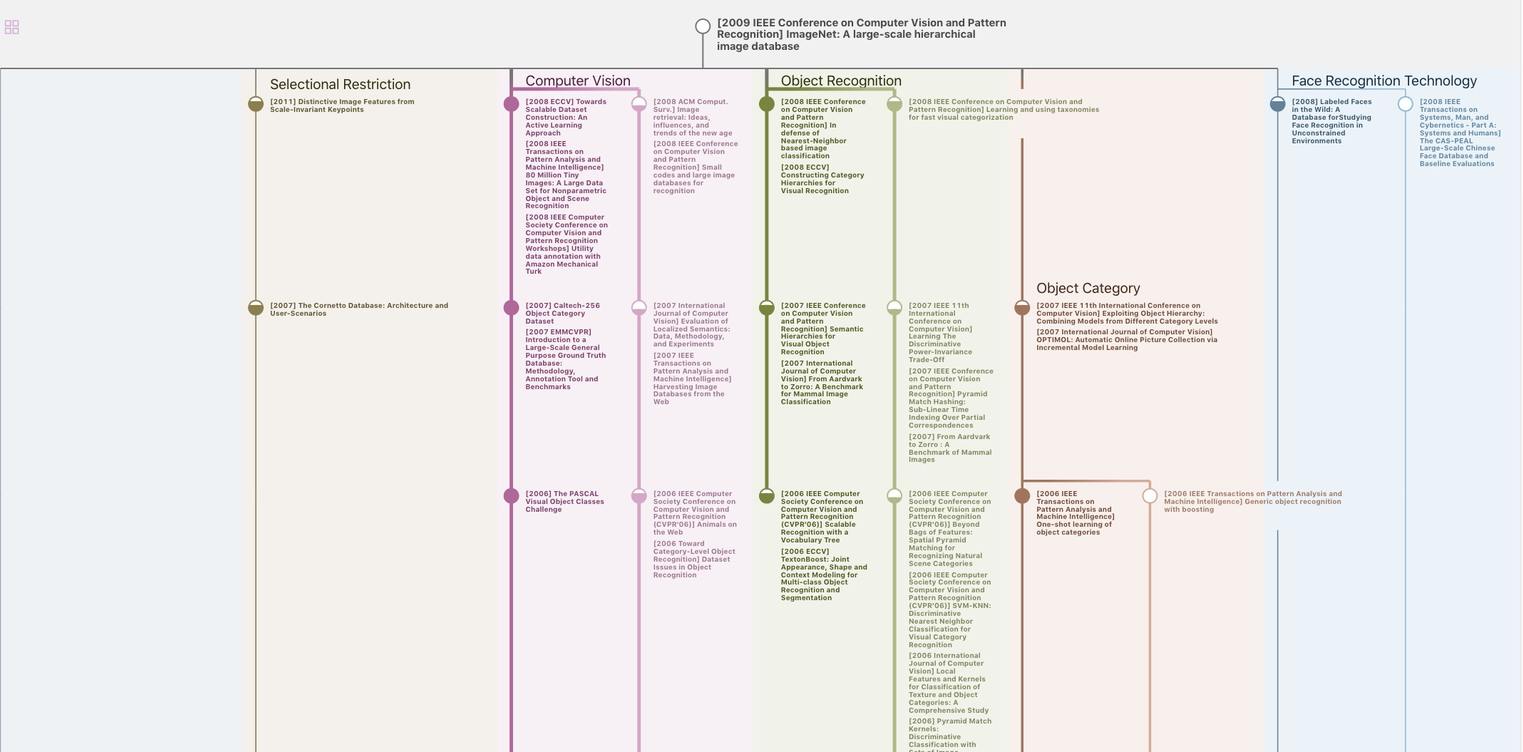
生成溯源树,研究论文发展脉络
Chat Paper
正在生成论文摘要