Classification models and SAR analysis on thromboxane A(2) synthase inhibitors by machine learning methods
SAR AND QSAR IN ENVIRONMENTAL RESEARCH(2022)
摘要
Thromboxane A(2) synthase (TXS) is a promising drug target for cardiovascular diseases and cancer. In this work, we conducted a structure-activity relationship (SAR) study on 526 TXS inhibitors for bioactivity prediction. Three types of descriptors (MACCS fingerprints, ECFP4 fingerprints, and MOE descriptors) were utilized to characterize inhibitors, 24 classification models were developed by support vector machine (SVM), random forest (RF), extreme gradient boosting (XGBoost), and deep neural networks (DNN). Then we reduced the number of fingerprints according to the contribution of descriptors to the models, and constructed 16 extra models on simplified fingerprints. In general, Model_4D built by DNN algorithm and 67 bits MACCS fingerprints performs best. The prediction accuracy of the model on the test set is 0.969, and Matthews correlation coefficient (MCC) is 0.936. The distance between compound and model (d(STD-PRO)) was used to characterize the application domain of the model. In the test set of Model_4D, d(STD-PRO) of 91.5% compounds is lower than the corresponding training set threshold (threshold(0.90) = 0.1055), and the accuracy of these compounds is 0.983. In addition, the important descriptors were summarized and further analyzed. It showed that aromatic nitrogenous heterocyclic groups were beneficial to improve the bioactivity of TXS inhibitors.
更多查看译文
关键词
Thromboxane A(2) synthase (TXS) inhibitor, structure-activity relationship (SAR), extreme gradient boosting (XGBoost), deep neural networks (DNN), applicability domain (AD)
AI 理解论文
溯源树
样例
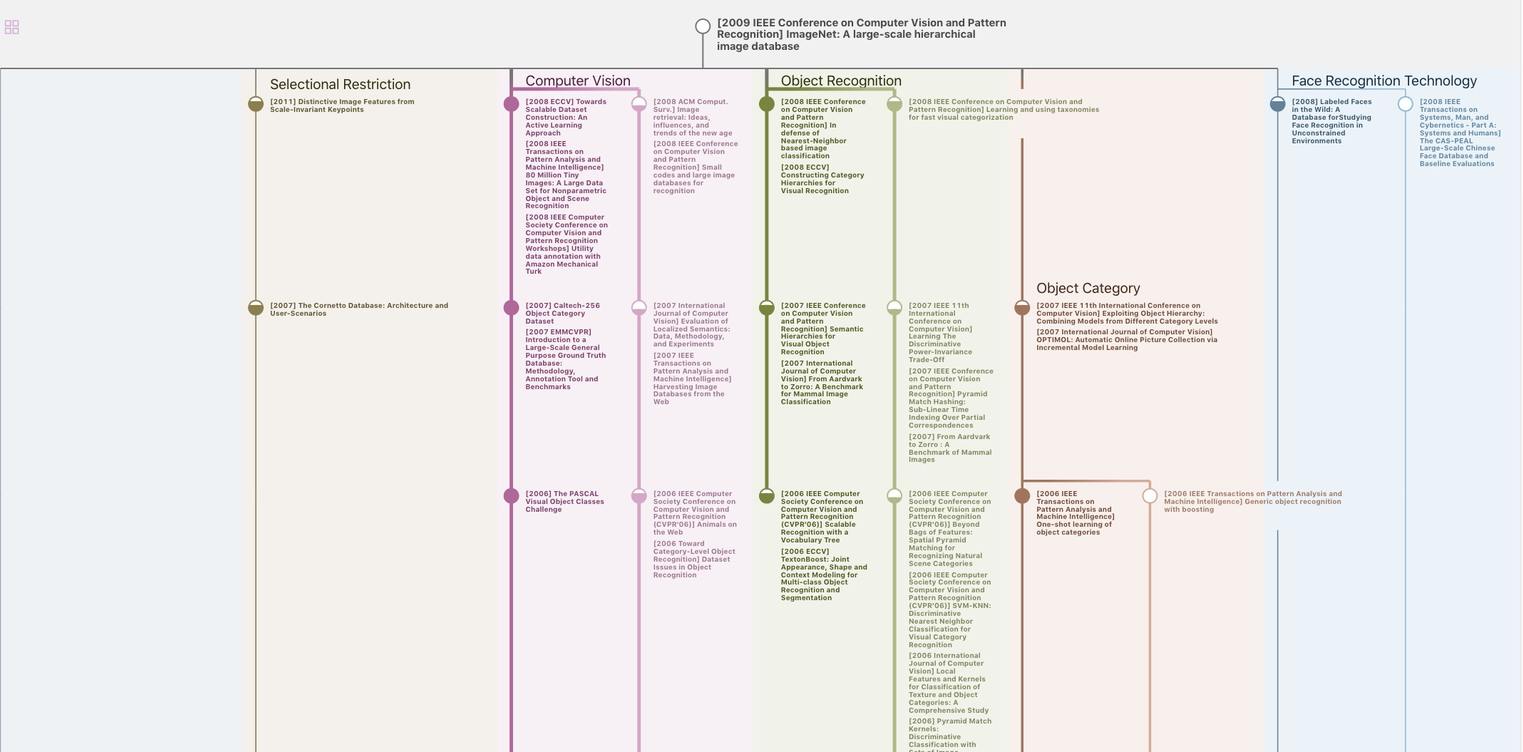
生成溯源树,研究论文发展脉络
Chat Paper
正在生成论文摘要