Diffusion probabilistic modeling of protein backbones in 3D for the motif-scaffolding problem
ICLR 2023(2022)
摘要
Construction of a scaffold structure that supports a desired motif, conferring protein function, shows promise for the design of vaccines and enzymes. But a general solution to this motif-scaffolding problem remains open. Current machine-learning techniques for scaffold design are either limited to unrealistically small scaffolds (up to length 20) or struggle to produce multiple diverse scaffolds. We propose to learn a distribution over diverse and longer protein backbone structures via an E(3)-equivariant graph neural network. We develop SMCDiff to efficiently sample scaffolds from this distribution conditioned on a given motif; our algorithm is the first to theoretically guarantee conditional samples from a diffusion model in the large-compute limit. We evaluate our designed backbones by how well they align with AlphaFold2-predicted structures. We show that our method can (1) sample scaffolds up to 80 residues and (2) achieve structurally diverse scaffolds for a fixed motif.
更多查看译文
关键词
Diffusion Models,Sequential Monte Carlo,Protein Design,Geometric Deep Learning
AI 理解论文
溯源树
样例
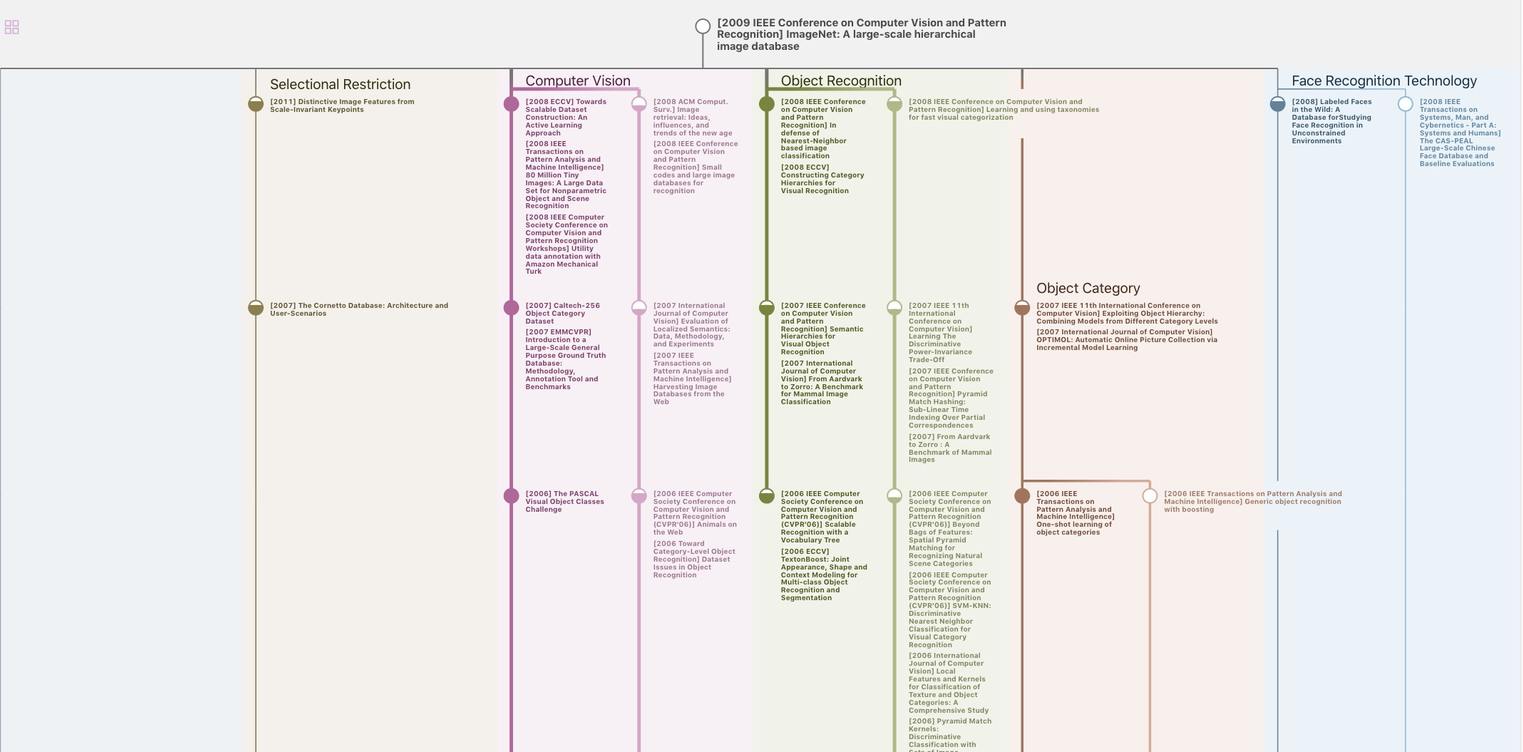
生成溯源树,研究论文发展脉络
Chat Paper
正在生成论文摘要