Depth Estimation Matters Most: Improving Per-Object Depth Estimation for Monocular 3D Detection and Tracking
2022 International Conference on Robotics and Automation (ICRA)(2022)
摘要
Monocular image-based 3D perception has become an active research area in recent years owing to its applications in autonomous driving. Approaches to monocular 3D perception including detection and tracking, however, often yield inferior performance when compared to LiDAR-based techniques. Through systematic analysis, we identified that per-object depth estimation accuracy is a major factor bounding the performance. Motivated by this observation, we propose a multi-level fusion method that combines different representations (RGB and pseudo-LiDAR) and temporal information across multiple frames for objects (tracklets) to enhance per-object depth estimation. Our proposed fusion method achieves the state-of-the-art performance of per-object depth estimation on the Waymo Open Dataset, the KITTI detection dataset, and the KITTI MOT dataset. We further demonstrate that by simply replacing estimated depth with fusion-enhanced depth, we can achieve significant improvements in monocular 3D perception tasks, including detection and tracking.
更多查看译文
关键词
3d detection,tracking,monocular,per-object
AI 理解论文
溯源树
样例
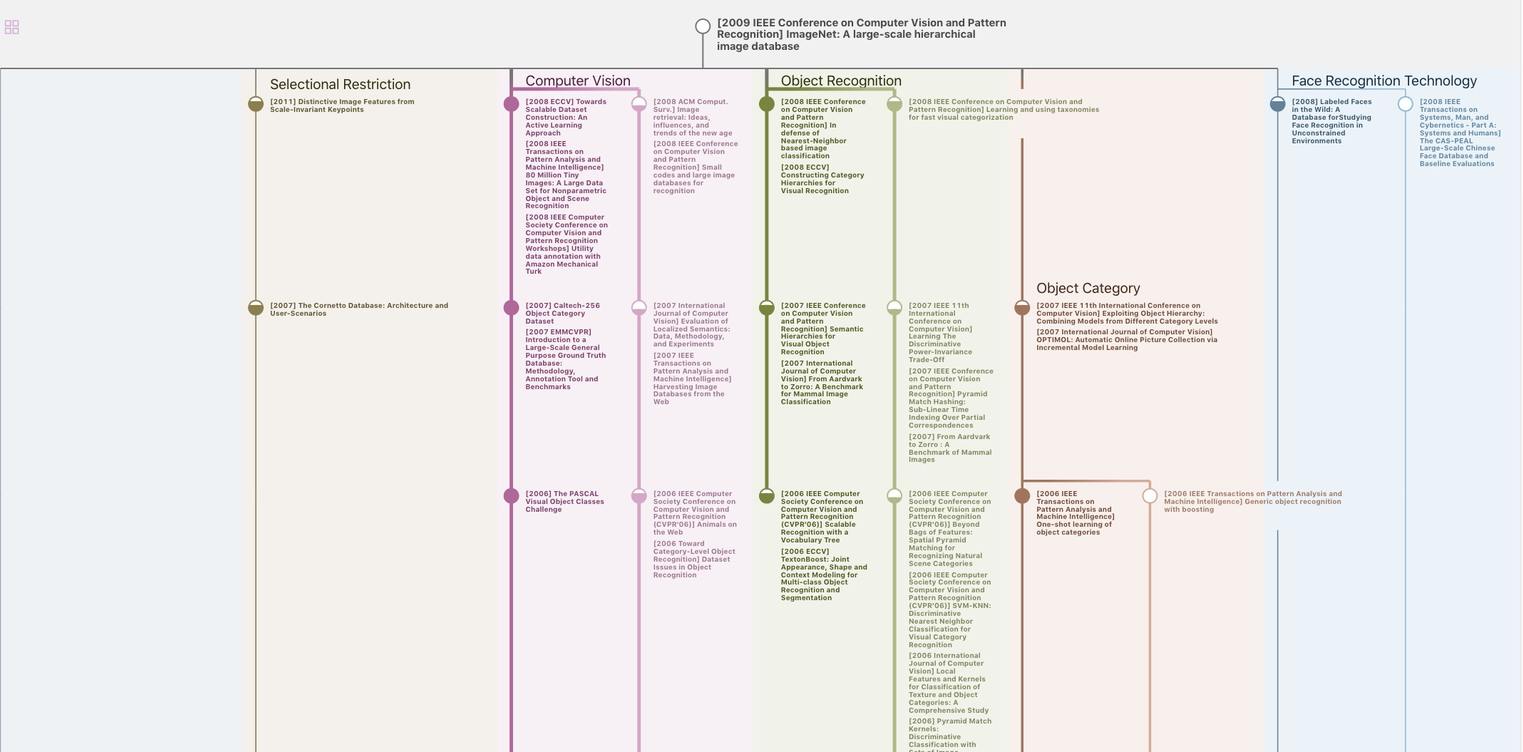
生成溯源树,研究论文发展脉络
Chat Paper
正在生成论文摘要