Robust Self-Tuning Semiparametric PCA for Contaminated Elliptical Distribution.
IEEE Trans. Signal Process.(2022)
摘要
Principal component analysis (PCA) is one of the most popular dimension reduction methods. The usual PCA is known to be sensitive to the presence of outliers, and thus many robust PCA methods have been developed. Among them, the Tyler's M-estimator is shown to be the most robust scatter estimator under the elliptical distribution. However, when the underlying distribution is contaminated and deviates from ellipticity, Tyler's M-estimator might not work well. In this article, we apply the semiparametric theory to propose a robust semiparametric PCA. The merits of our proposal are twofold. First, it is robust to heavy-tailed elliptical distributions as well as robust to non-elliptical outliers. Second, it pairs well with a data-driven tuning procedure, which is based on active ratio and can adapt to different degrees of data outlyingness. Theoretical properties are derived, including the influence functions for various statistical functionals and asymptotic normality. Simulation studies and a data analysis demonstrate the superiority of our method.
更多查看译文
关键词
Active ratio,elliptical distributions,influence function,PCA,robustness,semiparametric theory,Tyler's M-estimator
AI 理解论文
溯源树
样例
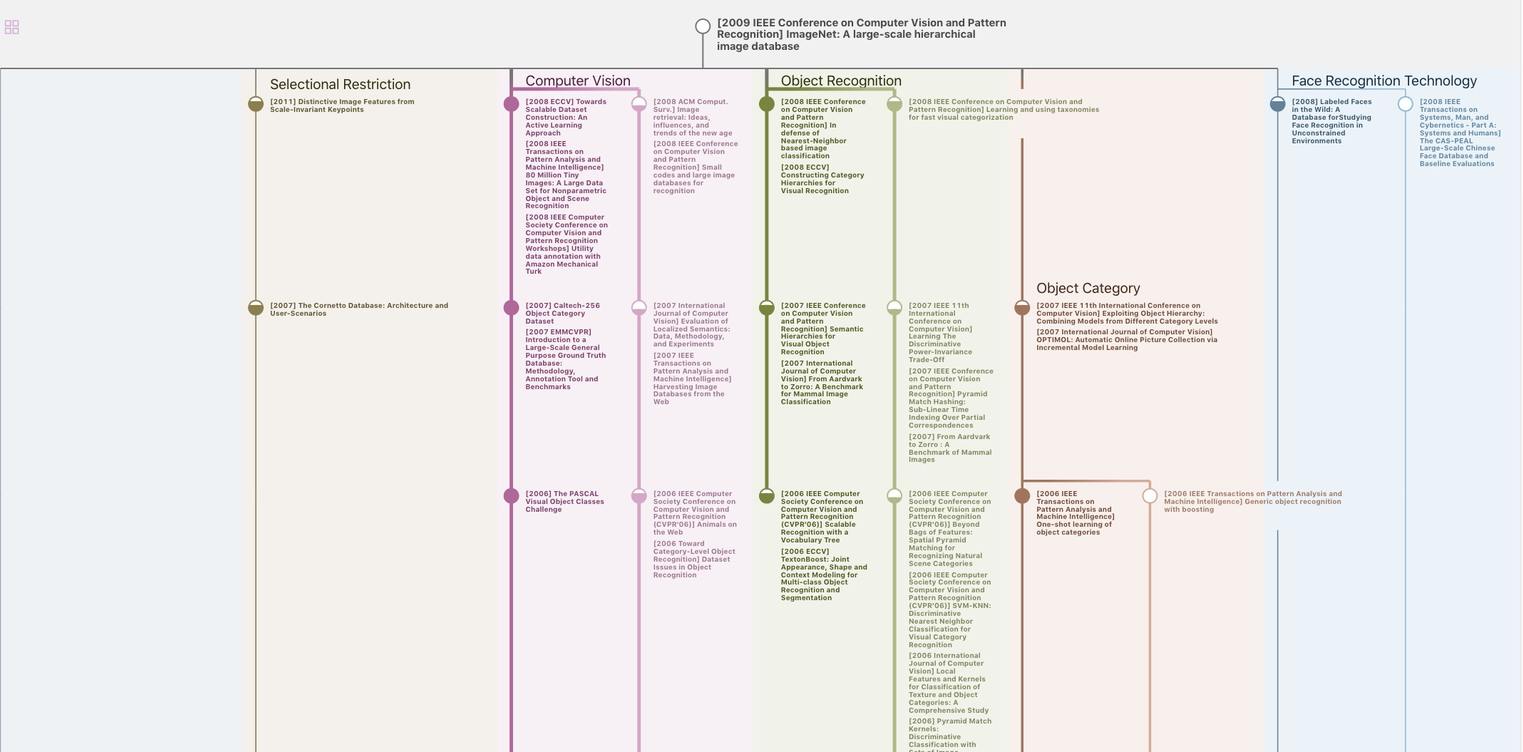
生成溯源树,研究论文发展脉络
Chat Paper
正在生成论文摘要