Graph Rationalization with Environment-based Augmentations
KDD '22: Proceedings of the 28th ACM SIGKDD Conference on Knowledge Discovery and Data Mining(2022)
摘要
Rationale is defined as a subset of input features that best explains or supports the prediction by machine learning models. Rationale identification has improved the generalizability and interpretability of neural networks on vision and language data. In graph applications such as molecule and polymer property prediction, identifying representative subgraph structures named as graph rationales plays an essential role in the performance of graph neural networks. Existing graph pooling and/or distribution intervention methods suffer from the lack of examples to learn to identify optimal graph rationales. In this work, we introduce a new augmentation operation called environment replacement that automatically creates virtual data examples to improve rationale identification. We propose an efficient framework that performs rationale-environment separation and representation learning on the real and augmented examples in latent spaces to avoid the high complexity of explicit graph decoding and encoding. Comparing against recent techniques, experiments on seven molecular and four polymer datasets demonstrate the effectiveness and efficiency of the proposed augmentation-based graph rationalization framework. Data and the implementation of the proposed framework are publicly available https://github.com/liugangcode/GREA.
更多查看译文
关键词
augmentations,environment-based
AI 理解论文
溯源树
样例
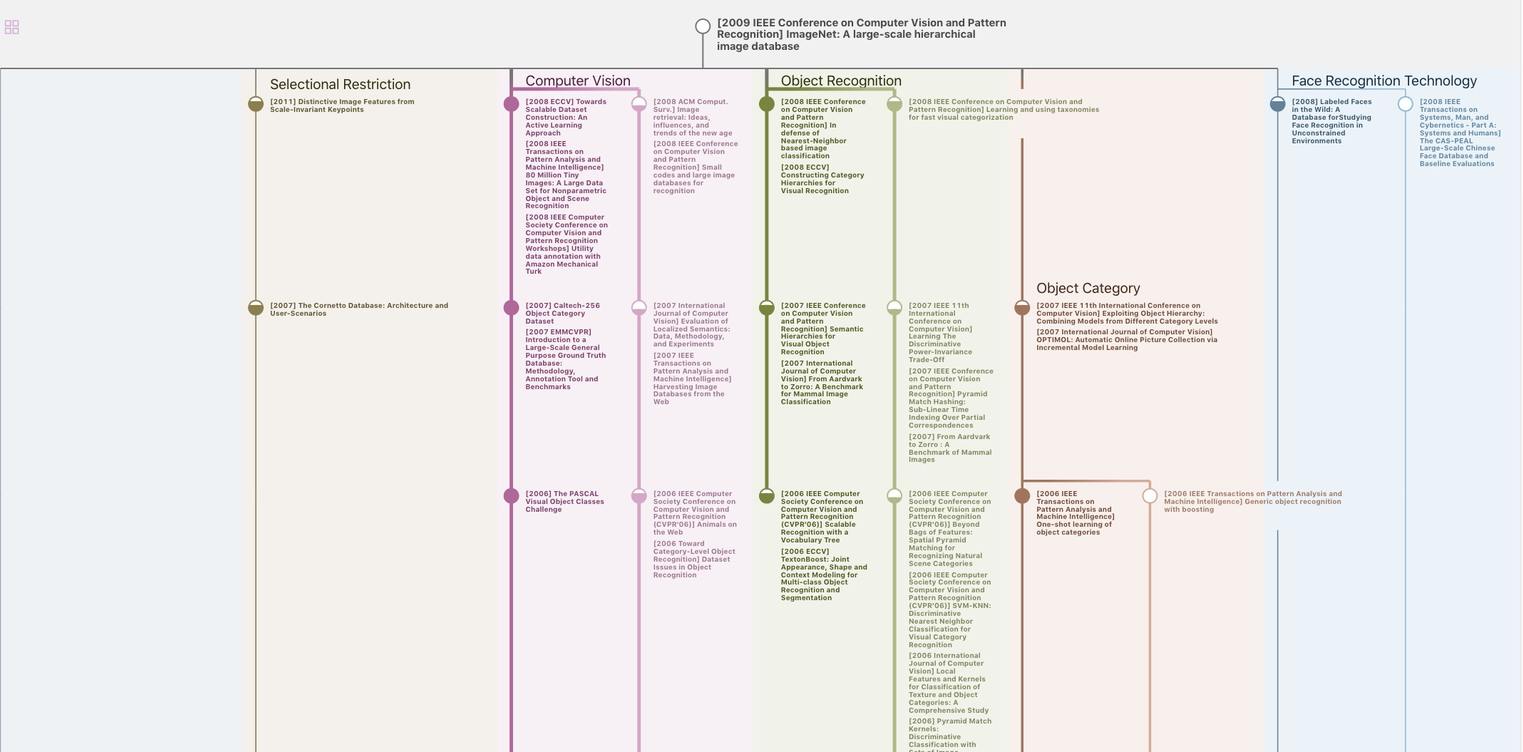
生成溯源树,研究论文发展脉络
Chat Paper
正在生成论文摘要