A Learning- and Scenario-based MPC Design for Nonlinear Systems in LPV Framework with Safety and Stability Guarantees
arxiv(2022)
摘要
This paper presents a learning- and scenario-based model predictive control (MPC) design approach for systems modeled in linear parameter-varying (LPV) framework. Using input-output data collected from the system, a state-space LPV model with uncertainty quantification is first learned through the variational Bayesian inference Neural Network (BNN) approach. The learned probabilistic model is assumed to contain the true dynamics of the system with a high probability and used to generate scenarios which ensure safety for a scenario-based MPC. Moreover, to guarantee stability and enhance performance of the closed-loop system, a parameter-dependent terminal cost and controller, as well as a terminal robust positive invariant set are designed. Numerical examples will be used to demonstrate that the proposed control design approach can ensure safety and achieve desired control performance.
更多查看译文
关键词
mpc design,lpv framework,nonlinear systems,scenario-based
AI 理解论文
溯源树
样例
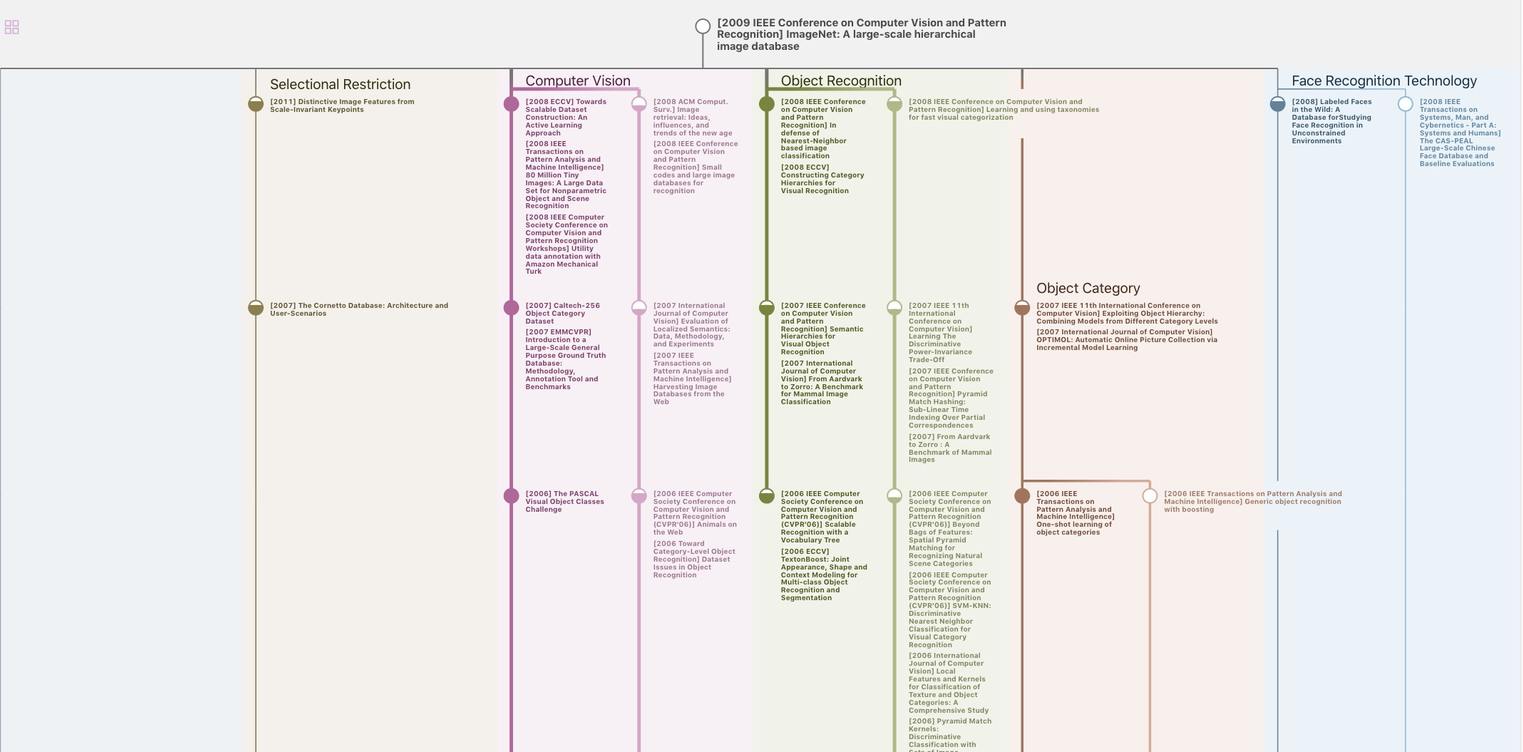
生成溯源树,研究论文发展脉络
Chat Paper
正在生成论文摘要