Saliency Attack: Towards Imperceptible Black-box Adversarial Attack
arxiv(2023)
摘要
Deep neural networks are vulnerable to adversarial examples, even in the black-box setting where the attacker is only accessible to the model output. Recent studies have devised effective black-box attacks with high query efficiency. However, such performance is often accompanied by compromises in attack imperceptibility, hindering the practical use of these approaches. In this article, we propose to restrict the perturbations to a small salient region to generate adversarial examples that can hardly be perceived. This approach is readily compatible with many existing black-box attacks and can significantly improve their imperceptibility with little degradation in attack success rates. Furthermore, we propose the Saliency Attack, a newblack-box attack aiming to refine the perturbations in the salient region to achieve even better imperceptibility. Extensive experiments showthat compared to the state-of-the-art black-box attacks, our approach achievesmuch better imperceptibility scores, including most apparent distortion (MAD), L-0 and L-2 distances, and also obtains significantly better true success rate and effective query number judged by a human-like threshold on MAD. Importantly, the perturbations generated by our approach are interpretable to some extent. Finally, it is also demonstrated to be robust to different detection-based defenses.
更多查看译文
关键词
Adversarial example,black-box adversarial attack,saliency map,deep neural networks
AI 理解论文
溯源树
样例
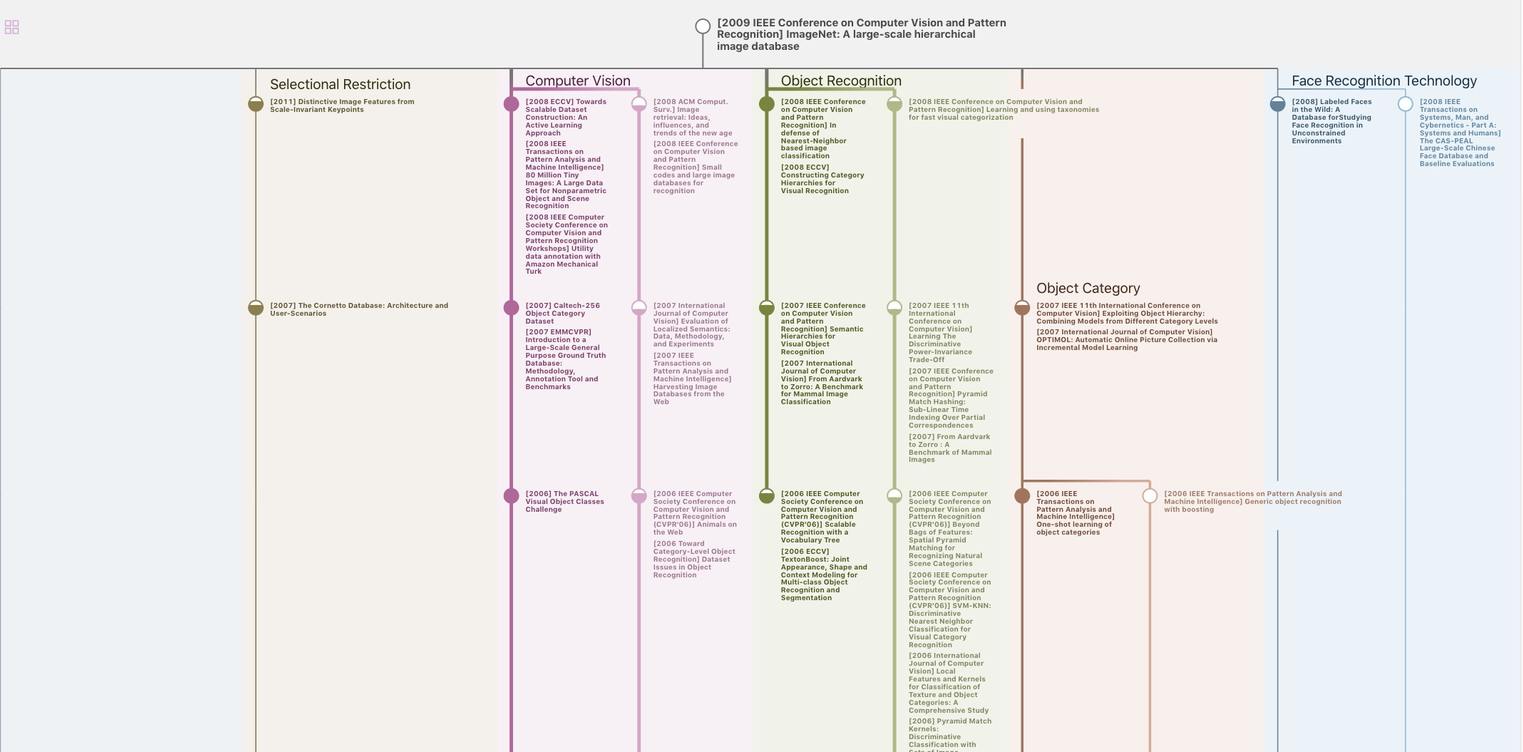
生成溯源树,研究论文发展脉络
Chat Paper
正在生成论文摘要