Learning Fine Scale Dynamics from Coarse Observations via Inner Recurrence
arxiv(2022)
摘要
Recent work has focused on data-driven learning of the evolution of unknown systems via deep neural networks (DNNs), with the goal of conducting long term prediction of the dynamics of the unknown system. In many real-world applications, data from time-dependent systems are often collected on a time scale that is coarser than desired, due to various restrictions during the data acquisition process. Consequently, the observed dynamics can be severely under-sampled and do not reflect the true dynamics of the underlying system. This paper presents a computational technique to learn the fine-scale dynamics from such coarsely observed data. The method employs inner recurrence of a DNN to recover the fine-scale evolution operator of the underlying system. In addition to mathematical justification, several challenging numerical examples, including unknown systems of both ordinary and partial differential equations, are presented to demonstrate the effectiveness of the proposed method.
更多查看译文
关键词
fine scale dynamics,coarse observations,learning
AI 理解论文
溯源树
样例
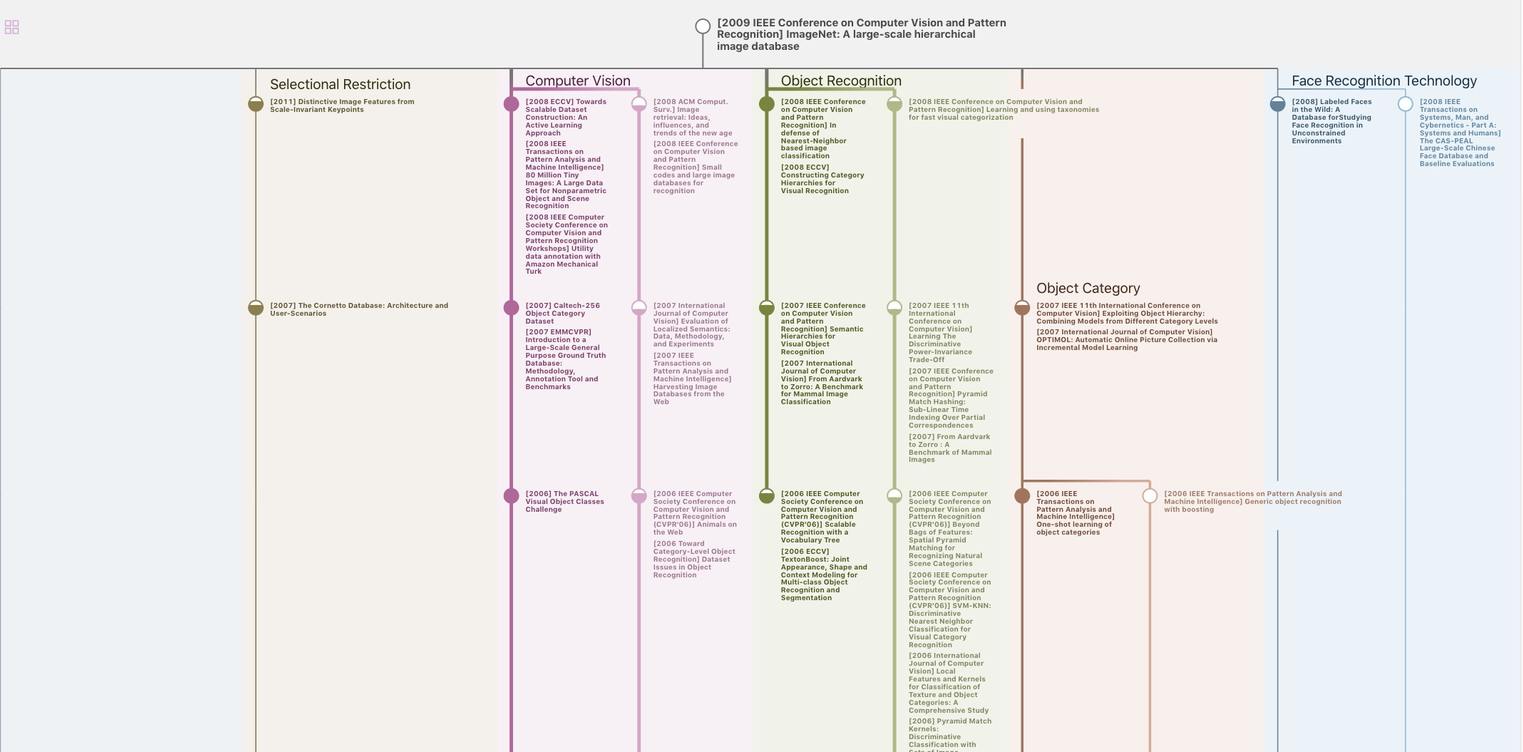
生成溯源树,研究论文发展脉络
Chat Paper
正在生成论文摘要