Mean Field Approximations via Log-Concavity
INTERNATIONAL MATHEMATICS RESEARCH NOTICES(2023)
摘要
We propose a new approach to deriving quantitative mean field approximations for any probability measure $P$ on $\mathbb {R}<^>{n}$ with density proportional to $e<^>{f(x)}$, for $f$ strongly concave. We bound the mean field approximation for the log partition function $\log \int e<^>{f(x)}dx$ in terms of $\sum _{i \neq j}\mathbb {E}_{Q<^>{*}}|\partial _{ij}f|<^>{2}$, for a semi-explicit probability measure $Q<^>{*}$ characterized as the unique mean field optimizer, or equivalently as the minimizer of the relative entropy $H(\cdot \,|\,P)$ over product measures. This notably does not involve metric-entropy or gradient-complexity concepts which are common in prior work on nonlinear large deviations. Three implications are discussed, in the contexts of continuous Gibbs measures on large graphs, high-dimensional Bayesian linear regression, and the construction of decentralized near-optimizers in high-dimensional stochastic control problems. Our arguments are based primarily on functional inequalities and the notion of displacement convexity from optimal transport.
更多查看译文
AI 理解论文
溯源树
样例
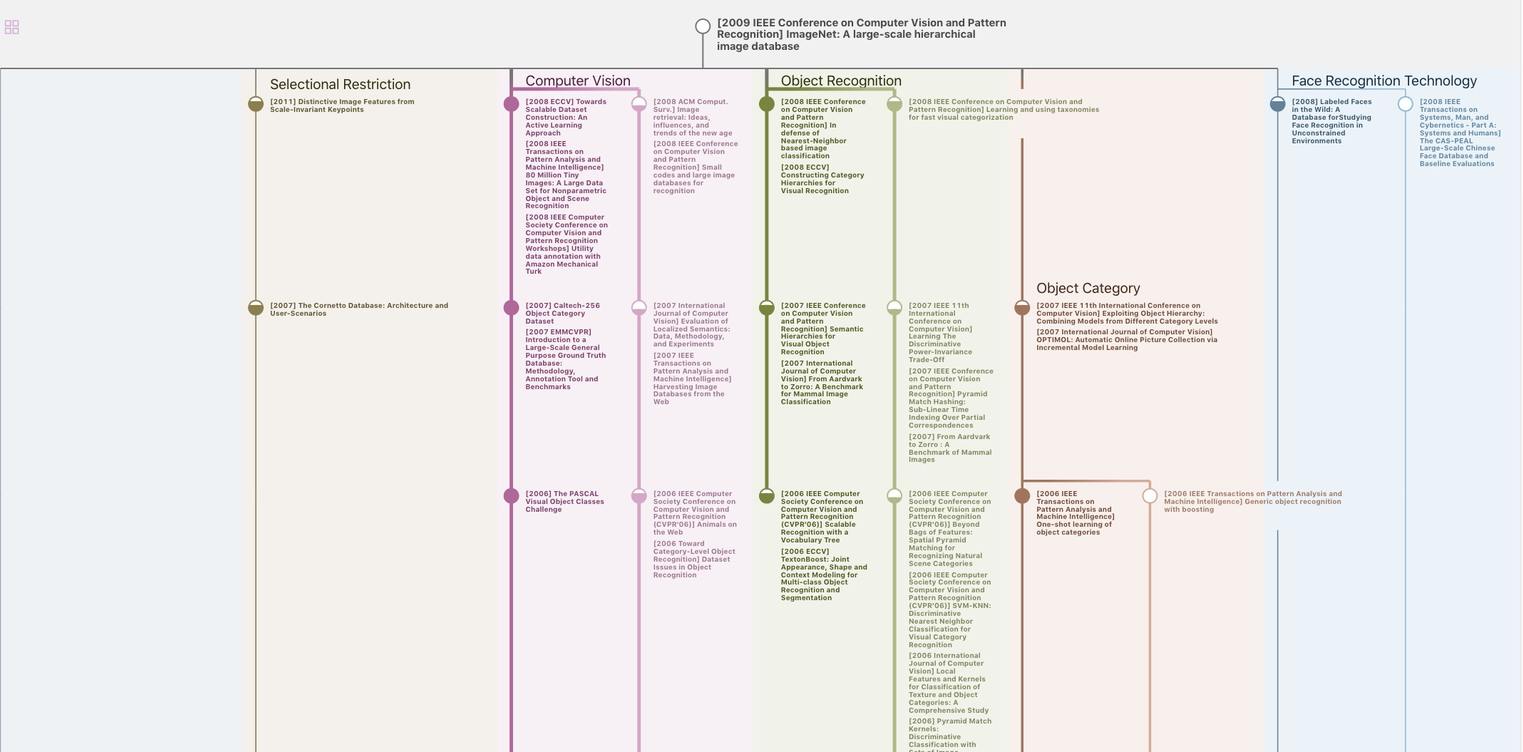
生成溯源树,研究论文发展脉络
Chat Paper
正在生成论文摘要