ConsAlign: simultaneous RNA structural aligner based on rich transfer learning and thermodynamic ensemble model of alignment scoring
Bioinformatics(2022)
摘要
Motivation: To capture structural homology in RNAs, predicting RNA structural alignments has been a fundamental framework around RNA science. Learning simultaneous RNA structural alignments in their rich scoring is an undeveloped subject because evaluating them is computationally expensive. Results: We developed ConsTrain, a gradient-based machine learning method for rich structural alignment scoring. We also implemented ConsAlign, a simultaneous RNA structural aligner composed of ConsTrain's learned scoring parameters. To aim for better structural alignment quality, ConsAlign employs (1) transfer learning from well-defined scoring models and (2) the ensemble model between the ConsTrain model and a mature thermodynamic scoring model. Keeping comparable running time, ConsAlign demonstrated competitive alignment prediction quality among current RNA structural aligners. Availability and implementation: Our code and our data are freely available at https://github.com/ heartsh/consalign. Contact: heartsh@heartsh.io Supplementary information: Supplementary data are available at Bioinformatics
### Competing Interest Statement
The authors have declared no competing interest.
更多查看译文
AI 理解论文
溯源树
样例
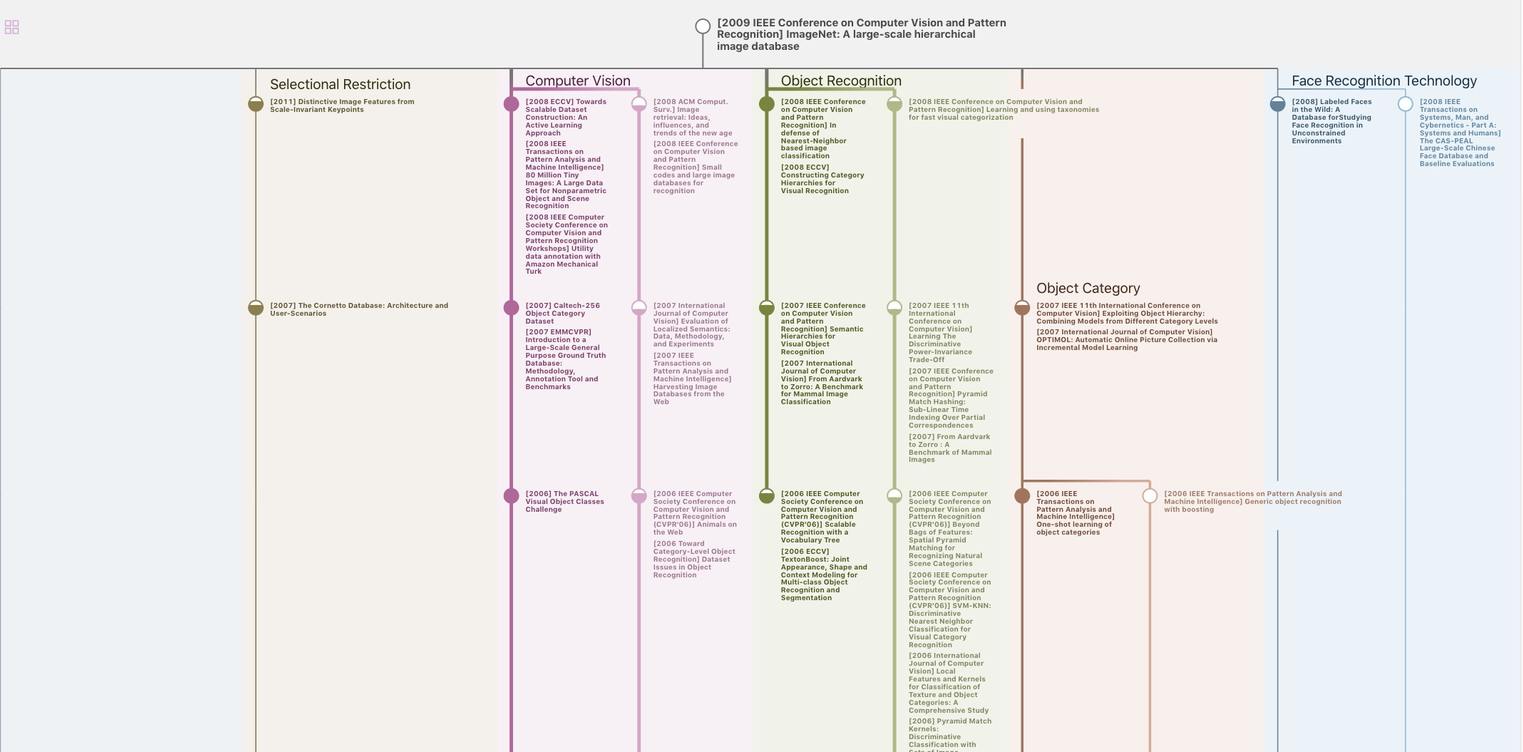
生成溯源树,研究论文发展脉络
Chat Paper
正在生成论文摘要