GeneVector: Identification of transcriptional programs using dense vector representations defined by mutual information
Nature Communications(2023)
摘要
Deciphering individual cell phenotypes from cell-specific transcriptional processes requires high dimensional single cell RNA sequencing. However, current dimensionality reduction methods aggregate sparse gene information across cells, without directly measuring the relationships that exist between genes. By performing dimensionality reduction with respect to gene co-expression, low-dimensional features can model these gene-specific relationships and leverage shared signal to overcome sparsity. We describe GeneVector, a scalable framework for dimensionality reduction implemented as a vector space model using mutual information between gene expression. Unlike other methods, including principal component analysis and variational autoencoders, GeneVector uses latent space arithmetic in a lower dimensional gene embedding to identify transcriptional programs and classify cell types. In this work, we show in four single cell RNA-seq datasets that GeneVector was able to capture phenotypespecific pathways, perform batch effect correction, interactively annotate cell types, and identify pathway variation with treatment over time.
### Competing Interest Statement
B.D.G. has received honoraria for speaking engagements from Merck, Bristol-Meyers Squibb and Chugai Pharmaceuticals; has received research funding from Bristol-Meyers Squibb; and has been a compensated consultant for PMV Pharma and Rome Therapeutics of which he is a co-founder. S.P.S. reports personal fees from Imagia Canexia Inc. S.S.F. is a co-inventor on provisional patent application No.62/866,261 related to prediction of melanoma immunotherapy outcomes.
更多查看译文
关键词
transcriptional programs,dense vector representations,mutual information
AI 理解论文
溯源树
样例
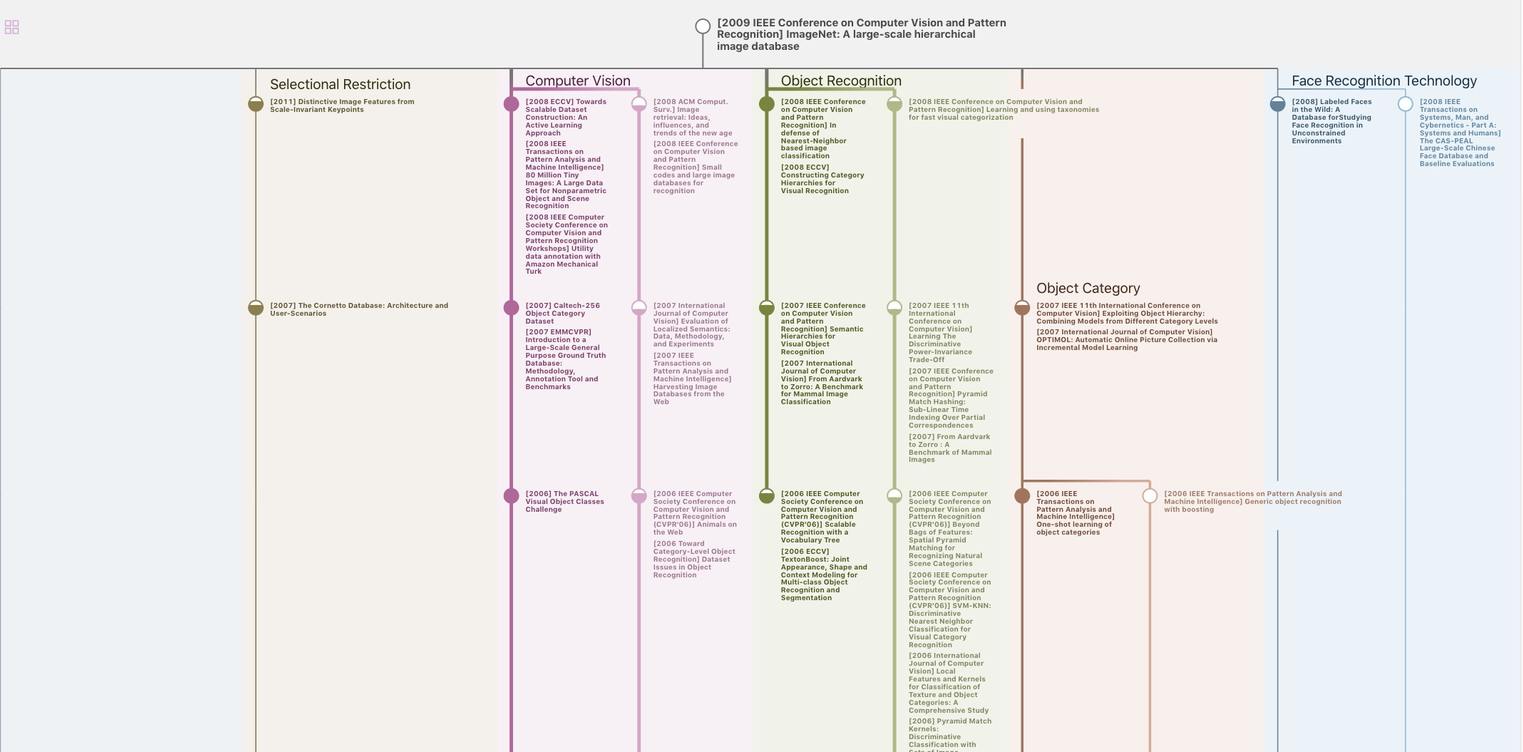
生成溯源树,研究论文发展脉络
Chat Paper
正在生成论文摘要