Parametric quantile autoregressive moving average models with exogenous terms
arxiv(2023)
摘要
Parametric autoregressive moving average models with exogenous terms (ARMAX) have been widely used in the literature. Usually, these models consider a conditional mean or median dynamics, which limits the analysis. In this paper, we introduce a class of quantile ARMAX models based on log-symmetric distributions. This class is indexed by quantile and dispersion parameters. It not only accommodates the possibility to model bimodal and/or light/heavy-tailed distributed data but also accommodates heteroscedasticity. We estimate the model parameters by using the conditional maximum likelihood method. Furthermore, we carry out an extensive Monte Carlo simulation study to evaluate the performance of the proposed models and the estimation method in retrieving the true parameter values. Finally, the proposed class of models and the estimation method are applied to a dataset on the competition “M5 Forecasting - Accuracy” that corresponds to the daily sales history of several Walmart products. The results indicate that the proposed log-symmetric quantile ARMAX models have good performance in terms of model fitting and forecasting.
更多查看译文
关键词
ARMAX models,Log-symmetric distributions,Monte Carlo simulation,Walmart sales data
AI 理解论文
溯源树
样例
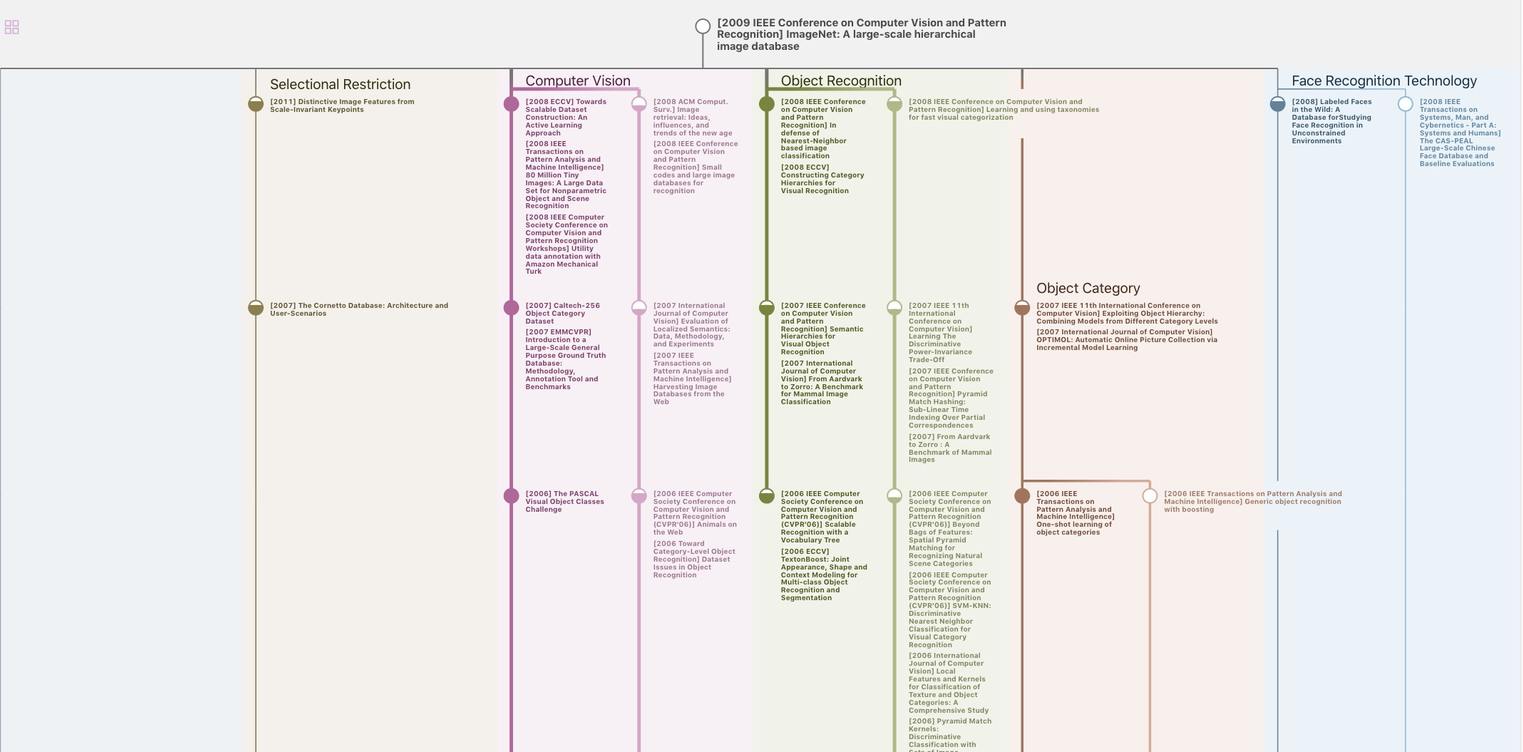
生成溯源树,研究论文发展脉络
Chat Paper
正在生成论文摘要