Automated Measurement of Pancreatic Fat Deposition on Dixon MRI Using nnU-Net
Journal of magnetic resonance imaging : JMRI(2023)
摘要
Background Pancreatic fat accumulation may cause or aggravate the process of acute pancreatitis, beta-cell dysfunction, T2DM disease, and even be associated with pancreatic tumors. The pathophysiology of fatty pancreas remains overlooked and lacks effective imaging diagnostics. Purpose To automatically measure the distribution of pancreatic fat deposition on Dixon MRI in multicenter/population datasets using nnU-Net models. Study Type Retrospective. Population A total of 176 obese/nonobese subjects (90 males, 86 females; mean age, 27.2 +/- 19.7) were enrolled, including a training set (N = 132) and a testing set (N = 44). Field Strength/Sequence A 3 T and 1.5 T/gradient echo T-1 dual-echo Dixon. Assessment The segmentation results of four types of nnU-Net models were compared using dice similarity coefficient (DSC), positive predicted value (PPV), and sensitivity. The ground truth was the manual delineation by two radiologists according to in-phase (IP) and opposed-phase (OP) images. Statistical Tests The group difference of segmentation results of four models were assessed by the Kruskal-Wallis H test with Dunn-Bonferroni comparisons. The interobserver agreement of pancreatic fat fraction measurements across three observers and test-retest reliability of human and machine were assessed by intragroup correlation coefficient (ICC). P < 0.05 was considered statistically significant. Results The three-dimensional (3D) dual-contrast model had significantly improved performance than 2D dual-contrast (DSC/sensitivity) and 3D one-contrast (IP) models (DSC/PPV/sensitivity) and had less errors than 3D one-contrast (OP) model according to higher DSC and PPV (not significant), with a mean DSC of 0.9158, PPV of 0.9105 and sensitivity of 0.9232 in the testing set. The test-retest ICC of this model was above 0.900 in all pancreatic regions, exceeded human. Data Conclusion 3D Dual-contrast nnU-Net aided segmentation of pancreas on Dixon images appears to be adaptable to multicenter/population datasets. It fully automates the assessment of pancreatic fat distribution and has high reliability. Evidence Level 3 Technical Efficacy Stage 3
更多查看译文
关键词
pancreas,ectopic fat deposition,fat quantification,deep learning,nnU-Net,T2DM
AI 理解论文
溯源树
样例
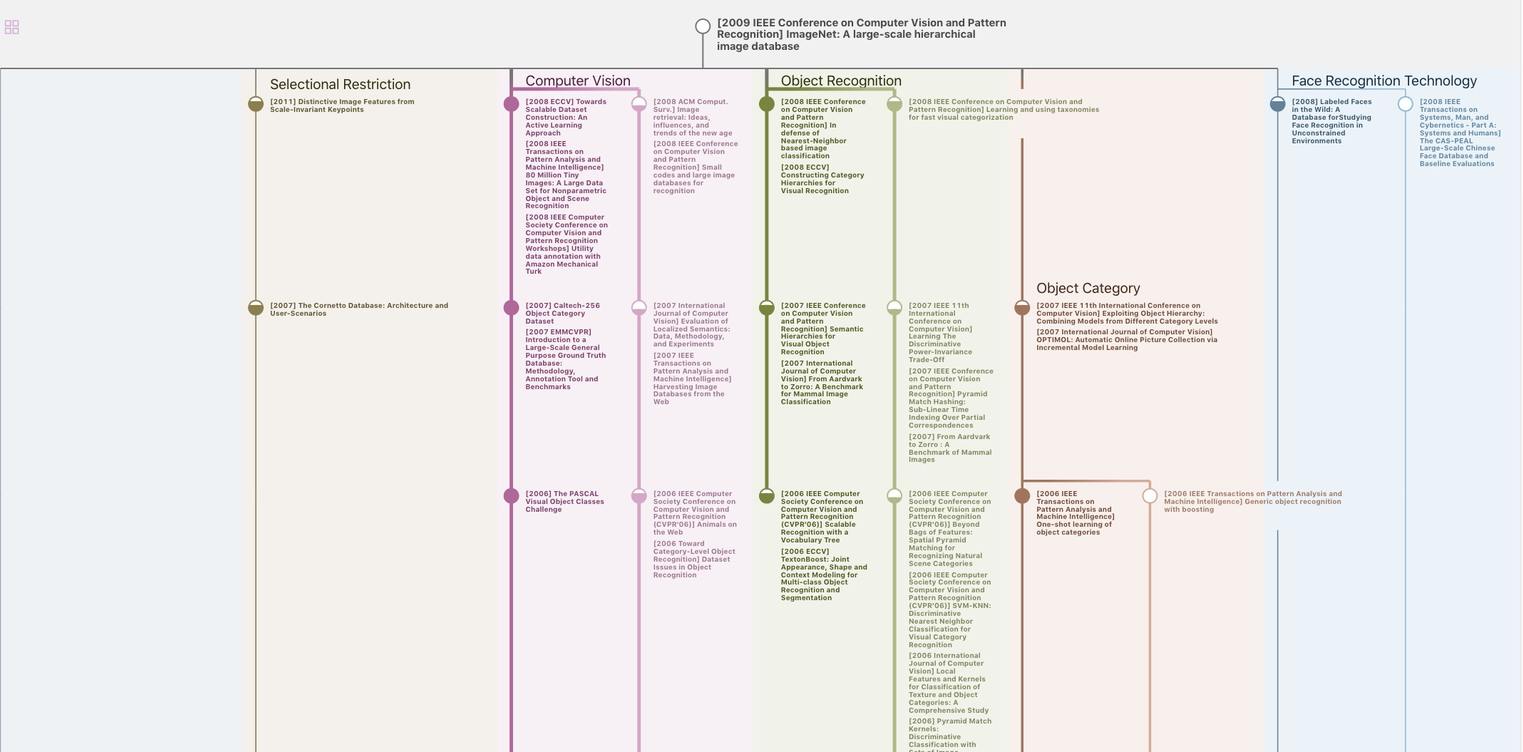
生成溯源树,研究论文发展脉络
Chat Paper
正在生成论文摘要