CALEB: A Conditional Adversarial Learning Framework to enhance bot detection
DATA & KNOWLEDGE ENGINEERING(2024)
摘要
The high growth of Online Social Networks (OSNs) over the last few years has allowed automated accounts, known as social bots, to gain ground. As highlighted by other researchers, many of these bots have malicious purposes and tend to mimic human behavior, posing high-level security threats on OSN platforms. Moreover, recent studies have shown that social bots evolve over time by reforming and reinventing unforeseen and sophisticated characteristics, making them capable of evading the current machine learning state-of-the-art bot detection systems. This work is motivated by the critical need to establish adaptive bot detection methods in order to proactively capture unseen evolved bots towards healthier OSNs interactions. In contrast with most earlier supervised ML approaches which are limited by the inability to effectively detect new types of bots, this paper proposes CALEB, a robust end-to-end proactive framework based on the Conditional Generative Adversarial Network (CGAN) and its extension, Auxiliary Classifier GAN (AC-GAN), to simulate bot evolution by creating realistic synthetic instances of different bot types. These simulated evolved bots augment existing bot datasets and therefore enhance the detection of emerging generations of bots before they even appear. Furthermore, we show that our augmentation approach overpasses other earlier augmentation techniques which fail at simulating evolving bots. Extensive experimentation on well established public bot datasets, show that our approach offers a performance boost of up to 10% regarding the detection of new unseen bots. Finally, the use of the AC-GAN Discriminator as a bot detector, has outperformed former ML approaches, showcasing the efficiency of our end to end framework.
更多查看译文
关键词
Online social networks,Social bot detection,Bot evolution,Adversarial machine learning,Conditional generative adversarial networks,Twitter
AI 理解论文
溯源树
样例
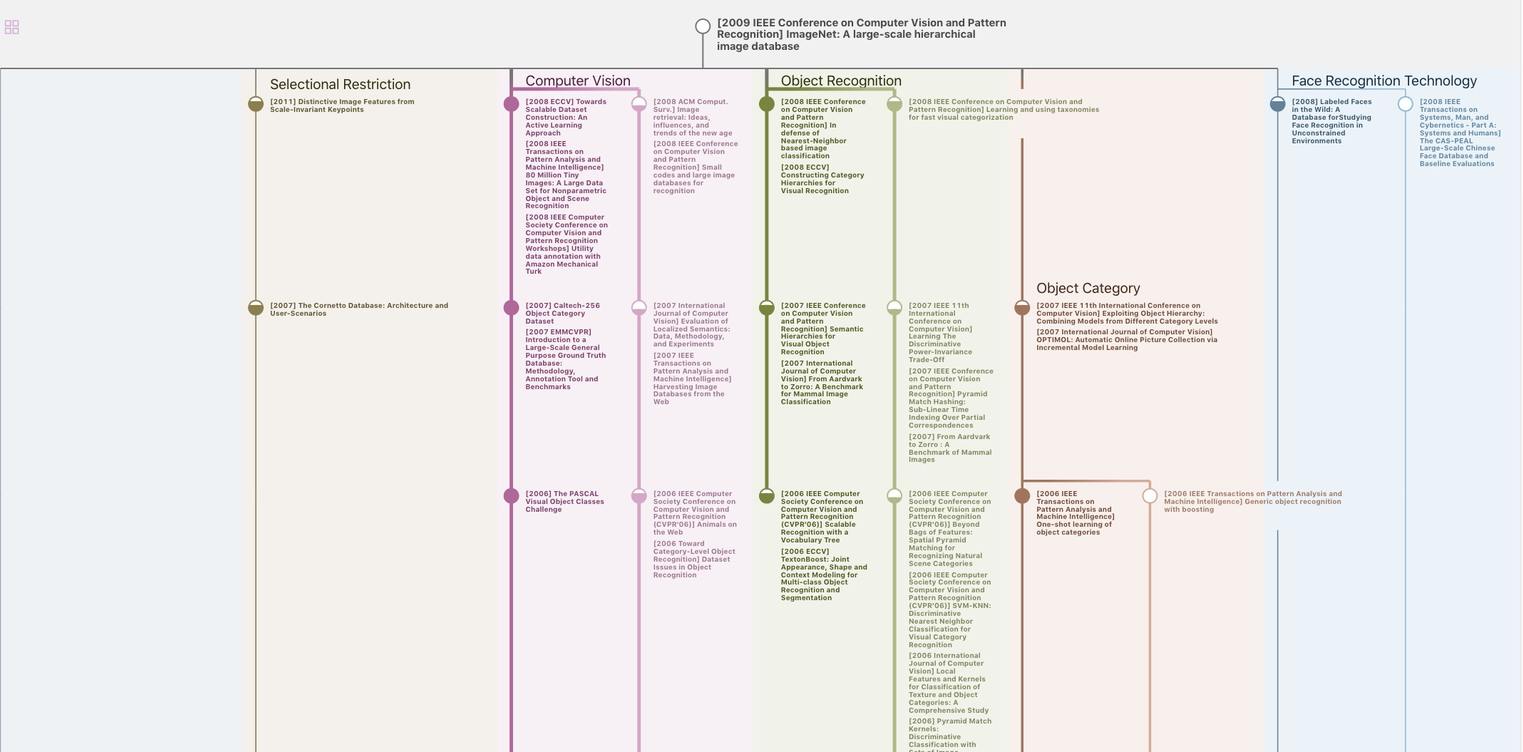
生成溯源树,研究论文发展脉络
Chat Paper
正在生成论文摘要