Calibration of nuclear charge density distribution by back-propagation neural networks
arxiv(2022)
摘要
Based on the back-propagation neural networks and density functional theory, a supervised learning is performed firstly to generate the nuclear charge density distributions. The charge density is further calibrated to the experimental charge radii by a composite loss function. It is found that, when the parity, pairing, and shell effects are taken into account, about $96\%$ of the nuclei in the validation set fall within two standard deviations of the predicted charge radii. The calibrated charge density is then mapped to the matter density, and further mapped to the binding energies according to the Hohenberg-Kohn theorem. It provides an improved description of some nuclei in both binding energies and charge radii. Moreover, the anomalous overbinding in $^{48}$Ca implies the existence of an indispensable beyond-mean-field effect.
更多查看译文
关键词
nuclear charge density distribution,neural networks,calibration,back-propagation
AI 理解论文
溯源树
样例
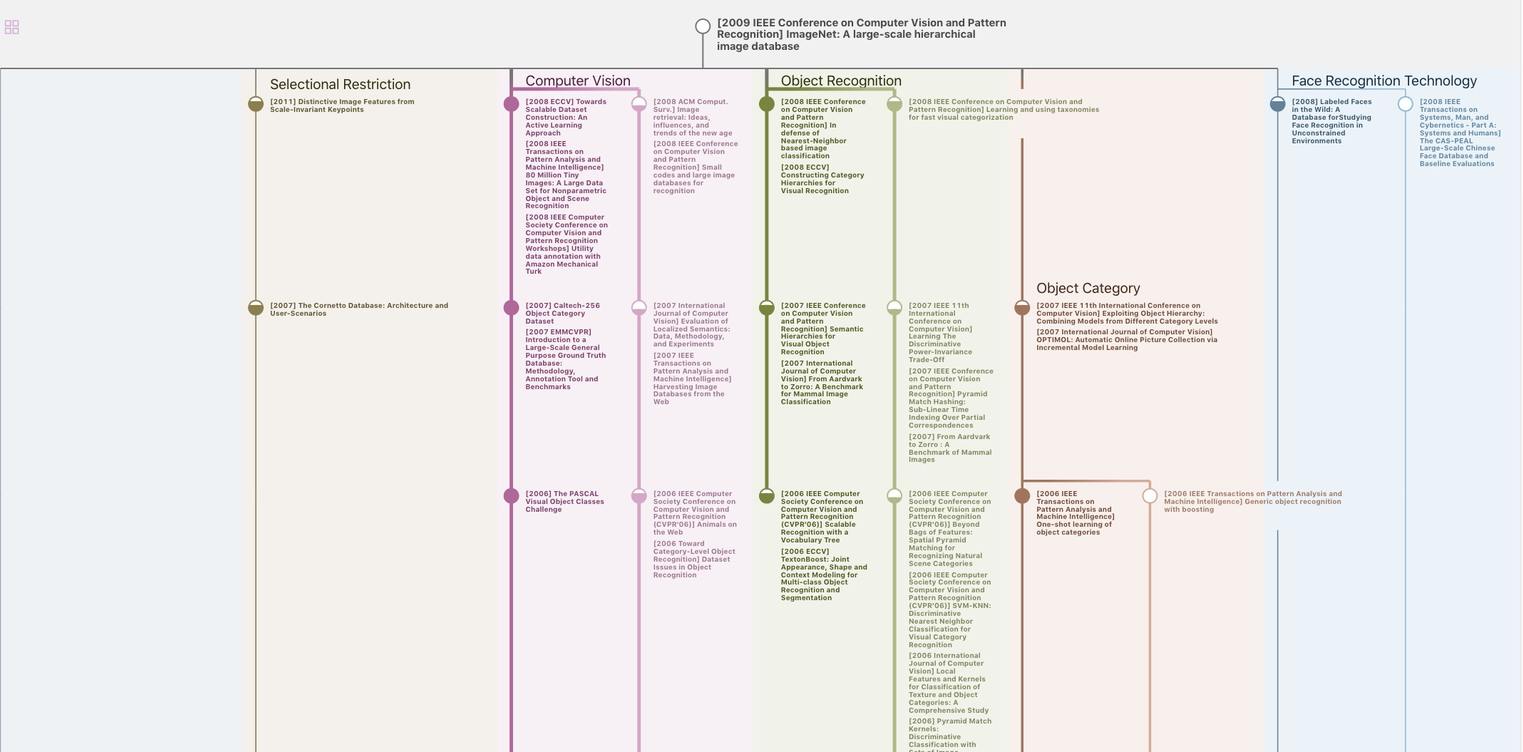
生成溯源树,研究论文发展脉络
Chat Paper
正在生成论文摘要