Gmml is all you need
2023 IEEE INTERNATIONAL CONFERENCE ON IMAGE PROCESSING, ICIP(2023)
摘要
Vision transformers (ViTs) have generated significant interest in the computer vision community because of their flexibility in exploiting contextual information, whether it is sharply confined local, or long range global. However, they are known to be data hungry and therefore often pretrained on large-scale datasets, e.g. JFT-300M or ImageNet. An ideal learning method would perform best regardless of the size of the dataset, a property lacked by current learning methods, with merely a few existing works studying ViTs with limited data. We propose Group Masked Model Learning (GMML), a self-supervised learning (SSL) method that is able to train ViTs and achieve state-of-the-art (SOTA) performance when pre-trained with limited data. The GMML uses the information conveyed by all concepts in the image. This is achieved by manipulating randomly groups of connected tokens, successively covering different meaningful parts of the image content, and then recovering the hidden information from the visible part of the concept. Unlike most of the existing SSL approaches, GMML does not require momentum encoder, nor relies on careful implementation details such as large batches and gradient stopping. Pretraining, finetuning, and evaluation codes are available under: https://github.com/GMML.
更多查看译文
关键词
Self-supervised Learning,Vision Transformers,Group Masked Model Learning,Deep Learning
AI 理解论文
溯源树
样例
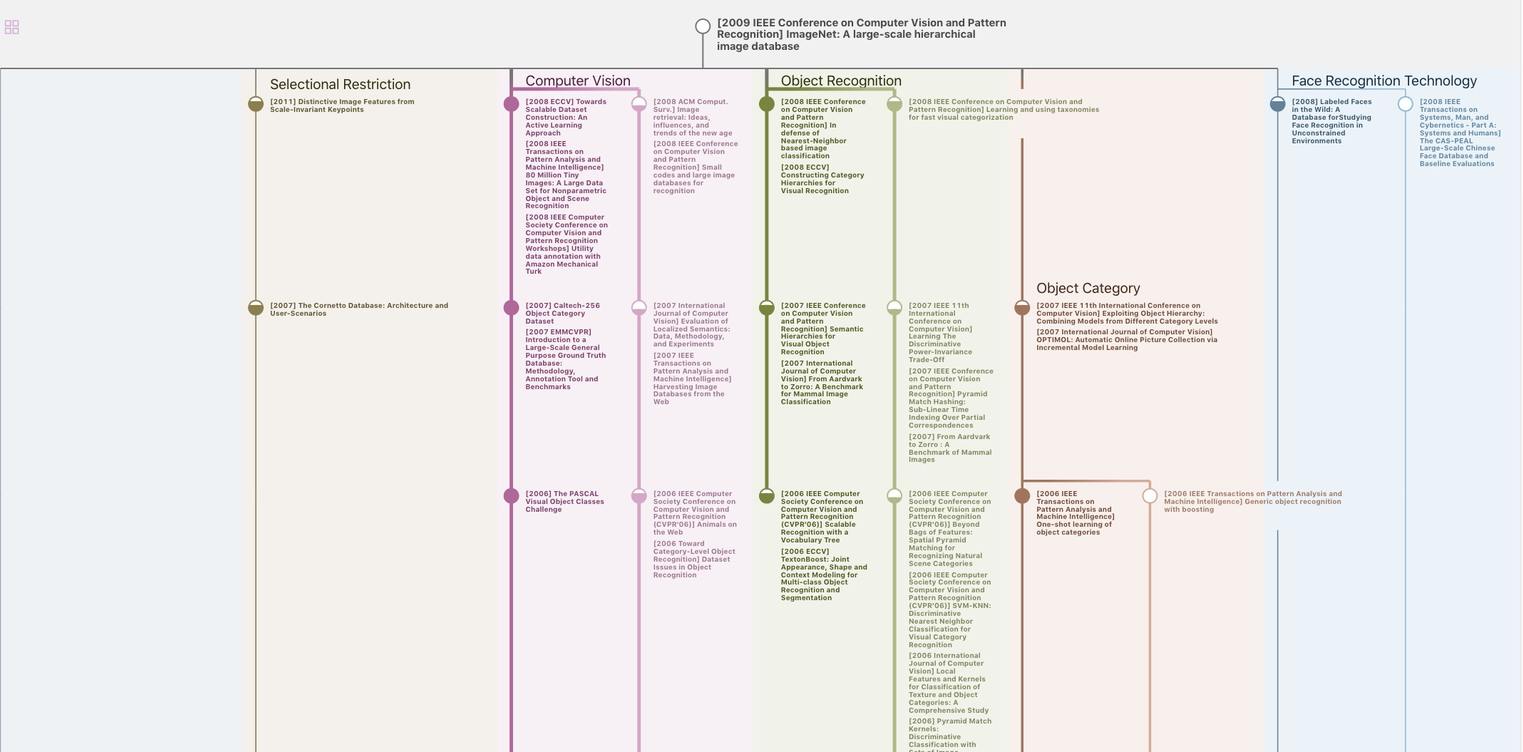
生成溯源树,研究论文发展脉络
Chat Paper
正在生成论文摘要