Provable Benefits of Representational Transfer in Reinforcement Learning
ICLR 2023(2022)
摘要
We study the problem of representational transfer in RL, where an agent first pretrains in a number of source tasks to discover a shared representation, which is subsequently used to learn a good policy in a target task. We propose a new notion of task relatedness between source and target tasks, and develop a novel approach for representational transfer under this assumption. Concretely, we show that given generative access to source tasks, we can discover a representation, using which subsequent linear RL techniques quickly converge to a near-optimal policy, with only online access to the target task. The sample complexity is close to knowing the ground truth features in the target task, and comparable to prior representation learning results in the source tasks. We complement our positive results with lower bounds without generative access, and validate our findings with empirical evaluation on rich observation MDPs that require deep exploration.
更多查看译文
关键词
reinforcement learning theory,representation learning,transfer learning
AI 理解论文
溯源树
样例
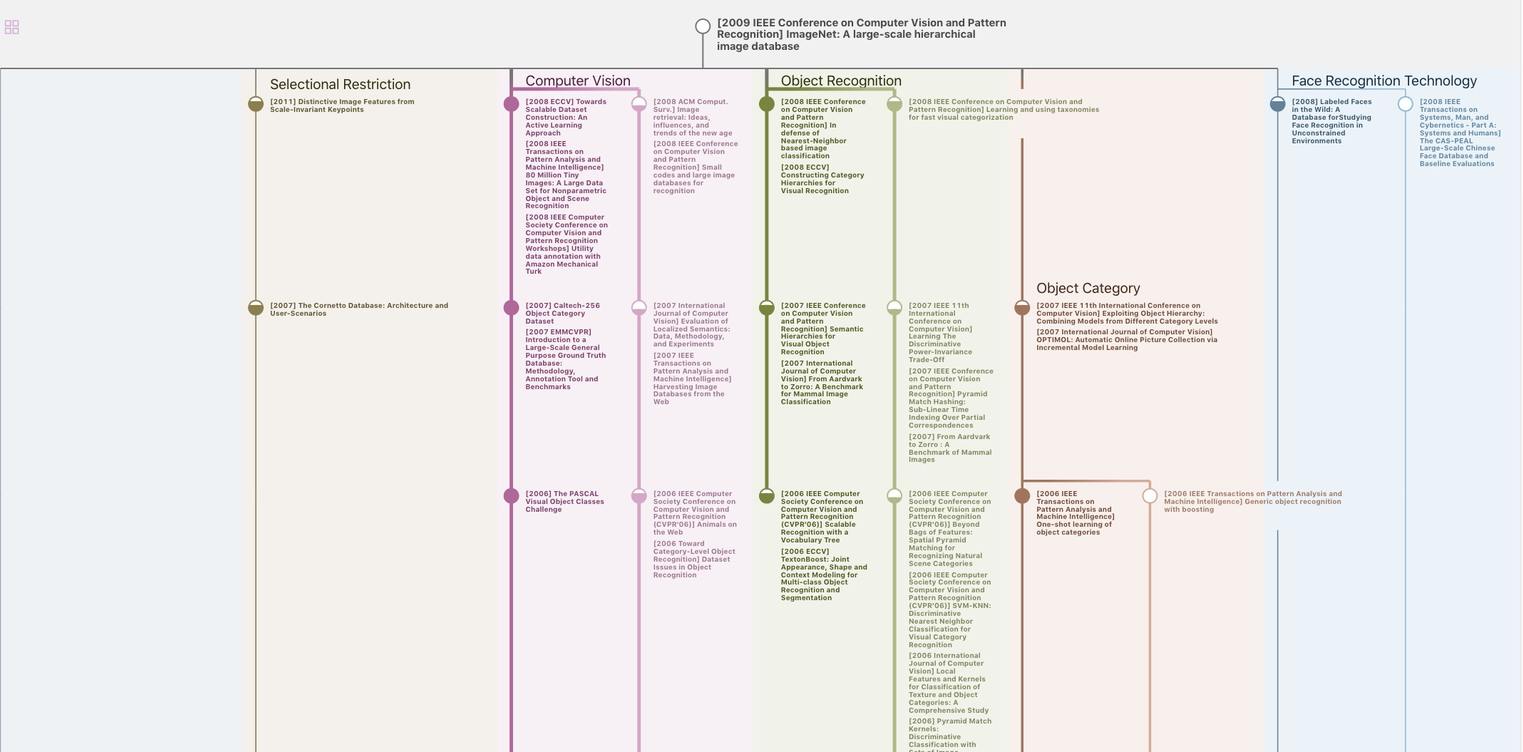
生成溯源树,研究论文发展脉络
Chat Paper
正在生成论文摘要