Capturing Graphs with Hypo-Elliptic Diffusions
NeurIPS 2022(2022)
摘要
Convolutional layers within graph neural networks operate by aggregating information about local neighbourhood structures; one common way to encode such substructures is through random walks. The distribution of these random walks evolves according to a diffusion equation defined using the graph Laplacian. We extend this approach by leveraging classic mathematical results about hypo-elliptic diffusions. This results in a novel tensor-valued graph operator, which we call the hypo-elliptic graph Laplacian. We provide theoretical guarantees and efficient low-rank approximation algorithms. In particular, this gives a structured approach to capture long-range dependencies on graphs that is robust to pooling. Besides the attractive theoretical properties, our experiments show that this method competes with graph transformers on datasets requiring long-range reasoning but scales only linearly in the number of edges as opposed to quadratically in nodes.
更多查看译文
关键词
Graph Diffusion,Random Walks,Hypo-Elliptic Laplacian,Graph Tensor Networks,Graph Classification
AI 理解论文
溯源树
样例
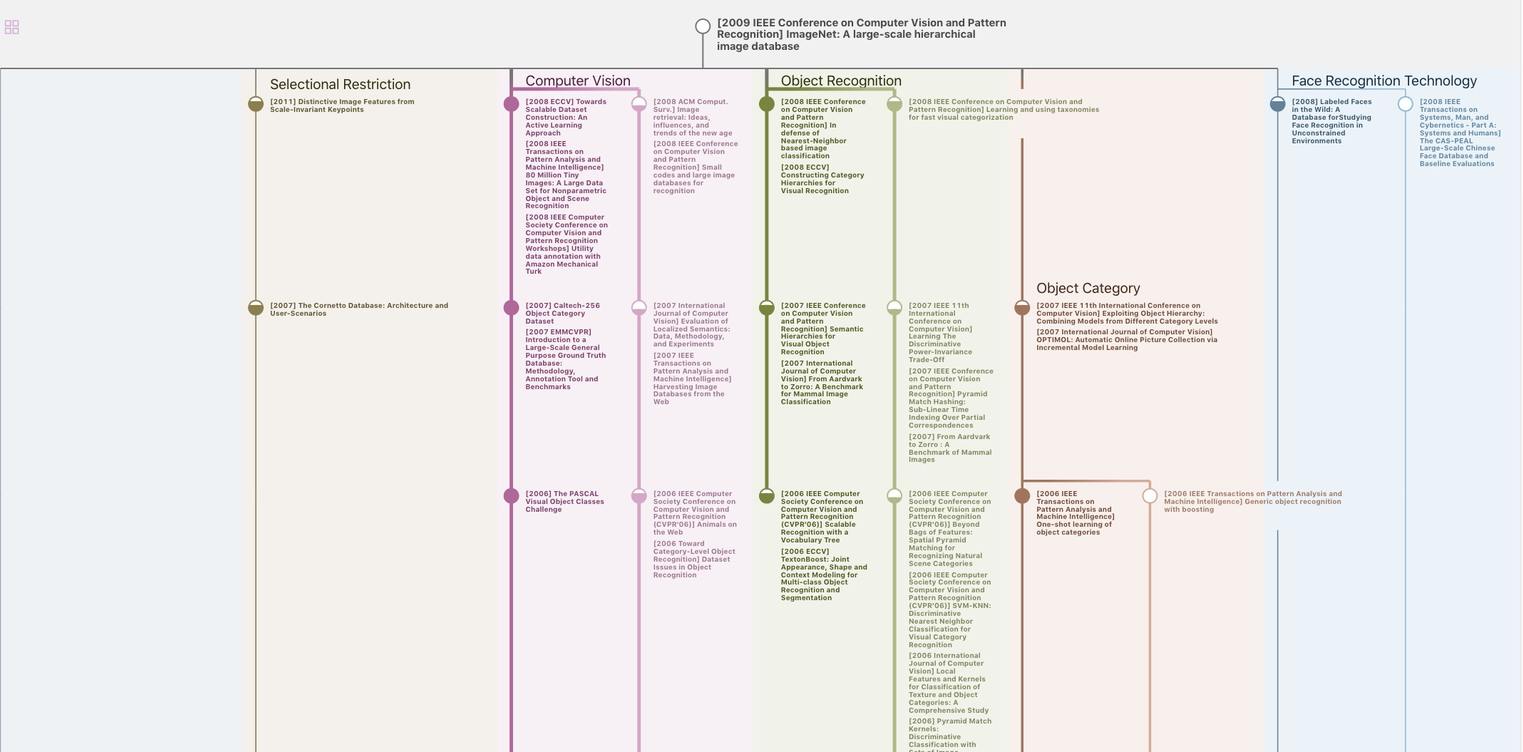
生成溯源树,研究论文发展脉络
Chat Paper
正在生成论文摘要