Learning to Control Linear Systems can be Hard.
Annual Conference on Computational Learning Theory(2022)
摘要
In this paper, we study the statistical difficulty of learning to control linear systems. We focus on two standard benchmarks, the sample complexity of stabilization, and the regret of the online learning of the Linear Quadratic Regulator (LQR). Prior results state that the statistical difficulty for both benchmarks scales polynomially with the system state dimension up to system-theoretic quantities. However, this does not reveal the whole picture. By utilizing minimax lower bounds for both benchmarks, we prove that there exist non-trivial classes of systems for which learning complexity scales dramatically, i.e. exponentially, with the system dimension. This situation arises in the case of underactuated systems, i.e. systems with fewer inputs than states. Such systems are structurally difficult to control and their system theoretic quantities can scale exponentially with the system dimension dominating learning complexity. Under some additional structural assumptions (bounding systems away from uncontrollability), we provide qualitatively matching upper bounds. We prove that learning complexity can be at most exponential with the controllability index of the system, that is the degree of underactuation.
更多查看译文
关键词
control linear systems,learning
AI 理解论文
溯源树
样例
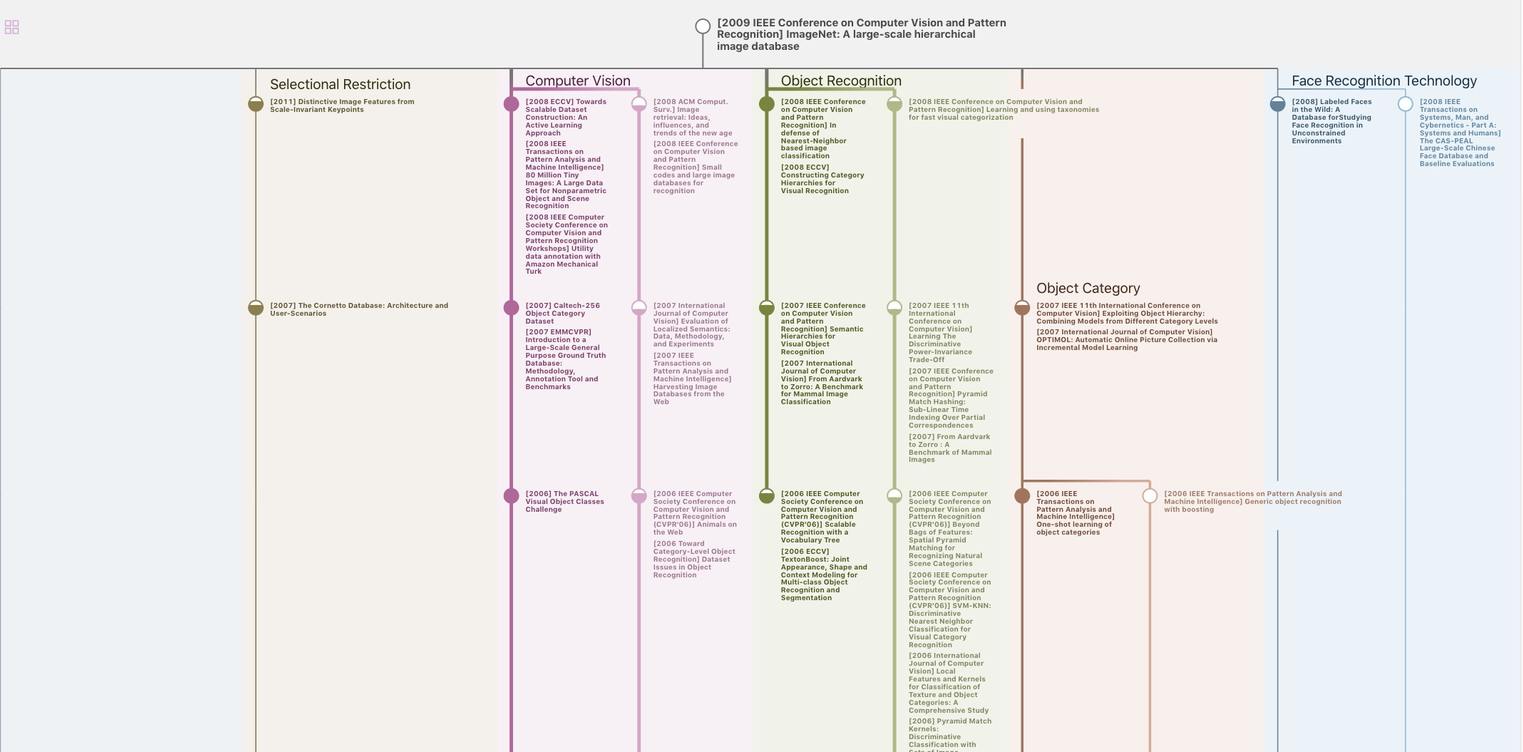
生成溯源树,研究论文发展脉络
Chat Paper
正在生成论文摘要