Demystifying Approximate Value-based RL with $\epsilon$-greedy Exploration: A Differential Inclusion View
arxiv(2023)
摘要
Q-learning and SARSA with $\epsilon$-greedy exploration are leading reinforcement learning methods. Their tabular forms converge to the optimal Q-function under reasonable conditions. However, with function approximation, these methods exhibit strange behaviors such as policy oscillation, chattering, and convergence to different attractors (possibly even the worst policy) on different runs, apart from the usual instability. A theory to explain these phenomena has been a long-standing open problem, even for basic linear function approximation (Sutton, 1999). Our work uses differential inclusion to provide the first framework for resolving this problem. We also provide numerical examples to illustrate our framework's prowess in explaining these algorithms' behaviors.
更多查看译文
关键词
differential inclusion,epsilon-greedy exploration,function approximation,value-based RL,Q-learning,SARSA,policy oscillation,chattering,discontinuous policies,stability
AI 理解论文
溯源树
样例
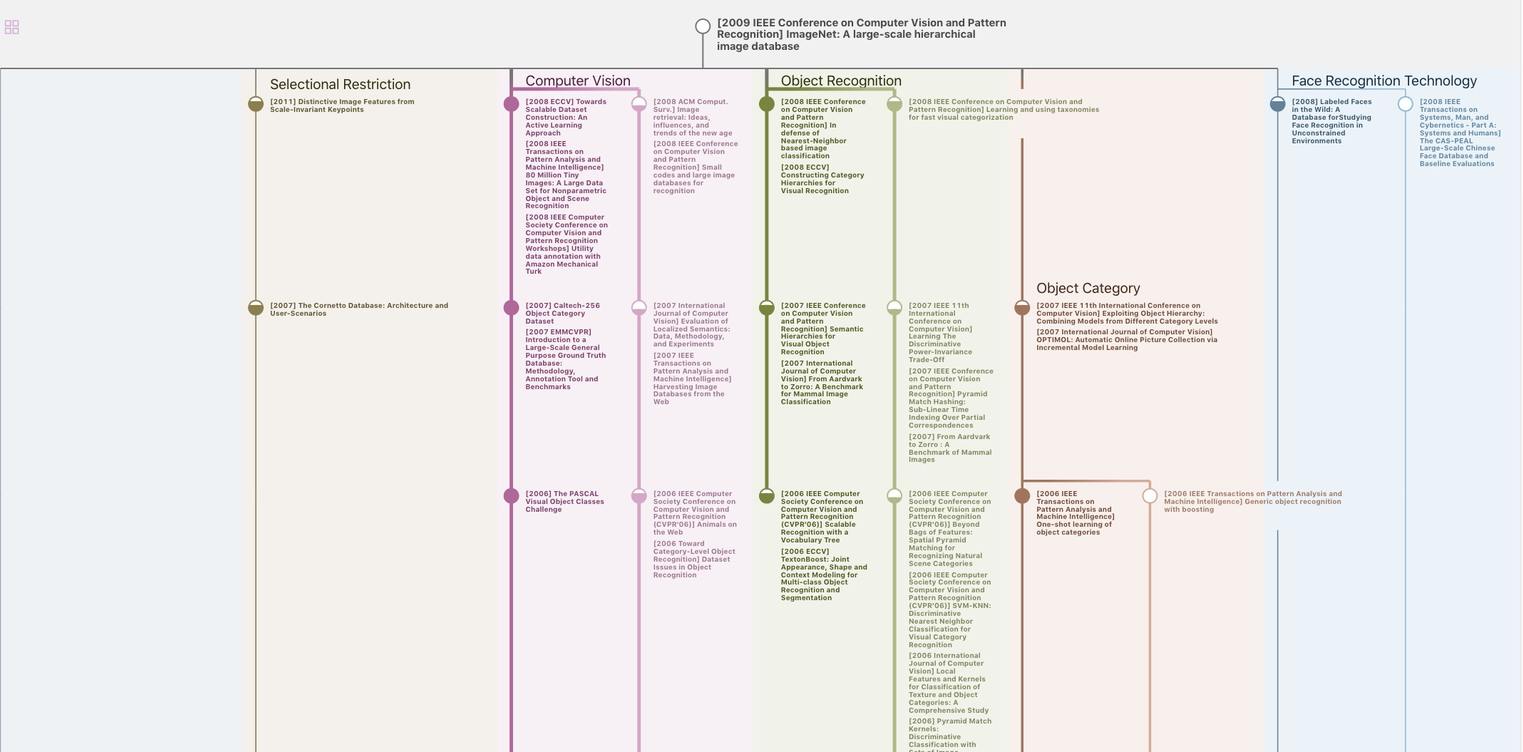
生成溯源树,研究论文发展脉络
Chat Paper
正在生成论文摘要