A LUNG-PARENCHYMA-CONTRAST HYBRID NETWORK FOR EGFR GENE MUTATION PREDICTION IN LUNG CANCER
2022 IEEE INTERNATIONAL SYMPOSIUM ON BIOMEDICAL IMAGING (IEEE ISBI 2022)(2022)
摘要
Epidermal growth factor receptor (EGFR) mutation status is critical for lung cancer treatment planning. Current identification relies on invasive biopsy and expensive gene sequencing. Recent studies revealed that CT images combined with deep learning can be used to non-invasively predict EGFR mutation status. However, how to enable the network to focus on the lung parenchyma area and extract discriminative features needs further exploration. In this study, we proposed a lung-parenchyma-contrast (LPC) hybrid network that: 1) uses a fully automatic whole-lung analysis method and enables the model to focus on the lung parenchyma area; 2) extracts local and global lung parenchyma features by a contrastive learning strategy; and 3) jointly performs feature learning and classifier learning to improve predictive performance. We evaluated our network on a large multi-center dataset (2316 patients), which outperforms (AUC=0.827) the previous state-of-the-art methods. Extensive experiments also demonstrated the effectiveness of the contrastive learning modules.
更多查看译文
关键词
EGFR gene mutation, targeted therapy, lung cancer, contrastive learning, computed tomography
AI 理解论文
溯源树
样例
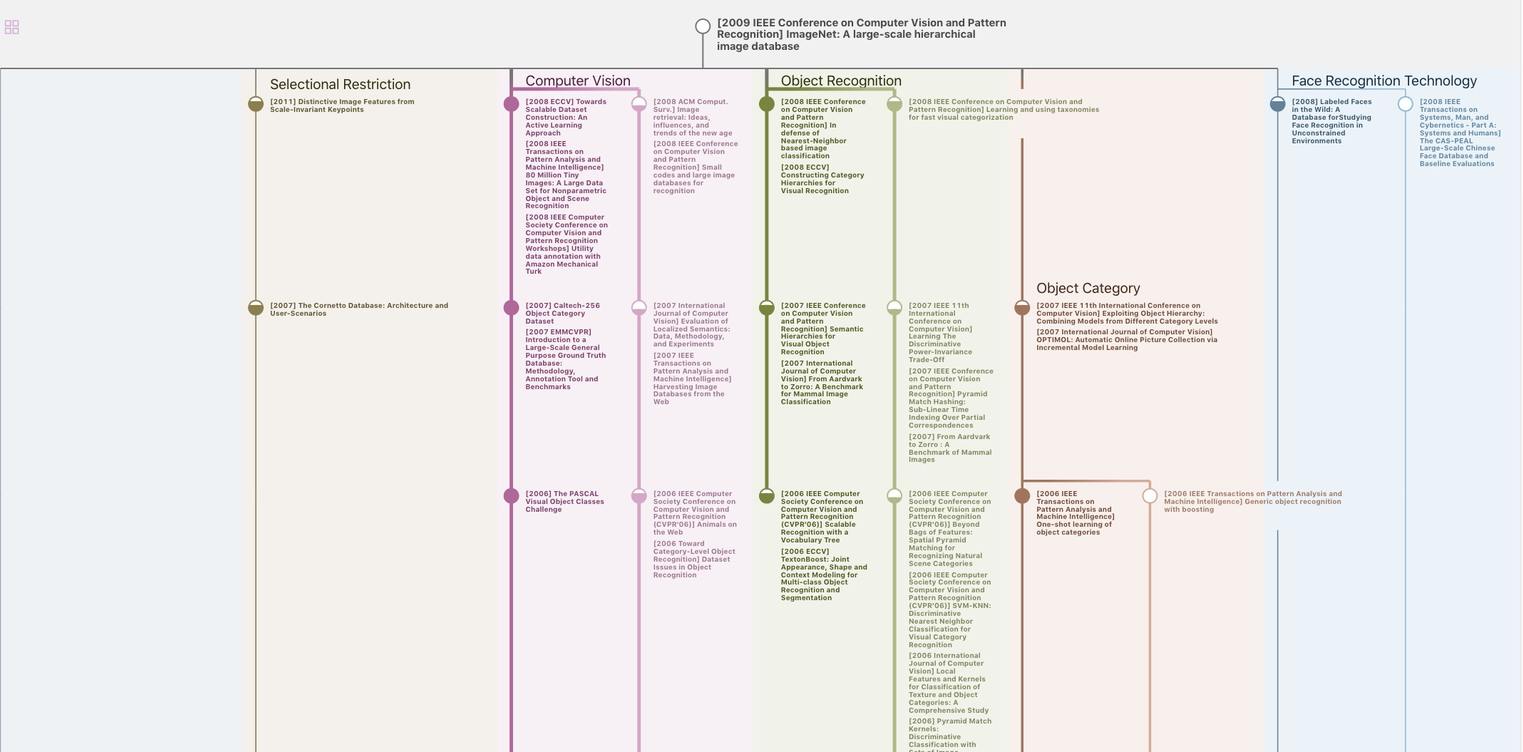
生成溯源树,研究论文发展脉络
Chat Paper
正在生成论文摘要