Dynamic Auxiliary Task-Based Evolutionary Multitasking for Constrained Multiobjective Optimization
user-61447a76e55422cecdaf7d19(2023)
摘要
When solving constrained multiobjective optimization problems (CMOPs), the utilization of infeasible solutions significantly affects algorithm’s performance because they not only maintain diversity but also provide promising search directions. In light of this situation, this article proposes a new multitasking-constrained multiobjective optimization (MTCMO) framework, in which a dynamic auxiliary task is created to assist in solving a complex CMOP (the main task) via the knowledge transfer. Moreover, the constraint boundary of the auxiliary task reduces dynamically, so that it keeps a high relatedness with the main task to continuously provide supplementary evolutionary directions. Furthermore, an improved
$\epsilon $
method is designed for the auxiliary task to utilize diverse high-quality infeasible solutions for breaking through infeasible obstacles in the early stage and approaching the feasible boundary from infeasible regions in the later stage. Besides, a new test function with decision space constraints is designed, where one parameter can be adjusted to control the overlap degree between the constrained Pareto front and the unconstrained Pareto front. This function and the other two modified existing functions are used to analyze the characteristics of MTCMO. Finally, compared with 11 state-of-the-art peer methods, the superior or competitive performance of MTCMO is demonstrated on 54 benchmark functions and two real-world applications.
更多查看译文
关键词
Task analysis, Optimization, Statistics, Sociology, Multitasking, Search problems, Convergence, Auxiliary task, constrained multiobjective optimization, evolutionary multitasking (EMT), improved epsilon method, knowledge transfer
AI 理解论文
溯源树
样例
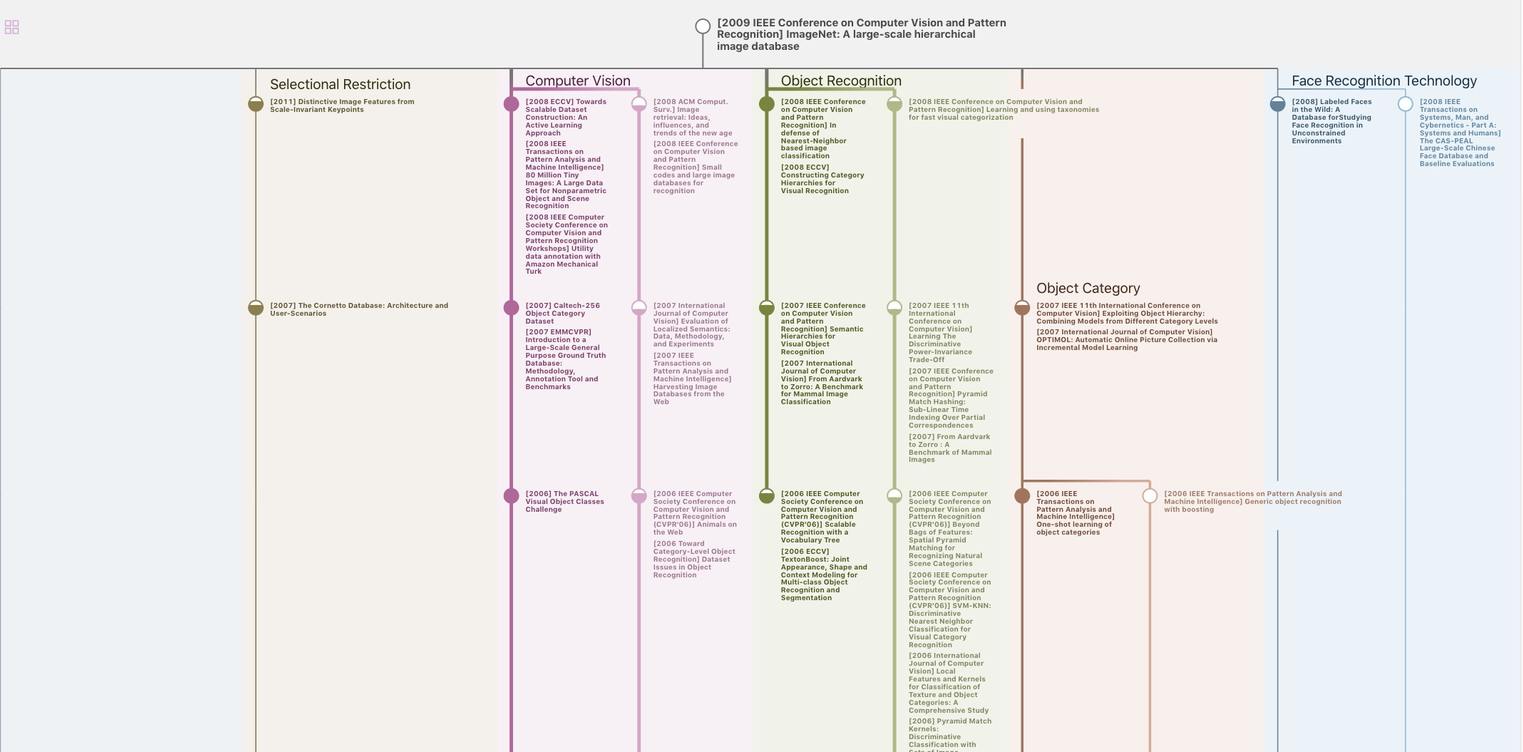
生成溯源树,研究论文发展脉络
Chat Paper
正在生成论文摘要