Multicategory damage detection and safety assessment of post-earthquake reinforced concrete structures using deep learning
COMPUTER-AIDED CIVIL AND INFRASTRUCTURE ENGINEERING(2022)
摘要
Earthquake damage investigation is critical to post-earthquake structural recovery and reconstruction. In this study, a method of assessing the component failure mode and damage level was established based on object detection and recognition. A quantitative structural damage level assessment method was developed based on the type and extent of damage to the components. A You Only Look Once v4 (YOLOv4) network was used to detect multicategory damage (fine crack, wide crack, concrete spalling, exposed rebar and buckled rebar). Depthwise separable convolution was introduced into YOLOv4 to decrease the computation cost without reducing accuracy. Finally, the damage detection method and assessment method were integrated within a graphical user interface (GUI) to facilitate the post-earthquake reinforced concrete (RC) structural damage assessment. The test results by GUI indicate that the improved object network can get accurate detection results, and the preliminary safety assessment method can judge the damage level and failure mode. The present study shows high potential for estimating the seismic damage states of RC structures.
更多查看译文
AI 理解论文
溯源树
样例
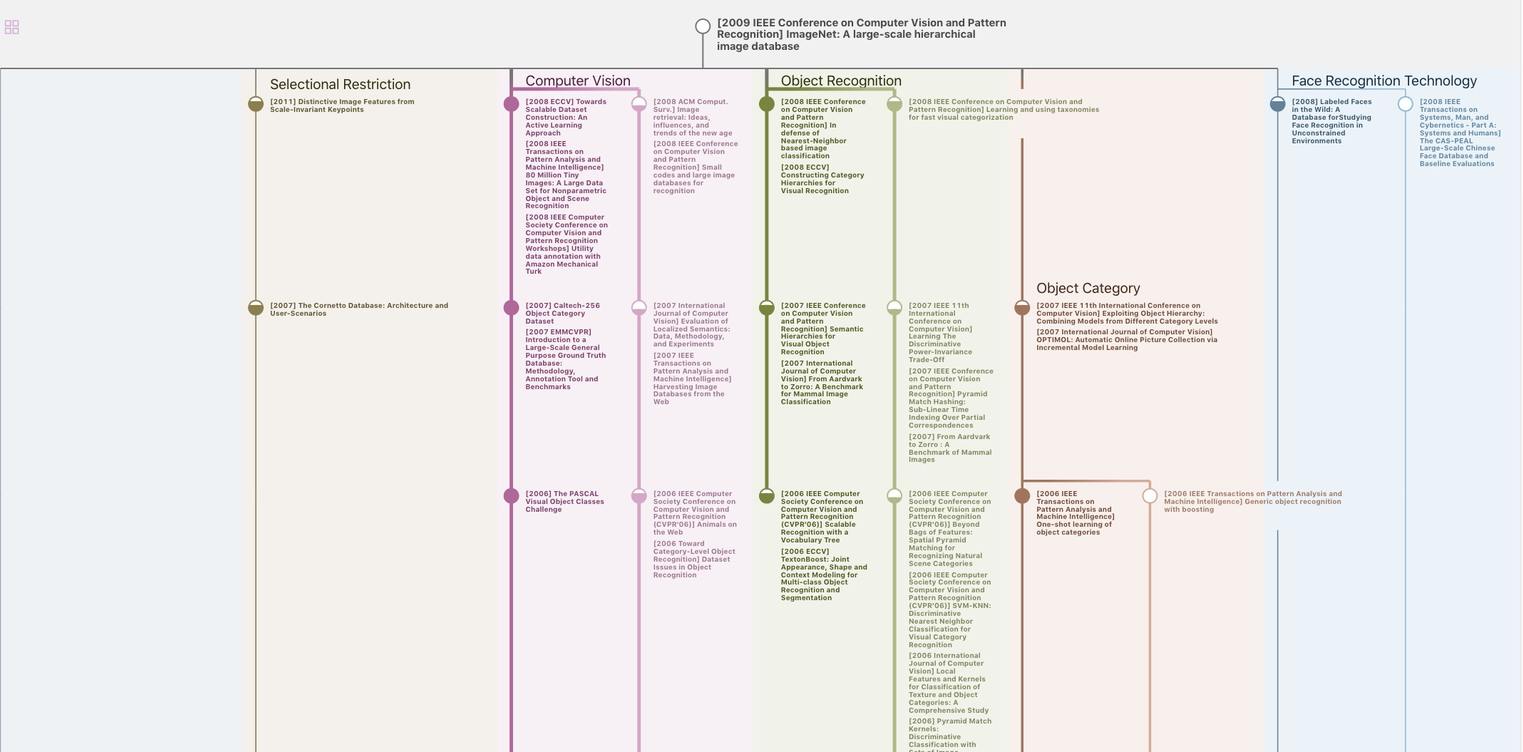
生成溯源树,研究论文发展脉络
Chat Paper
正在生成论文摘要