Unsupervised Learning-Driven Matheuristic for Production-Distribution Problems
user-61447a76e55422cecdaf7d19(2022)
摘要
In this paper, we study a capacitated production-distribution problem where facility location, production, and distribution decisions are tightly coupled and simultaneously considered in the optimal decision making. Such an integrated production-distribution problem is complicated, and the current commercial mixed-integer linear programming (MILP) solvers cannot obtain favorable solutions for the medium- and large-sized problem instances. Therefore, we propose an unsupervised learning-driven matheuristic that uses easily obtainable solution values (e.g., solutions associated with the linear programming relaxation) to build clustering models and integrates the clustering information with a genetic algorithm to progressively improve feasible solutions. Then we verify the performance of the proposed matheuristic by comparing its computational results with those of the rolling horizon algorithm, a non-cluster-driven matheuristic, and a commercial MILP solver. The computational results show that, under the same computing resources, the proposed matheuristic can deliver better production-distribution decisions. Specifically, it reduces the total system costs by 15% for the tested instances when compared with the ones found by the commercial MILP solver. Additionally, we apply the proposed matheuristic to a related production-distribution problem in the literature and obtain 152 equivalent or new best-known solutions out of 200 benchmark test instances.
更多查看译文
关键词
production-distribution, production planning, lot sizing, facility location, unsupervised learning, cluster analysis, genetic algorithms, machine learning
AI 理解论文
溯源树
样例
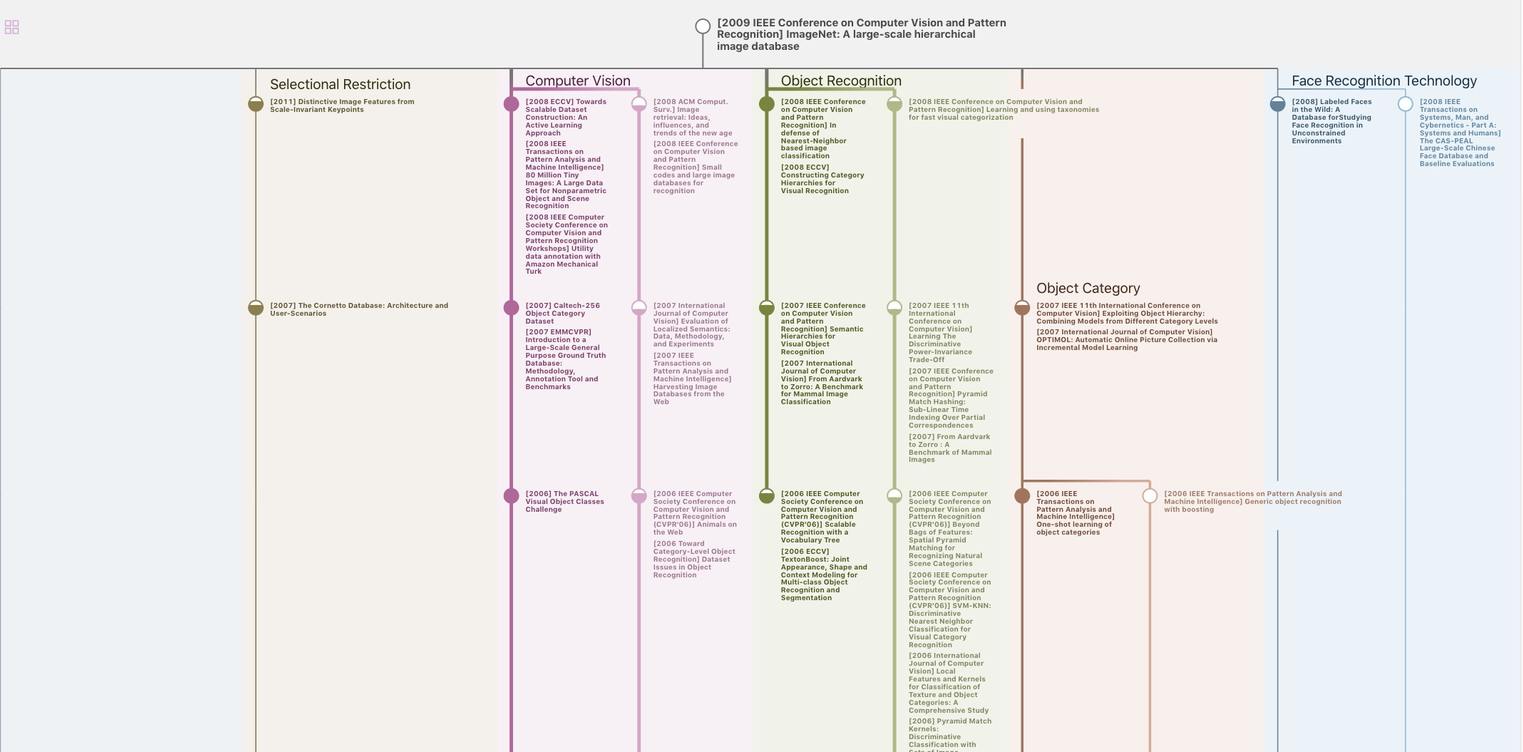
生成溯源树,研究论文发展脉络
Chat Paper
正在生成论文摘要