A New Graph-Based Fractality Index to Characterize Complexity of Urban Form
ISPRS INTERNATIONAL JOURNAL OF GEO-INFORMATION(2022)
摘要
Examining the complexity of urban form may help to understand human behavior in urban spaces, thereby improving the conditions for sustainable design of future cities. Metrics, such as fractal dimension, ht-index, and cumulative rate of growth (CRG) index have been proposed to measure this complexity. However, as these indicators are statistical rather than spatial, they result in an inability to characterize the spatial complexity of urban forms, such as building footprints. To overcome this problem, this paper proposes a graph-based fractality index (GFI), which is based on a hybrid of fractal theory and deep learning techniques. First, to quantify the spatial complexity, several fractal variants were synthesized to train a deep graph convolutional neural network. Next, building footprints in London were used to test the method, where the results showed that the proposed framework performed better than the traditional indices, i.e., the index is capable of differentiating complex patterns. Another advantage is that it seems to assure that the trained deep learning is objective and not affected by potential biases in empirically selected training datasets Furthermore, the possibility to connect fractal theory and deep learning techniques on complexity issues opens up new possibilities for data-driven GIS science.
更多查看译文
关键词
complexity, fractals, building groups, graph convolutional neural networks, urban form
AI 理解论文
溯源树
样例
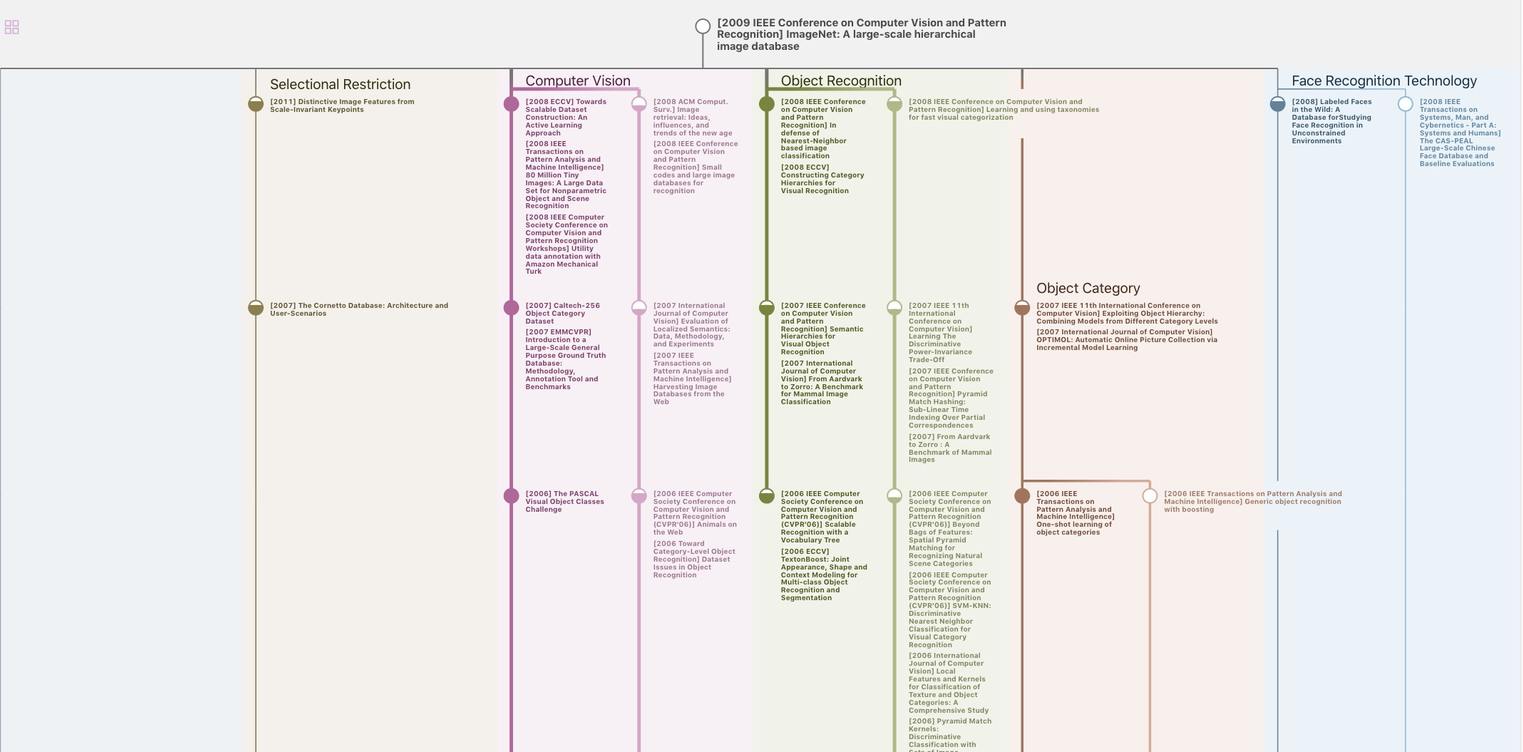
生成溯源树,研究论文发展脉络
Chat Paper
正在生成论文摘要