Category-Adapted Sound Event Enhancement with Weakly Labeled Data
IEEE International Conference on Acoustics, Speech, and Signal Processing (ICASSP)(2022)
摘要
Previous audio enhancement training usually requires clean signals with additive noises; hence commonly focuses on speech enhancement, where clean speech is easy to access. This paper goes beyond a broader sound event enhancement by using a weakly supervised approach via sound event detection (SED) to approximate the location and presence of a specific sound event. We propose a category-adapted system to enable enhancement on any selected sound category, where we first familiarize the model to all common sound classes and followed by a category-specific fine-tune procedure to enhance the targeted sound class. Evaluation is conducted on ten common sound classes, with a comparison to traditional and weakly supervised enhancement methods. Results indicate an average 2.86 dB SDR increase, with more significant improvement on speech (9.15 dB), music (5.01 dB), and typewriter (3.68 dB) under SNR of 0 dB. All enhancement metrics outperform previous weakly supervised methods and achieve comparable results to the state-of-the-art method that requires clean signals.
更多查看译文
关键词
source separation,weakly supervised learning,deep neural networks,category adaptation
AI 理解论文
溯源树
样例
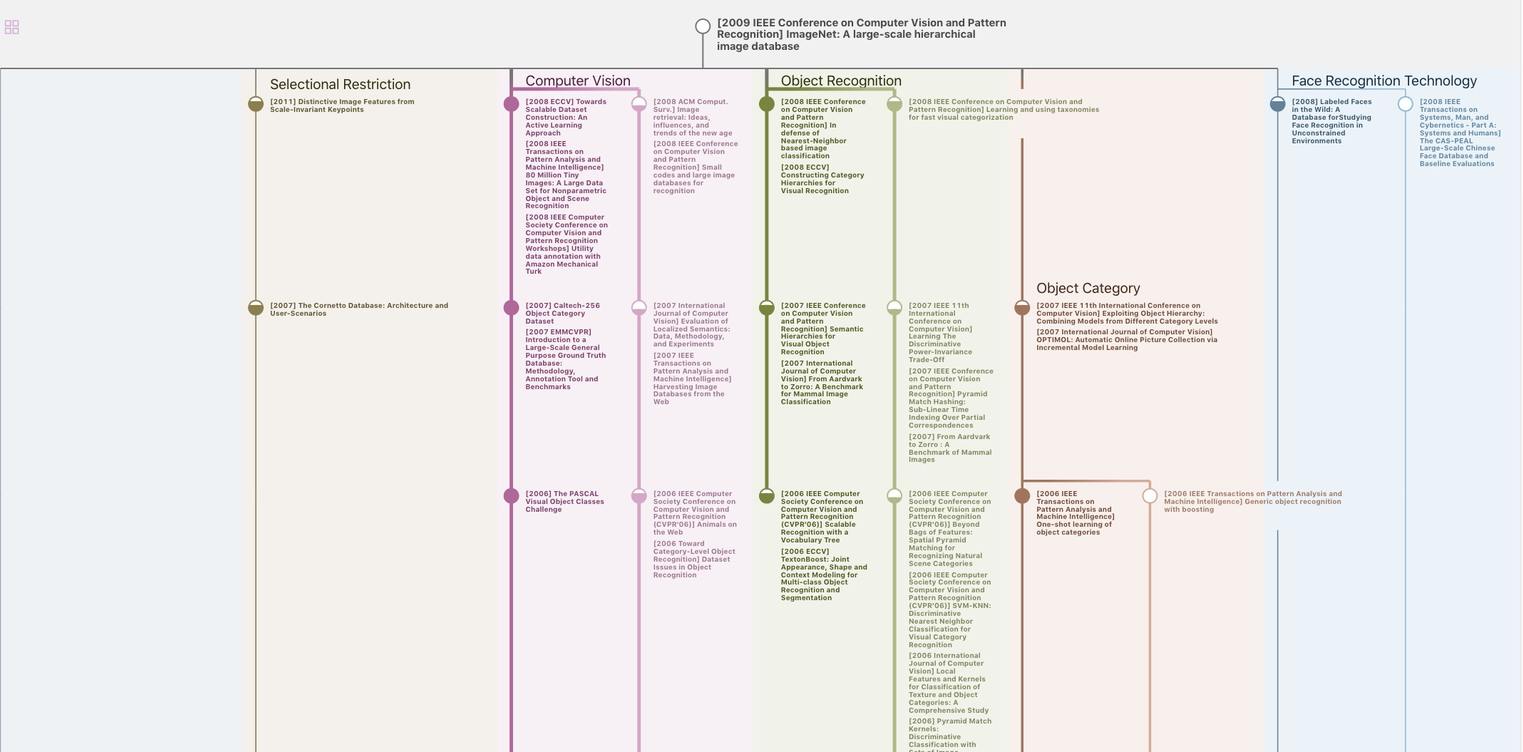
生成溯源树,研究论文发展脉络
Chat Paper
正在生成论文摘要