Scheduling to Optimize Sojourn Time of Successful Jobs
arxiv(2022)
摘要
Deep neural networks training jobs and other iterative computations frequently include checkpoints where jobs can be canceled based on the current value of monitored metrics. While most of existing results focus on the performance of all jobs (both successfully completed and canceled), in this work we explore scheduling policies that improve the sojourn time of successful jobs, which are typically more valuable to the user. Our model assumes that each job has a known discrete size distribution (e.g., estimated from previous execution logs) where the largest size value indicates a successful completion, while other size values correspond to termination checkpoints. In the single-server case where all jobs are available for scheduling simultaneously, we prove that optimal schedules do not preempt jobs, even when preemption overhead is negligible. Based on this, we develop a scheduling policy that minimizes the sojourn time of successful jobs asymptotically, i.e., when the number of jobs grows to infinity. Through an extensive numerical study, we show that this policy performs better than existing alternatives even when the number of jobs is finite. For more realistic scenarios with multiple servers and dynamic jobs arrivals, we propose an online approach based on our single-server scheduling policy. Through an extensive simulation study, using real-world traces, we demonstrate that this online approach results in better average sojourn time for successful jobs as compared to existing techniques.
更多查看译文
关键词
optimize sojourn time,scheduling,successful jobs
AI 理解论文
溯源树
样例
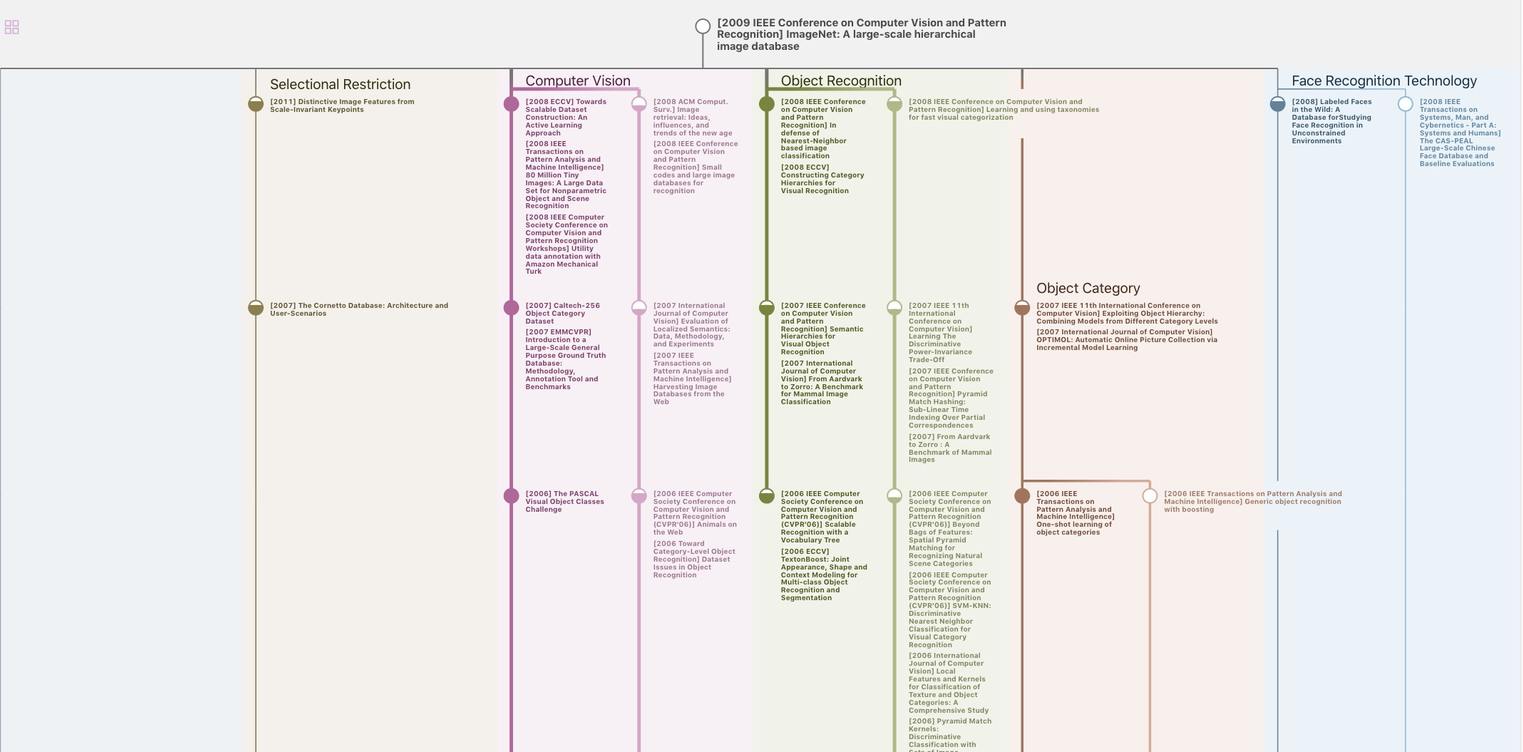
生成溯源树,研究论文发展脉络
Chat Paper
正在生成论文摘要