Maximum Mean Discrepancy on Exponential Windows for Online Change Detection
arxiv(2023)
摘要
Detecting changes is of fundamental importance when analyzing data streams and has many applications, e.g., predictive maintenance, fraud detection, or medicine. A principled approach to detect changes is to compare the distributions of observations within the stream to each other via hypothesis testing. Maximum mean discrepancy (MMD; also called energy distance) is a well-known (semi-)metric on the space of probability distributions. MMD gives rise to powerful non-parametric two-sample tests on kernel-enriched domains under mild conditions, which makes its deployment for change detection desirable. However, the classic MMD estimators suffer quadratic complexity, which prohibits their application in the online change detection setting. We propose a general-purpose change detection algorithm, Maximum Mean Discrepancy on Exponential Windows (MMDEW), which leverages the MMD two-sample test, facilitates its efficient online computation on any kernel-enriched domain, and is able to detect any disparity between distributions. Our experiments and analysis show that (1) MMDEW achieves better detection quality than state-of-the-art competitors and that (2) the algorithm has polylogarithmic runtime and logarithmic memory requirements, which allow its deployment to the streaming setting.
更多查看译文
AI 理解论文
溯源树
样例
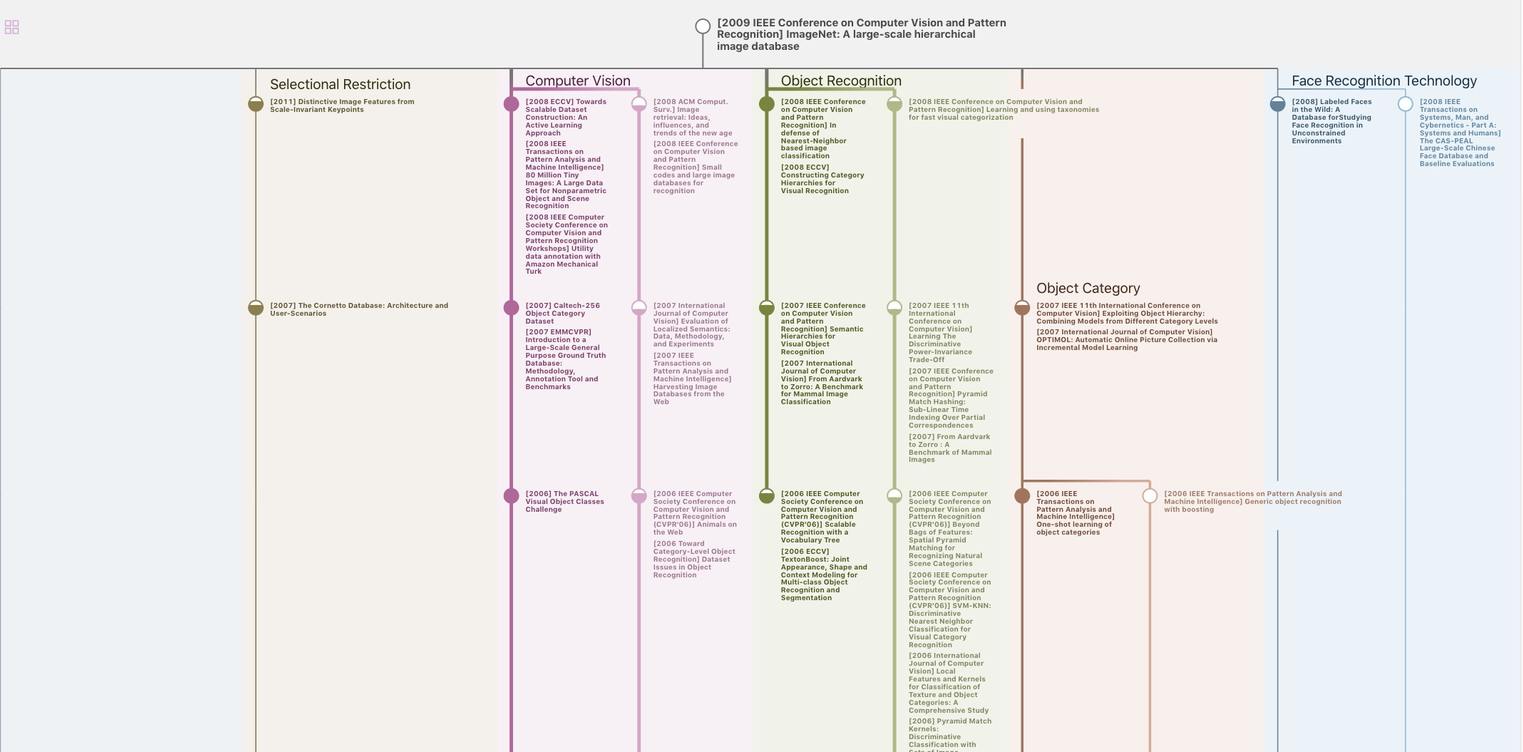
生成溯源树,研究论文发展脉络
Chat Paper
正在生成论文摘要