Fast & Furious: On the modelling of malware detection as an evolving data stream
Expert Systems with Applications(2023)
摘要
Malware is a major threat to computer systems and imposes many challenges to cyber security. Targeted threats, such as ransomware, cause millions of dollars in losses every year. The constant increase of malware infections has been motivating popular antiviruses (AVs) to develop dedicated detection strategies, which include meticulously crafted machine learning (ML) pipelines. However, malware developers unceasingly change their samples’ features to bypass detection. This constant evolution of malware samples causes changes to the data distribution (i.e., concept drifts) that directly affect ML model detection rates, something not considered in the majority of the literature work. In this work, we evaluate the impact of concept drift on malware classifiers for two Android datasets: DREBIN (≈130K apps) and a subset of AndroZoo (≈285K apps). We used these datasets to train an Adaptive Random Forest (ARF) classifier, as well as a Stochastic Gradient Descent (SGD) classifier. We also ordered all datasets samples using their VirusTotal submission timestamp and then extracted features from their textual attributes using two algorithms (Word2Vec and TF-IDF). Then, we conducted experiments comparing both feature extractors, classifiers, as well as four drift detectors (Drift Detection Method, Early Drift Detection Method, ADaptive WINdowing, and Kolmogorov–Smirnov WINdowing) to determine the best approach for real environments. Finally, we compare some possible approaches to mitigate concept drift and propose a novel data stream pipeline that updates both the classifier and the feature extractor. To do so, we conducted a longitudinal evaluation by (i) classifying malware samples collected over nine years (2009–2018), (ii) reviewing concept drift detection algorithms to attest its pervasiveness, (iii) comparing distinct ML approaches to mitigate the issue, and (iv) proposing an ML data stream pipeline that outperformed literature approaches, achieving an improvement of 22.05 percentage points of F1Score in the DREBIN dataset, and 8.77 in the AndroZoo dataset.
更多查看译文
关键词
Machine learning,Data streams,Concept drift,Malware detection,Android
AI 理解论文
溯源树
样例
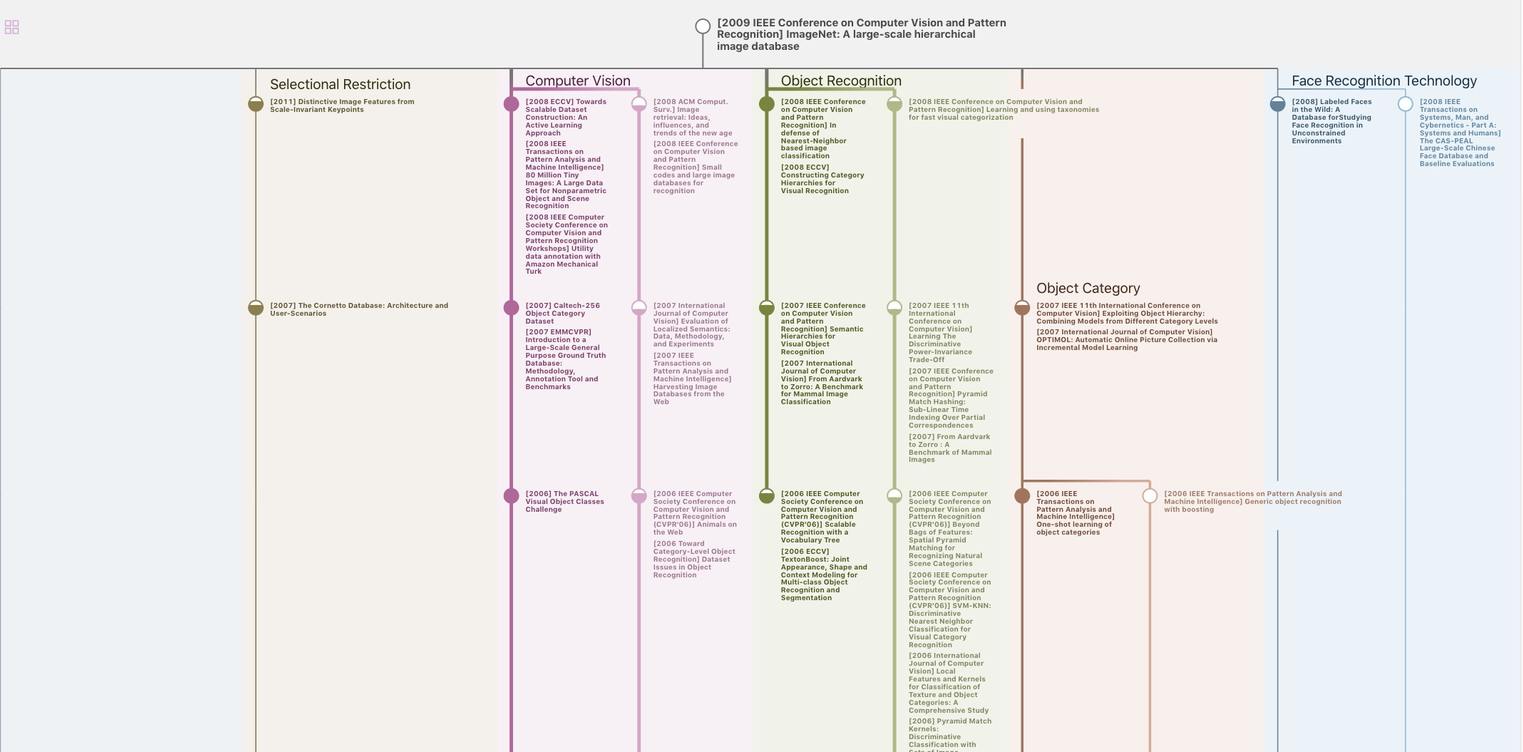
生成溯源树,研究论文发展脉络
Chat Paper
正在生成论文摘要