Learning interacting dynamical systems with latent Gaussian process ODEs.
NeurIPS 2022(2022)
摘要
We study time uncertainty-aware modeling of continuous-time dynamics of interacting objects. We introduce a new model that decomposes independent dynamics of single objects accurately from their interactions. By employing latent Gaussian process ordinary differential equations, our model infers both independent dynamics and their interactions with reliable uncertainty estimates. In our formulation, each object is represented as a graph node and interactions are modeled by accumulating the messages coming from neighboring objects. We show that efficient inference of such a complex network of variables is possible with modern variational sparse Gaussian process inference techniques. We empirically demonstrate that our model improves the reliability of long-term predictions over neural network based alternatives and it successfully handles missing dynamic or static information. Furthermore, we observe that only our model can successfully encapsulate independent dynamics and interaction information in distinct functions and show the benefit from this disentanglement in extrapolation scenarios.
更多查看译文
关键词
Gaussian Processes,Ordinary Differential Equations,Interacting Dynamical Systems
AI 理解论文
溯源树
样例
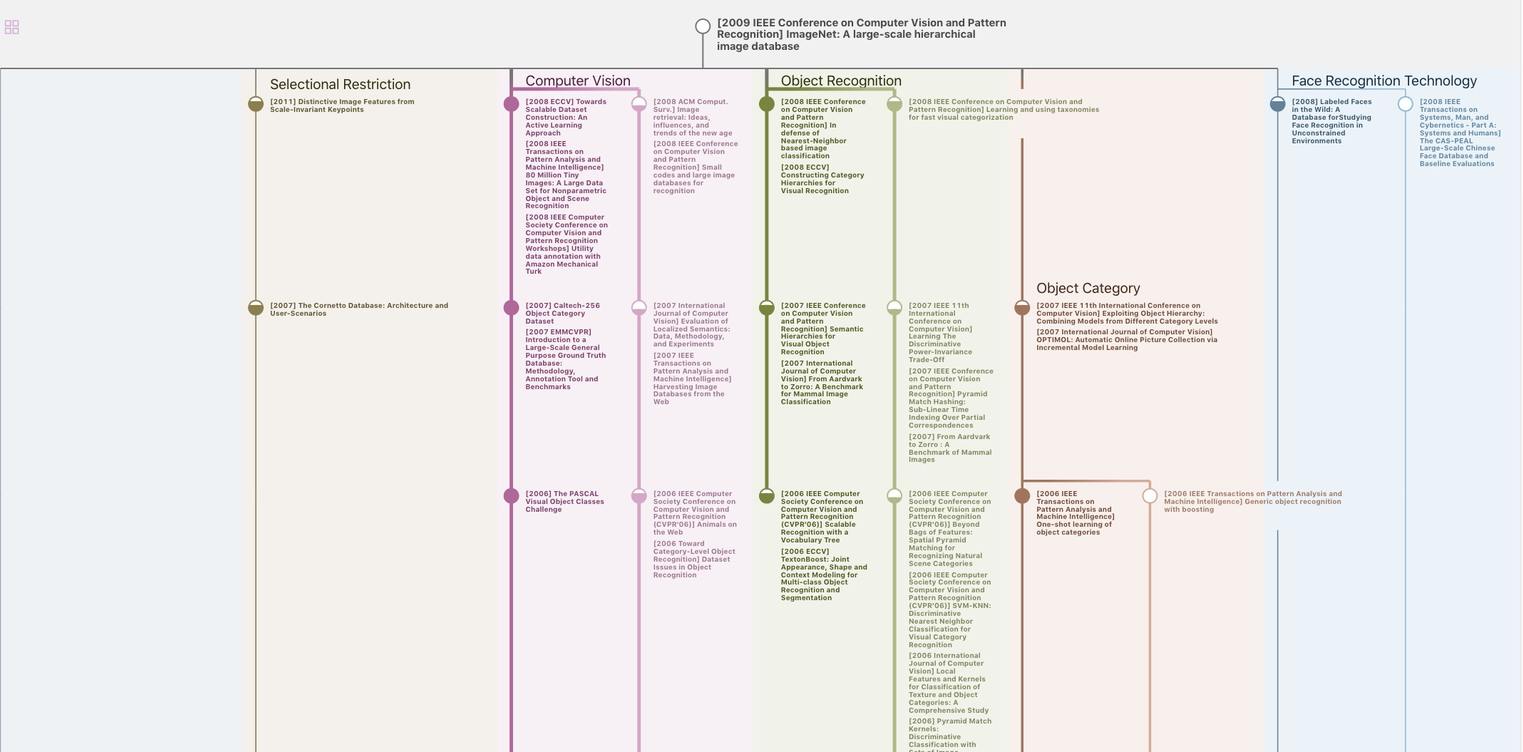
生成溯源树,研究论文发展脉络
Chat Paper
正在生成论文摘要