Embedding Neighborhoods Simultaneously t-SNE (ENS-t-SNE)
arxiv(2022)
摘要
We propose an algorithm for visualizing a dataset by embedding it in 3-dimensional Euclidean space based on various given distances between the same pairs of datapoints. Its aim is to find an Embedding which preserves Neighborhoods Simultaneously for all given distances by generalizing the t-Stochastic Neighborhood Embedding approach (ENS-t-SNE). We illustrate the utility of ENS-t-SNE by demonstrating its use in three applications. First, to visualize different notions of clusters and groups within the same high-dimensional dataset with one 3-dimensional embedding, as opposed to providing different embeddings of the same data and trying to match the corresponding points. Second, to illustrate the effects of different hyper-parameters of the classical t-SNE. Third, by considering multiple different notions of clustering in data, ENS-t-SNE can generate an alternative embedding than the classic t-SNE. We provide an extensive quantitative evaluation with real-world and synthetic datasets of different sizes and using different numbers of projections.
更多查看译文
关键词
neighborhoods,ens-t-sne
AI 理解论文
溯源树
样例
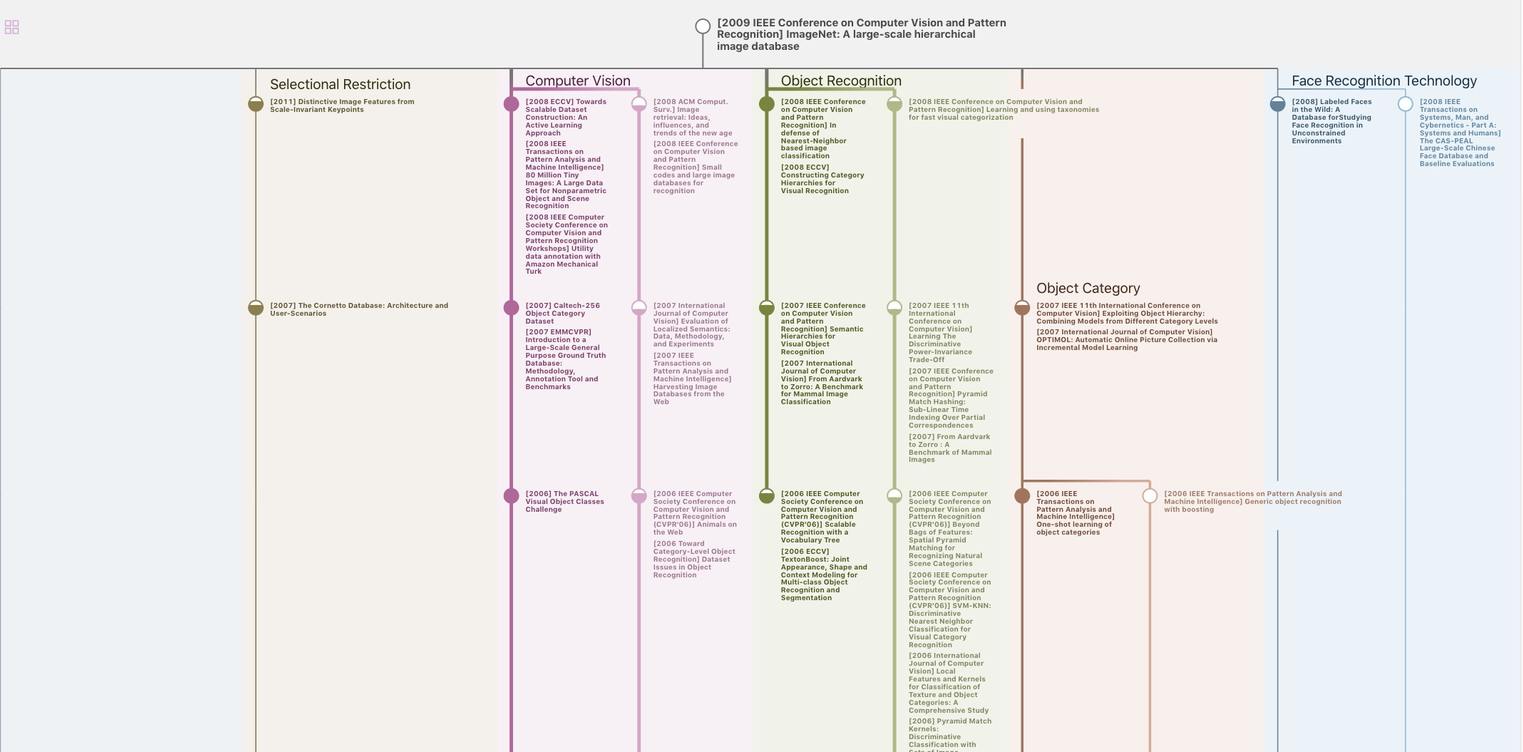
生成溯源树,研究论文发展脉络
Chat Paper
正在生成论文摘要