Deep Representations for Time-varying Brain Datasets
KDD '22: Proceedings of the 28th ACM SIGKDD Conference on Knowledge Discovery and Data Mining(2022)
摘要
Finding an appropriate representation of dynamic activities in the brain is crucial for many downstream applications. Due to its highly dynamic nature, temporally averaged fMRI (functional magnetic resonance imaging) can only provide a narrow view of underlying brain activities. Previous works lack the ability to learn and interpret the latent dynamics in brain architectures. This paper builds an efficient graph neural network model that incorporates both region-mapped fMRI sequences and structural connectivities obtained from DWI (diffusion-weighted imaging) as inputs. We find good representations of the latent brain dynamics through learning sample-level adaptive adjacency matrices and performing a novel multi-resolution inner cluster smoothing. We also attribute inputs with integrated gradients, which enables us to infer (1) highly involved brain connections and subnetworks for each task, (2) temporal keyframes of imaging sequences that characterize tasks, and (3) subnetworks that discriminate between individual subjects. This ability to identify critical subnetworks that characterize signal states across heterogeneous tasks and individuals is of great importance to neuroscience and other scientific domains. Extensive experiments and ablation studies demonstrate our proposed method's superiority and efficiency in spatial-temporal graph signal modeling with insightful interpretations of brain dynamics.
更多查看译文
关键词
deep representations,brain,time-varying
AI 理解论文
溯源树
样例
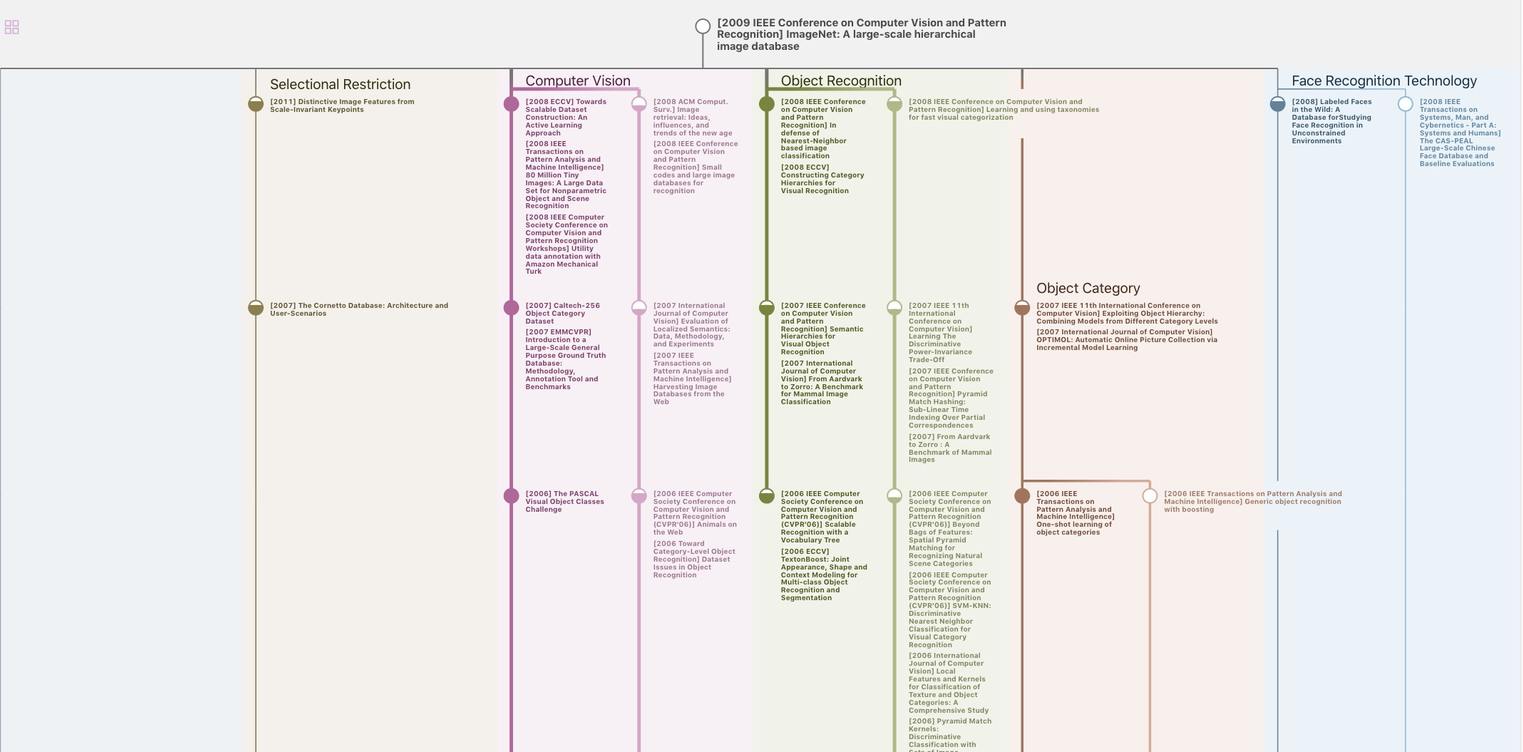
生成溯源树,研究论文发展脉络
Chat Paper
正在生成论文摘要