THE FEDERATED TUMOR SEGMENTATION (FETS) INITIATIVE: THE FIRST REAL-WORLD LARGE-SCALE DATA-PRIVATE COLLABORATION FOCUSING ON NEURO-ONCOLOGY
NEURO-ONCOLOGY(2021)
摘要
Abstract PURPOSE Robustness and generalizability of artificial intelligent (AI) methods is reliant on the training data size and diversity, which are currently hindered in multi-institutional healthcare collaborations by data ownership and legal concerns. To address these, we introduce the Federated Tumor Segmentation (FeTS) Initiative, as an international consortium using federated learning (FL) for data-private multi-institutional collaborations, where AI models leverage data at participating institutions, without sharing data between them. The initial FeTS use-case focused on detecting brain tumor boundaries in MRI. METHODS The FeTS tool incorporates: 1) MRI pre-processing, including image registration and brain extraction; 2) automatic delineation of tumor sub-regions, by label fusion of pretrained top-performing BraTS methods; 3) tools for manual delineation refinements; 4) model training. 55 international institutions identified local retrospective cohorts of glioblastoma patients. Ground truth was generated using the first 3 FeTS functionality modes as mentioned earlier. Finally, the FL training mode comprises of i) an AI model trained on local data, ii) local model updates shared with an aggregator, which iii) combines updates from all collaborators to generate a consensus model, and iv) circulates the consensus model back to all collaborators for iterative performance improvements. RESULTS The first FeTS consensus model, from 23 institutions with data of 2,200 patients, showed an average improvement of 11.1% in the performance of the model on each collaborator’s validation data, when compared to a model trained on the publicly available BraTS data (n=231). CONCLUSION Our findings support that data increase alone would lead to AI performance improvements without any algorithmic development, hence indicating that the model performance would improve further when trained with all 55 collaborating institutions. FL enables AI model training with knowledge from data of geographically-distinct collaborators, without ever having to share any data, hence overcoming hurdles relating to legal, ownership, and technical concerns of data sharing.
更多查看译文
AI 理解论文
溯源树
样例
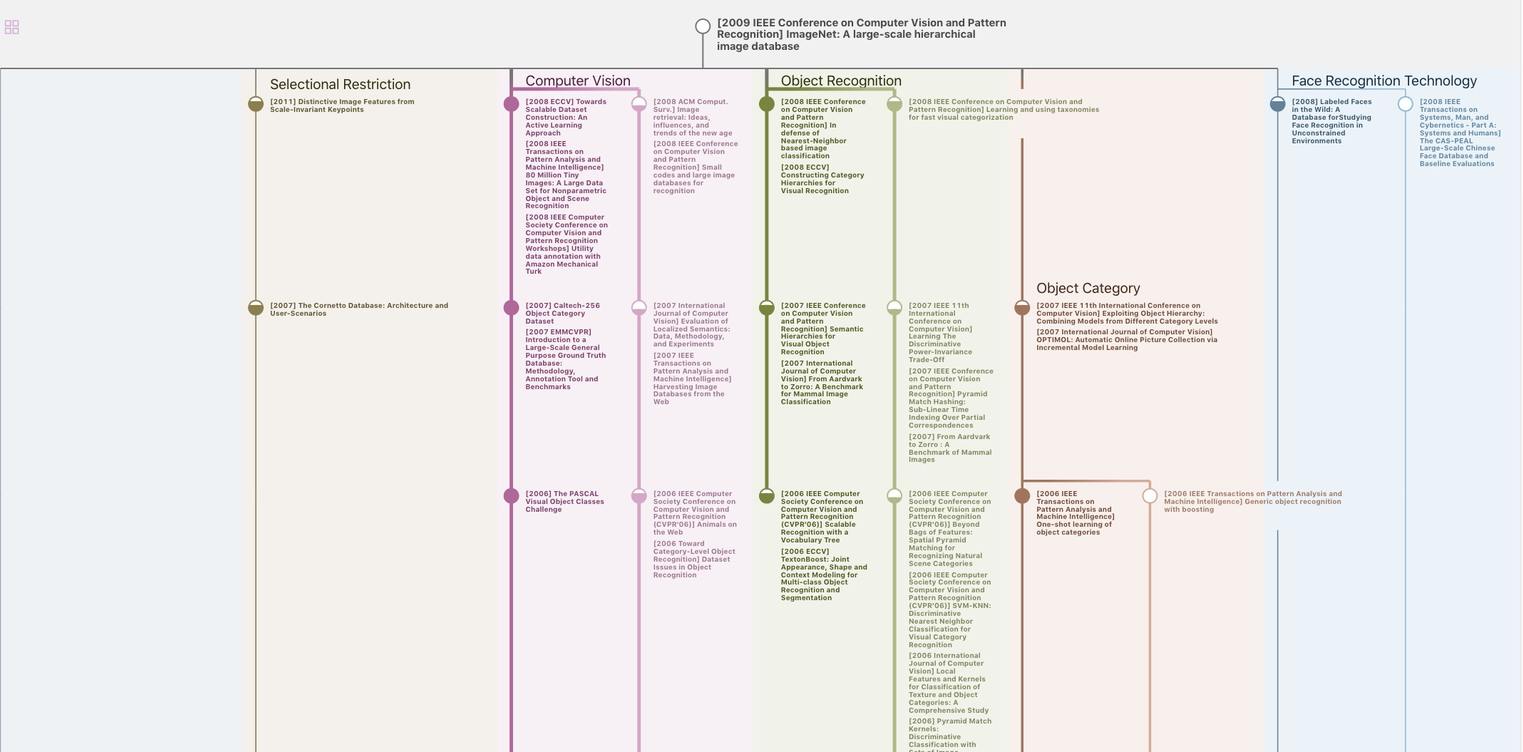
生成溯源树,研究论文发展脉络
Chat Paper
正在生成论文摘要