A Dynamic Scheduling Framework for Byproduct Gas System Combining Expert Knowledge and Production Plan
IEEE TRANSACTIONS ON AUTOMATION SCIENCE AND ENGINEERING(2023)
摘要
Effective scheduling for byproduct gas systems of steel industry is becoming increasingly vital for maintaining their safe operating and improving energy utilization. Considering that the existing studies failed to capture the dynamic changes in the production environment, a novel dynamic scheduling framework is proposed that seamingly integrates expert knowledge with a dynamic programming process. Given the phase characteristics of the steelmaking processes, data series are first partitioned into information granules based on the production plan to form the knowledge-based initial policies. To achieve dynamic scheduling process, a two-stage value function approximation method is proposed, where in the first stage one learns an event-driven Q-function by the fuzzy rule-based states, and then an action fitting strategy is developed for evaluating continuous actions. Considering the difficulties of establishing a mechanism-based model, the state transition process is described by a granular prediction model to simulate taking actions. On their basis, a dynamic compensation for the initial policies is finally achieved. A number of comparative experiments are conducted by utilizing the practical data coming from a steel plant. The results show that the proposed method can deliver effective solutions for long-term scheduling scenarios.
更多查看译文
关键词
Job shop scheduling, Dynamic scheduling, Production, Predictive models, Dynamic programming, Steel, Steel industry, Energy scheduling, byproduct gas system, steel industry, granular computing, reinforcement learning
AI 理解论文
溯源树
样例
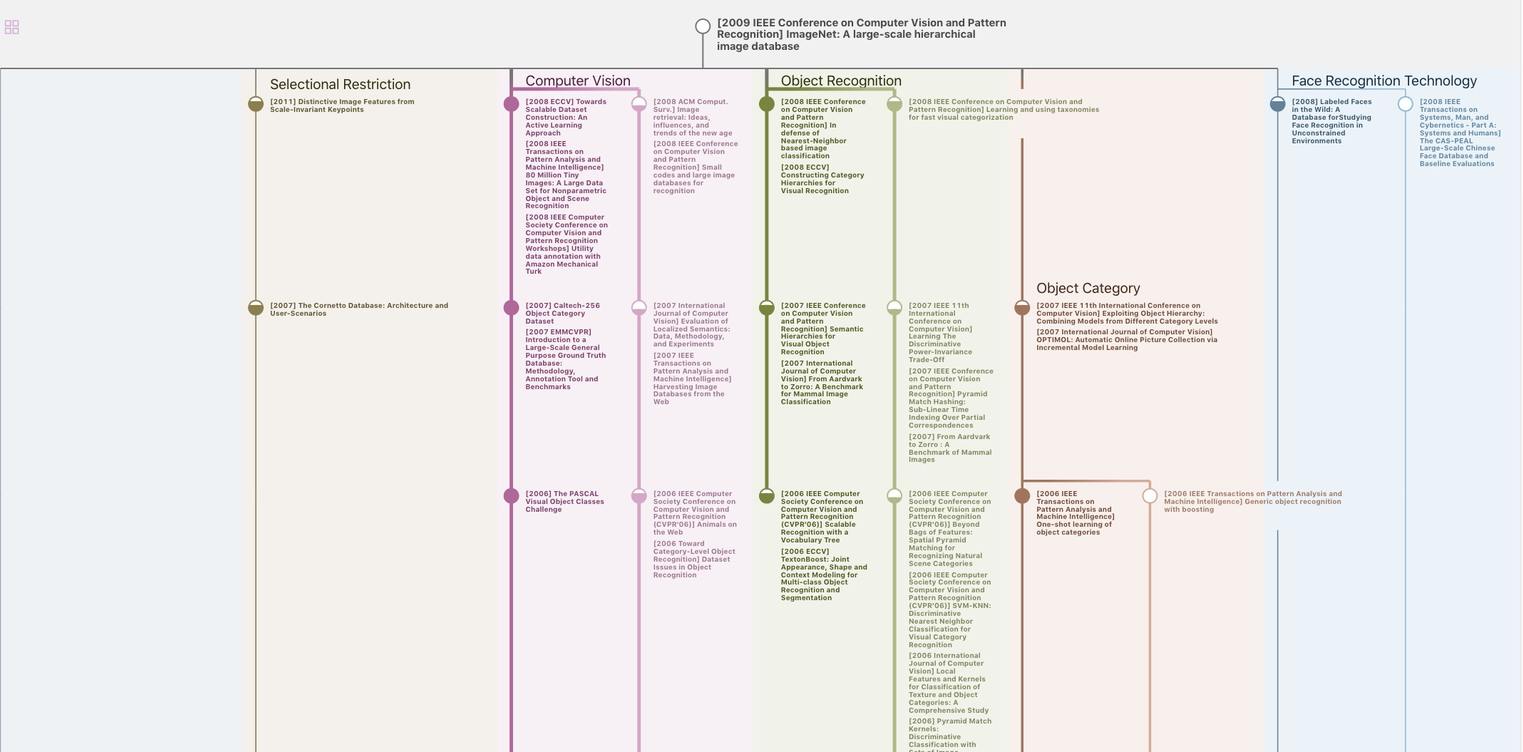
生成溯源树,研究论文发展脉络
Chat Paper
正在生成论文摘要