Automated diagnosis of depression from EEG signals using traditional and deep learning approaches: A comparative analysis
BIOCYBERNETICS AND BIOMEDICAL ENGINEERING(2022)
摘要
Depression is one of the significant contributors to the global burden disease, affecting nearly 264 million people worldwide along with the increasing rate of suicidal deaths. Electroencephalogram (EEG), a non-invasive functional neuroimaging tool has been widely used to study the significant biomarkers for the diagnosis of the disorder. Computational Psychiatry is a novel avenue of research that has shown a tremendous success in the automated diagnosis of depression. The present comprehensive review concentrate on two approaches widely adopted for an EEG based automated diagnosis of depression: Deep Learning (DL) approach and the traditional approach based upon Machine Learning (ML). In this review, we focus on performing the comparative analysis of a variety of signal processing and classification methods adopted in the existing literature for these approaches. We have discussed a variety of EEG based objective biomarkers and the data acquisition systems adopted for the diagnosis of depression. Few EEG studies focusing on multi modal fusion of data have also been explained. Additionally, the research based upon the analysis and prediction of treatment outcome response for depression using EEG signals and machine learning techniques has been briefly discussed to aware the researchers about this emerging field. Finally, the future opportunities and a valuable discussion on major issues related to this field have been summarized that will help the researchers in developing more reliable and computationally intelligent systems in the field of psychiatry.(c) 2021 Nalecz Institute of Biocybernetics and Biomedical Engineering of the Polish Academyof Sciences. Published by Elsevier B.V. All rights reserved.
更多查看译文
关键词
Electroencephalogram, Major depressive disorder, Automated-diagnosis, Treatment-outcome prediction, Machine learning, Deep learning
AI 理解论文
溯源树
样例
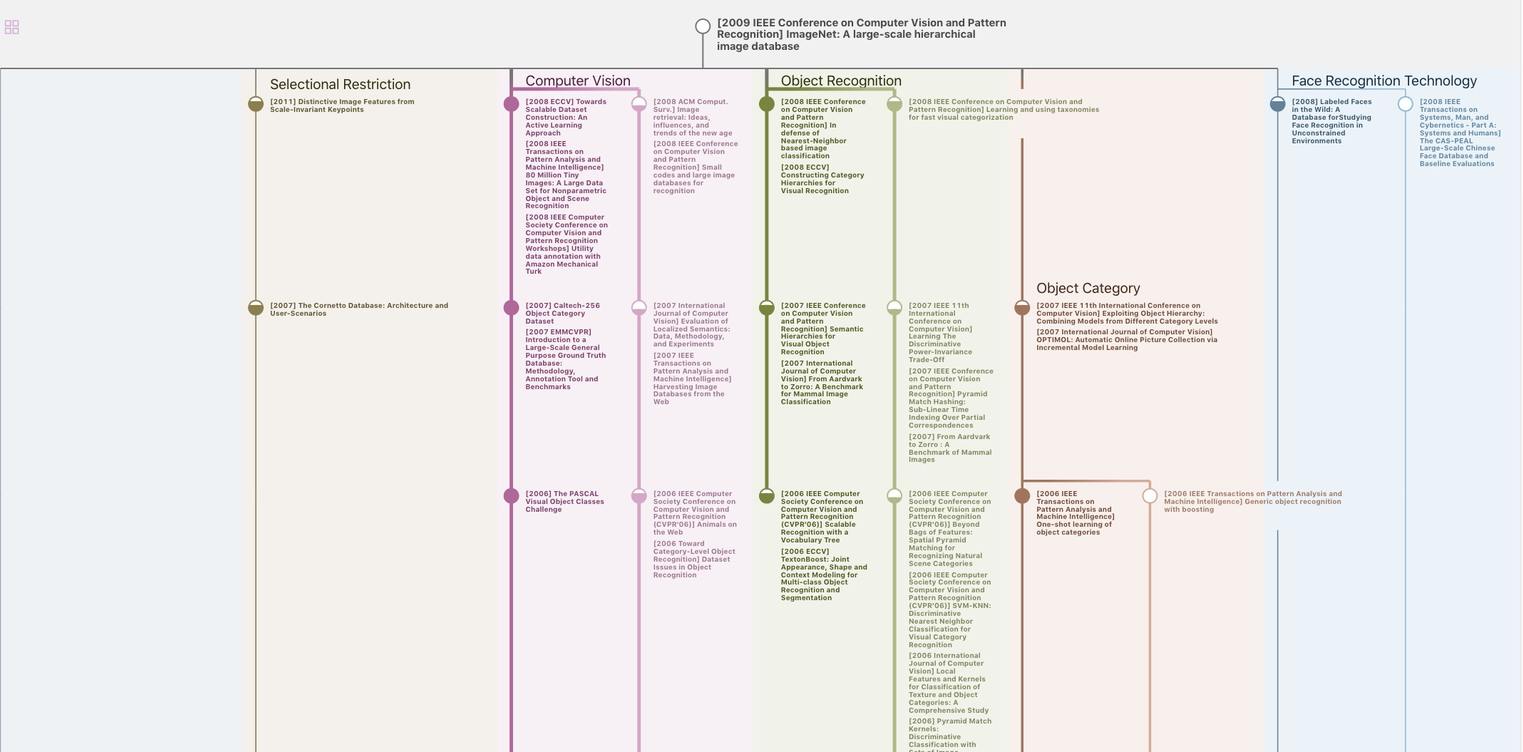
生成溯源树,研究论文发展脉络
Chat Paper
正在生成论文摘要