Training data reduction for deep learning-based image classifications using random sample consensus
JOURNAL OF ELECTRONIC IMAGING(2022)
摘要
Training data for deep learning algorithms can have many redundancies, which should be resolved to achieve faster training speed and efficient storage usage. We proposed a random sample consensus (RANSAC)-based training data selection technique to reduce the training data size for deep learning-based image classification tasks. First, we formulate the data reduction problem as a least square problem and reformulate the equation as maximizing the accuracy of the total training set. Based on the reformulated equation, we applied an RANSAC algorithm to solve the optimization problem. We obtain superior or comparable accuracies to other data selection approaches, such as random, greedy k-means-based, and least square-based approaches. Notably, our algorithm was not degraded in small data selection, unlike other state-of the art algorithms. (C) 2022 SPIE and IS&T
更多查看译文
关键词
deep learning, core-set selection, data reduction, convolutional neural networks, image classification
AI 理解论文
溯源树
样例
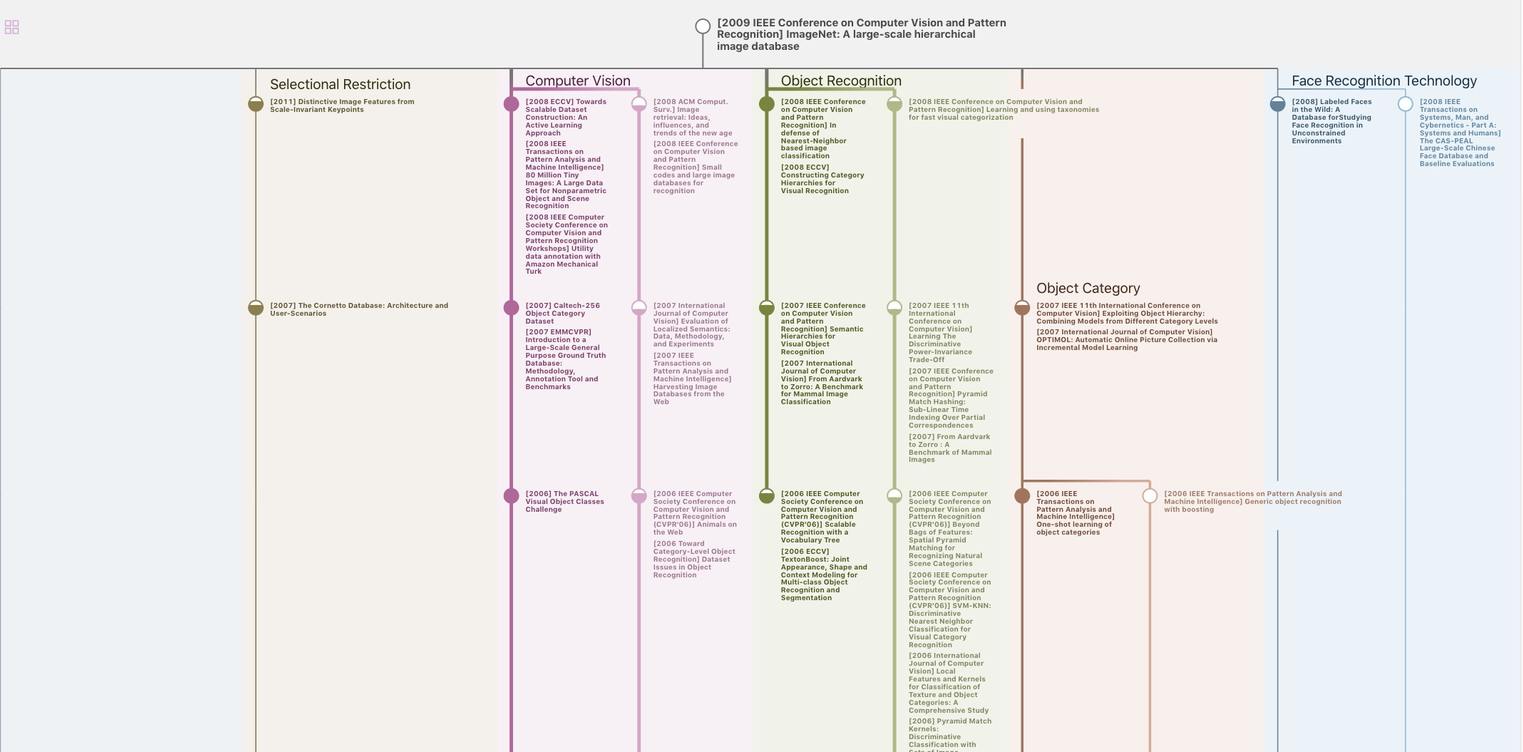
生成溯源树,研究论文发展脉络
Chat Paper
正在生成论文摘要