Fast feature selection via streamwise procedure for massive data
BRAZILIAN JOURNAL OF PROBABILITY AND STATISTICS(2022)
摘要
Variable selection has become an indispensable part of statistical analysis for high-dimensional datasets. However, classical variable selection algorithms, such as regularization methods, are computationally high demanding when sample size and dimension of dataset are both large. Lin, Foster and Ungar (Journal of the American Statistical Association 106 (2011) 232-247) proposed a variable selection algorithm called VIF regression for massive datasets which is more computationally efficient and able to control the marginal false discovery rate. Building on the idea of VIF regression, we propose a new variable selection algorithm, Double-Gates Streamwise regression (DGS), which quickly tests whether predictors significantly reduce the prediction error in one-pass search. DGS regression has two main appealing features. First, DGS regression is computationally efficient and low demanding in the usage of memory. Second, DGS regression can control the false discovery rate, and hence improve the predictive and explanatory abilities. Its advantages relative to VIF regression and some other popular variable selection algorithms are demonstrated in extensive numerical simulated experiments and a real dataset analysis.
更多查看译文
关键词
Massive data, variable selection, streamwise selection
AI 理解论文
溯源树
样例
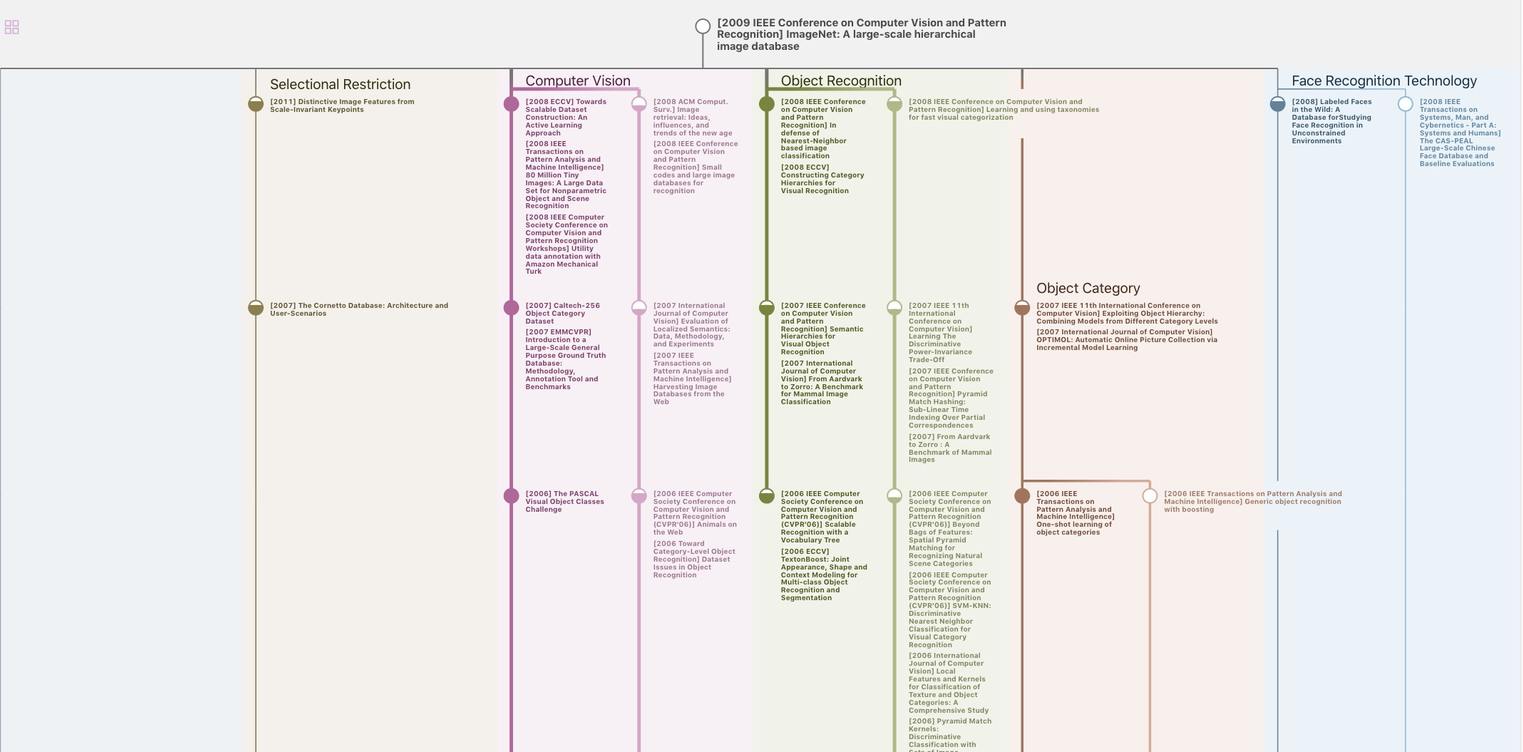
生成溯源树,研究论文发展脉络
Chat Paper
正在生成论文摘要