Early Warning Method for Coal and Gas Outburst Prediction Based on Indexes of Deep Learning Model and Statistical Model
FRONTIERS IN EARTH SCIENCE(2022)
摘要
The early warning models for coal and gas outburst have become increasingly more important and have gained more attention in the mining industry in an effort to further improve mine safety. In the warning process, however, the theoretical models do not always work in a timely manner largely due to the delayed capture of the real time parameters. Based on the evolving mechanism of gas outburst, the gas emission is considered a dominant factor in this work because its data is attainable in real time and clearly characterizes the entire outburst process. In order to characterize and distinguish the variation of the gas emission during an outburst and normal mining activity, a total of four statistical methods were employed to quantify the variation of gas emission: the moving average, the deviation ratio, the dispersion ratio, and the fluctuation ratio. Also, the Root Mean Square Error (RMSE) and Mean Absolute Percentage Error (MAPE) are also included to demonstrate the accuracy of the deep learning model for predicting the variation of gas emission. Developed from these six indicators, the multi-factor fuzzy comprehensive evaluation model forms the outburst early warning system by calculating the combined index of the difference among the indicators. The accuracy of the early warning system is examined in the case study of the "3.25" gas outburst hazard in Shigang Coal Mine. The results show advantages of the comprehensive evaluation model established from the six characteristic indicators when predicting an outburst.
更多查看译文
关键词
gas emission quantity, coal and gas outburst, deep learning, Bi-LSTM, coal
AI 理解论文
溯源树
样例
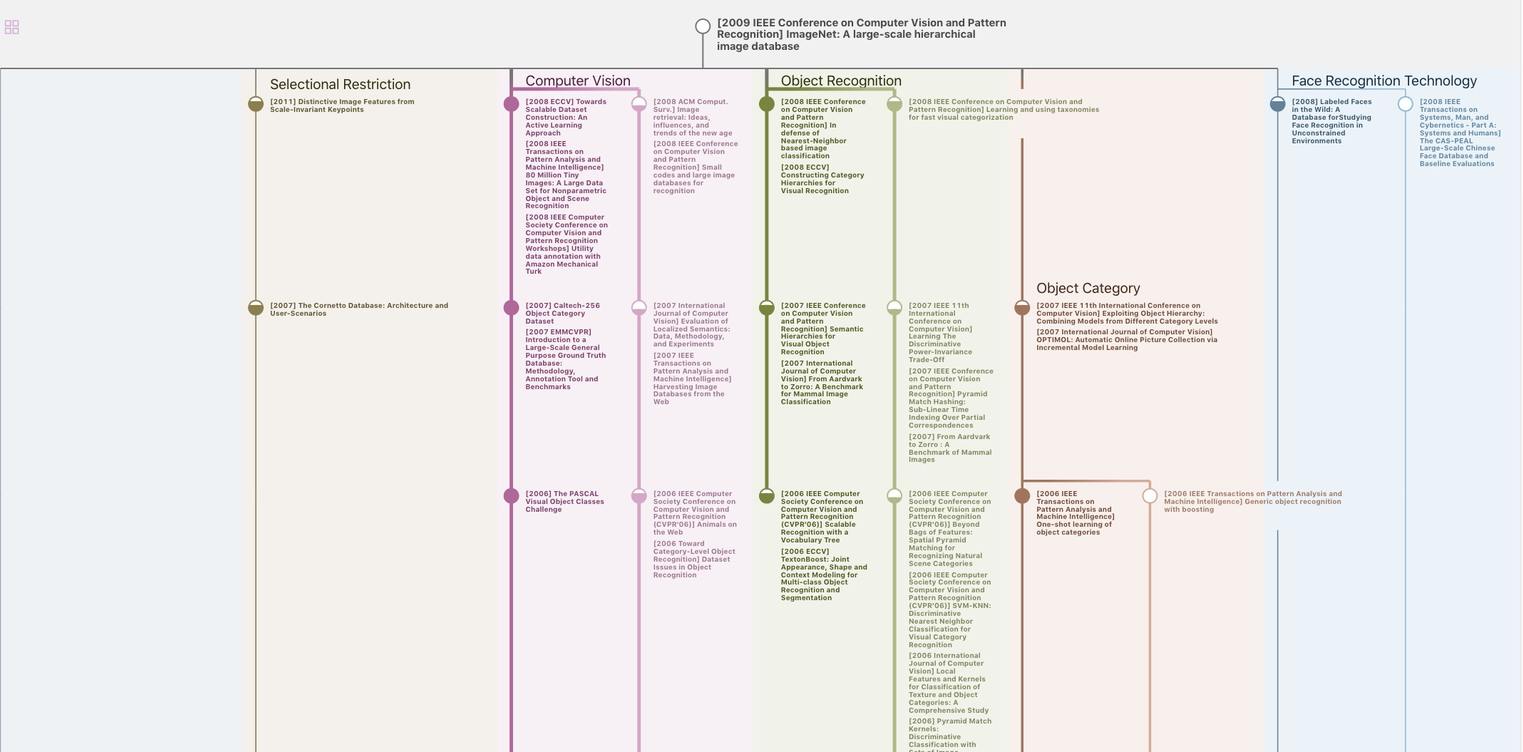
生成溯源树,研究论文发展脉络
Chat Paper
正在生成论文摘要